Discovery and construction of prognostic model for clear cell renal cell carcinoma based on single-cell and bulk transcriptome analysis
Introduction
Kidney cancer is a common malignant tumor with 431,288 new cases diagnosed worldwide, and 179,368 deaths are recorded in 2020 (1). Renal cell carcinoma (RCC) represents about 90% of kidney cancers, clear cell renal cell carcinoma (ccRCC) is the most common histological subtype, accounting for 80–90% of RCC patients (2). Approximately 20–40% of RCC patients may suffer tumor recurrence after surgery (3). As the most lethal form of RCC, ccRCC is associated with a worse prognosis when compared with papillary RCC, chromophobe RCC and other subtypes, the 5-year survival rate of metastatic ccRCC drops to 10–20% (4). Tumor heterogeneity is a hallmark of ccRCC, which has been a major obstacle to personalized medicine and may contribute to tumor recurrence (5). And considering the clinical feature of insensitivity to chemotherapy and radiotherapy, a substantial proportion of patients showing no response to targeted therapy and immunotherapy, investigations on molecular biology and prognostic predictors of ccRCC are still imperatively needed.
Single-cell transcriptome sequencing generally refers to single-cell RNA sequencing (scRNA-seq), which is used to detect gene expression of a single cell. The technique provides comprehensive and high-resolution information of tumors at the single cell level and has been used in cancer research increasingly. Tumor heterogeneity and biomarkers related to prognosis could be studied at a deeper level. Prognostic predictors of ccRCC have been studied, age of patients, tumor histologic grade and metastasis status are reported to be independent risk factors for overall survival (OS) of ccRCC patients (6). ccRCC is a representative of immune-infiltrated tumors. The tumor microenvironment influences tumor biology and affects the response to treatment (7). The screening of valuable immune cell-related genes could provide a new optimized strategy for prognostic prediction of ccRCC. Postoperative prognostic nomograms are superior to conventional prognostic schemes in predictive accuracy (2). And considering the cost of scRNA-seq and next-generation sequencing (NGS) has been popularized in hospitals gradually, we integrated scRNA-seq and NGS data to construct an optimized prognostic nomogram for ccRCC. We present the following article in accordance with the TRIPOD reporting checklist (available at https://dx.doi.org/10.21037/tau-21-581).
Methods
Data acquisition
The scRNA-seq count matrix has been described by Young et al. in their supplementary materials, the sequencing data can also be acquired from the European Genome-phenome Archive (EGA) database (8). The bulk transcriptome data and corresponding clinical information of ccRCC patients were downloaded from The Cancer Genome Atlas (TCGA) database (https://portal.gdc.cancer.gov/). Five hundred and thirty-nine ccRCC samples and 72 matched normal kidney samples were retrospectively studied. The study was conducted in accordance with the Declaration of Helsinki (as revised in 2013). The ethical approval was not required because the data we used were obtained from public databases. Because of the retrospective nature of the research, the requirement for informed consent was waived.
Processing of scRNA-seq data
Transcriptome sequencing data of 14,112 cells from 3 ccRCC patients’ 5 samples was extracted from the scRNA-seq count matrix. The Seurat package in R software was used for quality control (9). Cells with less than 500 detected genes or with more than 10% of mitochondrion-derived genes were filtered out. Genes detected in less than 3 cells were excluded. After the preprocessing, the gene expression of the remaining 5,933 cells was normalized. Gene symbols with a significant difference across cells were identified and a characteristic variance diagram of the top 1,500 variable genes was plotted. After using the ScaleData function for data preparation, principal component analysis (PCA) was performed for linear dimensionality reduction and to identify significantly available dimensions of datasets (10). Then, we used the t-distributed stochastic neighbor embedding (tSNE) algorithm to visualize cluster classification across all cells (11). The marker genes of each cluster were identified according to the cutoff criteria of adjusted P-value <0.05 and |logFC| >0.5 by using the FindAllMarkers function of Seurat. Finally, we annotated cell clusters with singleR package and manually determined them on the basis of marker genes from the CellMarker database (12,13).
Classification of ccRCC cell types and characteristic analysis
According to the recognized tumor markers, we identified two clusters of ccRCC cells. We performed pseudotime trajectory analysis to reveal the changes of cancer cells in the evolutionary process by the monocle algorithm (14). The marker genes of the two clusters listed by the FindAllMarkers were used to perform gene ontology (GO) enrichment analysis using clusterProfiler package (15). Clinicopathological data of ccRCC patients from Beijing Hospital was collected and retrospectively studied, immunohistochemical staining confirmed the two subtypes of cancer cells that we described.
Identification of novel cancer cell biomarkers
Basing on the detected marker genes from the scRNA-seq, we further analyzed the gene expression signature of cancer cells. Two novel biomarkers of ccRCC were discovered to be specially expressed in cancer cells. To further confirm their expression in tumor tissues, the bulk RNA-seq data of 539 tumor tissues and 72 matched normal kidney tissues was downloaded from TCGA database (https://portal.gdc.cancer.gov/). The expression of the two novel markers between the tumor group and matched normal group was compared. Statistical significance was set at P<0.05.
Generation and validation of the prognostic risk score model
The marker genes of T cells calculated from scRNA-seq data were further investigated by combining the clinical information of ccRCC patients in TCGA. 70 marker genes with adjusted P-value <0.05 and logFC >1 in scRNA-seq were selected as T cell-related genes (TCRGs). The bulk transcriptome profiles of the TCRGs from 530 patients with matched survival data were extracted from TCGA database. Univariate Cox regression analysis and Kaplan-Meier (KM) survival analysis were performed to screen TCRGs that the differential expression was significantly correlated with OS. A P-value <0.05 was considered statistically significant. The common prognosis-related genes were then included in the least absolute shrinkage and selection operator (Lasso) regression algorithm and multivariate Cox analysis to identify hub genes using the glmnet and survival packages. Afterwards, we constructed a risk score model based on the hub prognosis-related TCRGs to predict the prognosis of ccRCC patients according to bulk RNA-seq (16). The risk score of each patient was calculated as the formula, risk score = Ʃ (Expi * Coefi), in which “Exp” represented the expression level of the corresponding gene and “Coef” represented the regression coefficient calculated by the multivariate Cox analysis. All the ccRCC patients from TCGA were accordingly stratified into a high-risk group and low-risk group, the correlation between risk score and OS was evaluated using KM survival analysis with the log-rank test. The receiver operating characteristic (ROC) curve was plotted to assess the predictive accuracy of the TCRGs based prognostic model by the area under the curve (AUC).
Construction of prognostic nomogram
The TCRG signature and clinical information of ccRCC patients from TCGA were merged. Thirty-two patients with incomplete clinical information were excluded and 498 patients were included in further analysis in this process. Univariate and multivariate Cox regression analyses were performed to evaluate the prognostic significance of clinical features, meanwhile, the hazard ratios (HR) and 95% confidence intervals (CI) were calculated. After considering the weight of clinical parameters in prognosis prediction, the selected independent prognostic parameters were used to construct a prognostic nomogram based on the results of multivariate Cox regression analysis by the rms package. The nomogram model was applied to the prediction of 3-year and 5-year survival outcomes for ccRCC patients, and the predictive accuracy of the nomogram was evaluated by ROC curve analysis.
Statistical analysis
All statistical analyses and graphical representations were calculated by using R software version 3.6.1 and corresponding packages. P<0.05 was considered to be statistically significant.
Results
Data processing and identification of 15 cell clusters in human ccRCC using scRNA-seq data
The schematic diagram of this study is shown in Figure 1. A total of 14,112 cells from 5 samples of 3 ccRCC patients who underwent radical nephrectomy were acquired in this study. The range of detected gene numbers, the sequencing counts and the percentage of mitochondrial sequencing count of each cell were illustrated (Figure 2A). After removing cells of low quality according to quality control standards, 5,933 cells finally passed the quality filtering and were included in the further analysis. The correlation of sequencing depth with the percentage of mitochondrial sequencing count and the detected gene numbers were calculated. The detected gene numbers were significantly positively correlated with the sequencing depth with a Pearson's correlation coefficient of 0.93 (Figure 2B). One thousand five hundred highly variable genes and the top 10 gene names across the cell samples were illustrated following data normalization (Figure 2C). We used the linear dimensionality reduction method PCA to identify available dimensions and screen correlated genes (Figure 2D). The top significantly correlated genes of the first 4 principal components (PCs) were displayed (Figure S1). Twenty PCs with an estimated P-value <0.05 were selected for further analysis (Figure 2E). Afterwards, all the cells were classified into 15 separate clusters and visualized by using the t-SNE algorithm (Figure 2F). A total of 5,750 marker genes (|logFC| >0.5 and adjusted P-value <0.05) of the 15 cell clusters were identified by differential expression analysis (Figure 2G). Cell types of the 15 cell clusters were annotated by singleR and the CellMarker database (Figure S2).
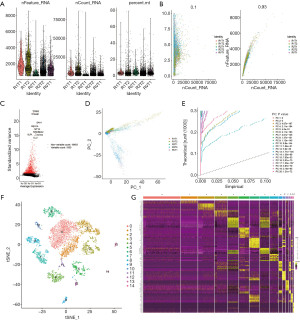
Identification of two ccRCC cell types with distinct differentiation status and biological processes
We identified cancer cells of ccRCC by using carbonic anhydrase IX (CA9) and ANGPTL4, cancer cells were divided into two subgroups, cluster 5 and cluster 9 (Figure 3A). Cells of cluster 5 were annotated as adipocytic type cancer cells and cluster 9 were annotated as epithelial type cancer cells by SingleR. Pseudotime trajectory analysis was performed and we observed a significant differentiation tendency from cluster 5 of the adipocytic type cancer cells to cluster 9 of the epithelial type cancer cells in the first branch, indicating the underlying degree of differentiation and transcriptional heterogeneity between the two cancer cell subtypes in ccRCC (Figure 3B). The marker genes of cluster 5 (Figure 3C) and cluster 9 (Figure 3D) were applied to GO analysis, respectively. Both of them were mainly enriched in the biological processes of T cell activation, neutrophil activation and response to hypoxia, etc. The adipocytic type cancer cells showed the tendency to activate adaptive immune response more than the epithelial type cancer cells, while the epithelial type cancer cells significantly correlated with innate immune response. The epithelial type cancer cells specially expressed the epithelial marker PAX8 (Figure 3E). The samples R1T1 and R1T2 came from the same patient (RCC1), so did R2T1 and R2T2 (RCC2). Cancer cells of RVT1 and R2T2 were adipocytic type and R1T1 were epithelial type. The R1T2 and R2T2 were mixtures of both types of cancer cells, indicating intertumor and intratumor heterogeneity of ccRCC (Figure 3F). We also retrospectively analyzed pathological reports of ccRCC patients during January 2014 to January 2020 in Beijing Hospital. Since PAX8 immunohistochemical staining was not generally performed, only 41 cases of ccRCC were involved. The real-world data showed that 31 primary and 4 metastatic ccRCC were PAX8 positive, while 4 primary and 2 metastatic ccRCC were PAX8 negative, proving our findings of the existence of the two types of cancer cells (Table S1).
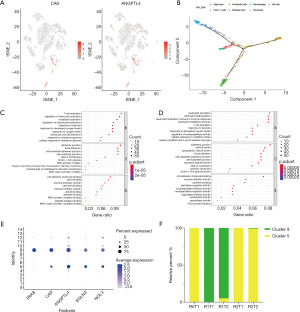
EGLN3 and NOL3 could be novel biomarkers of ccRCC
CA9 and ANGPTL4 have been reported to be biomarkers of ccRCC (17). However, there are not many generally acknowledged biomarkers for ccRCC. We found that egl-9 family hypoxia-inducible factor 3 (EGLN3) and nucleolar protein 3 (NOL3) were specially expressed in the two cancer cell clusters (Figures 3E,4A), they may be novel biomarkers of ccRCC. To confirm their expression levels in tumor tissues, we extracted bulk transcriptome data of 539 ccRCC samples and 72 matched normal kidney samples. EGLN3 and NOL3 were detected highly expressed in tumor tissues than matched normal tissues significantly (Figure 4B). Seventy-two pairs of tumor and normal tissues were matched in the data, the two genes showed significant expression difference between the two groups via Wilcoxon matched pairs test (Figure 4C)

Screening of TCRGs for risk score prognostic model
T cells are the most abundant population in the tumor microenvironment of ccRCC, which are attractive prognostic biomarkers and show promise as therapeutic targets (18). According to our cell classification of scRNA-seq data, cluster0, cluster1, clusster3 and cluster 11 were annotated as T cell clusters (Figure S2). Seventy marker genes with logFC >1 were selected as the hub TCRGs from the four T cell clusters. We extracted the gene expression profiles of the 70 hub TCRGs from TCGA database and merged them with 530 corresponding survival information of ccRCC patients (Table S2). Univariate Cox analysis and KM survival analysis were performed, 39 and 33 prognostic TCRGs were screened out, respectively (P<0.05) (Figure 5A,5B). Twenty-seven common prognostic genes were further assessed by Lasso regression analysis and the process was repeated 1,000 times, 8 genes were identified (Figure 5C,5D). Afterwards, multivariate Cox analysis was performed and 4 key prognosis-related genes were identified (Figure 5E).
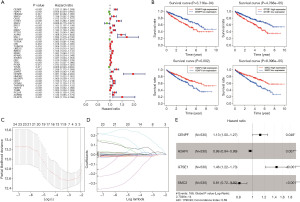
A prognostic risk score model was constructed based on the 4 survival-related TCRGs in TCGA dataset. The risk score of each patient was calculated as: risk score = ExpCENPF × 0.12 + ExpH2AFV × (−0.037) + ExpGTSE1 × 0.376 + ExpSMC2 × (−0.207). Then we used the median value of the risk scores as the cutoff value to divide the 530 patients into a low-risk (low score) group and a high-risk (high score) group. KM survival analysis showed that patients in the high-risk group had a worse prognosis than those in the low-risk group in OS (P<0.001) (Figure 6A). The ROC curve showed favorable AUC values indicating that the risk score model had excellent performance in predicting OS of ccRCC patients (Figure 6B). The curve of risk score distribution and the scatterplot of survival status also showed the significant difference between the two groups (Figure 6C,6D).
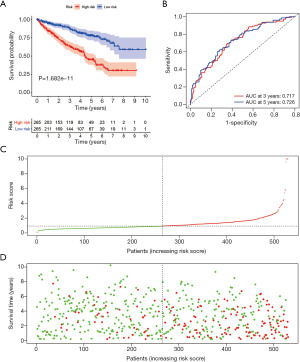
Construction of prognostic nomogram with the risk score and clinical parameters
To make this risk score model clinically applicable, we generated a nomogram according to the expression of the four genes to predict 3-year and 5-year OS for ccRCC patients (Figure S3). Since some clinical characteristics are also viewed as prognostic predictors, we performed univariate and multivariate Cox analysis to investigate whether the risk score was independent of the other clinical characteristics in predicting the OS of ccRCC patients (Figure 7A,B). The forest plots showed that the TCRG risk score was an independent prognostic predictor. Age, histologic grade and tumor, node, metastasis (TNM) classification were significantly correlated with OS, gender seemed to be irrelevant in survival prediction. After weighing the importance of the above parameters, we selected risk score, age, histologic grade and metastasis classification (P<0.01) to construct a concise and clinically applicable nomogram model to help clinicians and patients in prognosis prediction (Figure 7C). Five patients with risk score more than 6 were all died, the majority of the patients have the risk score within 6, so we removed the five outliners to make the model more accurate. The total points summarized the points of selected parameters that could predict the probability of OS at 3-year and 5-year. ROC curves were plotted with the AUC of 3-year and 5-year reached 0.710 and 0.717, indicating the excellent predictive ability of the nomogram model (Figure 7D,7E).
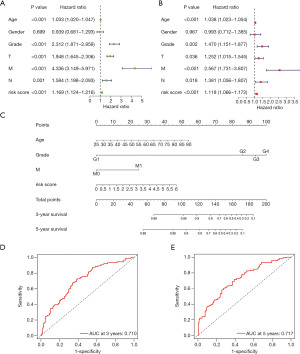
Discussion
The incidence of RCC is increasing in most countries. Though the disease outcome has shown some improvement in recent years, the overall prognosis is relatively poor. It remains the most lethal of the common urologic cancers (19). The most frequent RCC subtype is ccRCC, which is often viewed to arise from proximal tubular epithelial cells (20). The primary therapy for localized ccRCC is surgical resection, but about 30% of patients may experience recurrence (21). To make matters worse, the cancer is resistant to conventional radiotherapy and chemotherapy, tumor heterogeneity is also an obstacle to effective therapies (22). Biomarkers for potential prognostication of ccRCCs have been frequently proposed, but no recognized predictor has been used in clinical practice. Further understandings of tumor biology and biomarkers for prognosis in ccRCC are urgently needed.
Single-cell transcriptome sequencing enables the detection of gene expression of each cell. The utility of scRNA-seq helps us to better understand the underlying biological mechanism and may bring promising prospect for clinical diagnosis and therapy. ccRCC is distinct for abundant immune-infiltration and angiogenesis (23). Targeted therapy and novel immunotherapy have changed the treatment landscape of advanced ccRCC, however, a substantial proportion of patients show no response to these therapies (24). The reason for innate or acquired drug resistance is the cancer cell itself or influenced by the tumor microenvironment, or else both is still unclear. In the present study, we analyzed scRNA-seq data of 5 samples from 3 patients to depict gene expression features of ccRCC. After quality control to filter out low-quality cells, we identified 15 cell clusters including stromal cells and cancer cells within ccRCC tissues. We recognized two subtypes of cancer cells, cluster 5 was annotated as adipocytic type cancer cells and cluster 9 was annotated as epithelial type cancer cells. ccRCC is derived from proximal tubular epithelial cells, abnormal glycogen deposit and lipid accumulation are typical characteristics of ccRCC (20). The two phenotypes of cancer cells showed some corresponding similarities with the two types of tissues. Pseudotime trajectory analysis showed the distinct differentiation status of the two cancer cell types. The epithelial type cancer cells showed higher differentiation status than the adipocytic type cancer cells. We performed GO analysis to identify related biological processes of cancer cells with distinct phenotypes. The results revealed that maker genes of the two cancer cells were both enriched in response to hypoxia, T cell activation and neutrophil activation. The adipocytic type cancer cells showed more tendency to be correlated with T cell activation, regulation of lymphocyte activation and cellular response to interferon-gamma, while the epithelial type cancer cells were more related to neutrophil activation, regulation endopeptidase activity and cellular modified amino acid metabolic process. It suggested that the two types of cancer cells may give preferential response to innate or adaptive immune, which may influence the outcome of immunotherapy. We also found that the epithelial marker PAX8 was specially expressed in the epithelial type cancer cells, it could be a potential predictor for immunotherapy that needs to be verified in the future. The composition of tumors was different, the RVT1, R1T1 and R2T1 consisted of either the epithelial type cancer cells or adipocytic type cancer cells, meanwhile, R1T2 and R2T2 were both mixtures of the two types of cancer cells and the relative cell percent was different, which proved intertumor and intratumor heterogeneity. These findings indicate that ccRCC cells in distinct differentiation states may attribute to different tumor biology characteristics, including both intrinsic properties and the regulation of biological processes, which might provide new evidence for the clinical phenotypes of ccRCC. Although we are insufficient in the investigations on significance of each cell type in clinical practice. It is expected that the two types of cancer cell targeting immunotherapy might become a rational modality in therapy for ccRCC.
A variety of biomarkers hitherto have been proposed as pathological indexes for diagnosing ccRCC, including CD10, vimentin and renal cell carcinoma antigen (20). But most of them are normally expressed in proximal tubular cells, supporting the origin of ccRCC. There are not many biomarkers specially expressed in cancer cells while absent in normal tissues such as CA9, though ANGPTL4, FABP6 and NDUFA4L2 were reported to be biomarkers of ccRCC (17). We found that EGLN3 and NOL3 were co-expressed with the conventional marker CA9 in ccRCC cell clusters, the special expression pattern indicated that they could be candidate biomarkers for ccRCC. To confirm their expression in ccRCC tissue, we downloaded the gene expression data of EGLN3 and NOL3 in ccRCC from TCGA database. The data sets showed that both of the two genes were expressed markedly higher in tumor tissues than normal tissues. We also compared the 72 pairs of ccRCC tissues and matched normal kidney tissues, the result was consistent with the previous comparison. EGLN3 is also known as prolyl hydroxylase 3 (PHD3), it exists in the nucleus and cytoplasm. Under normoxic conditions, EGLN3 hydroxylates proline residues of hypoxia-inducible factor α (HIF-α) and the critical factor in the process of recognizing the ubiquitin ligase complex von Hippel−Lindau (VHL) will bind to the HIF-α to initiate the proteasomal degradation of HIF-α (25). Moreover, EGLN3 may play important roles in fatty acid oxidation and glucose metabolism in cancers (26,27). It has been reported that EGLN3 may serve as one of potent immunogenic antigens of RCC and become a rational modality in therapy (28). We confirmed that the EGLN3 was specially expressed in ccRCC cancer cells but not in stromal cells at a single-cell level. A high level of serum EGLN3 is also expected to be a diagnostic parameter for RCC (29). NOL3 is also known as an apoptosis repressor with a caspase recruitment domain (ARC), it potentially antagonizes apoptosis pathways to inhibit cell death (30). Increased expression of NOL3 is shown in solid tumors and mediate cellular responsiveness to pharmacologic apoptosis induction (31). But a study held the view that NOL3 was decreased in the majority of ccRCC and decreased NOL3 conferred resistance to sunitinib in vitro, which are contradictory to available researches (32). The roles of NOL3 in ccRCC remain controversial and need further study.
There have been various models designed for prognostic prediction following surgery. Unfortunately, no generally acknowledged risk model has been applied in routine clinical practice for ccRCC until now. Many models are developed based on clinical features, including age, TNM classification, histologic grade, etc. But there is little room for improvement to optimize conventional models by selecting clinical parameters. Identification and inclusion of molecular biomarkers have led to new insights into survival outcome prediction (33). We used the scRNA-seq data of ccRCC with a process of quality control, 15 cell clusters were identified and Cluster 0, Cluster 1, Cluster3 and Cluster 11 were annotated as T cell clusters. We selected 70 hub TCRGs for further study from scRNA-seq data according to logFC >1 and P<0.05. Afterwards, we extracted the transcriptome sequencing data of ccRCC patients from TCGA database and integrated them with corresponding clinical information. 27 common prognostic genes were screened by KM survival analysis and univariate Cox analysis on OS of 530 patients from TCGA. Then we performed Lasso regression analysis and identified 4 prognostic TCRGs for risk model establishment based on multivariate Cox analysis. The ROC curves indicated the good performance of the TCRG risk model in survival prediction. To improve the risk model, we integrated the risk score with other important clinical characteristics to construct a comprehensive nomogram. The risk score and other clinical characteristics were analyzed by univariate and multivariate Cox analysis. The results showed that age, histologic grade, TNM classification and risk score were significantly correlated with OS. We excluded T and N classification for they showed less influence in the model and finally selected four independent risk features into our model which consisting of age, histologic grade, M classification and TCRG risk score. The ROC curve for the observed 3-year and 5-year outcomes showed that the nomogram model performed well in prognosis prediction.
Of note, one advantage of our study was the combination of scRNA-seq and bulk RNA-seq data. Single-cell transcriptomics analysis provides accurate cell states and gene expression characteristics, but the expensive cost limits its routine practice. Bulk RNA-seq has been popularized in clinical and basic researches gradually. The cost is comparatively cheap which is suitable for routine detection and huge amounts of bulk RNA-seq data is stored in public databases. T cells are a type of foremost component of the tumor microenvironment. CD4+ helper T cells and cytotoxic CD8+ T cells respond to tumor immunogen and kill tumor cells (18). Treatments focused on T cells show promise and have been applied in immune checkpoint therapy. Therefore, we sought to construct a predictive model for the oncological outcomes of ccRCC by combining TCRGs and bulk transcriptional data. Nevertheless, there are still several points of our study need to be optimized. First, we make this analysis using data from published databases, the predictive model needs to be validated in a large cohort of local patients. Additionally, this work is based on bioinformatics analysis and basic experiments are still needed to uncover the underlying mechanism.
Conclusions
In this study, we discovered and demonstrated that ccRCC cells could be divided into two subtypes with distinct differentiation characteristics and biological features. This discovery may give some enlightenment for novel immunotherapy. In addition, EGLN3 and NOL3 could be efficient biomarkers for ccRCC, they may have important pathological diagnosis and clinical implication value. Notably, we firstly screened marker genes of T cells from scRNA-seq data of ccRCC and constructed a novel nomogram for survival prediction by combining TCGA database. Taken together, our findings are helpful to further understand ccRCC and establish a maneuverable and convenient model which could be used in routine clinical practice.
Acknowledgments
We are grateful to The Cancer Genome Atlas (TCGA), the Clinical Biobank of Beijing Hospital, Dr. Young and Dr. Behjati’ group for providing public data in this study.
Funding: None.
Footnote
Reporting Checklist: The authors have completed the TRIPOD reporting checklist. Available at https://dx.doi.org/10.21037/tau-21-581
Data Sharing Statement: Available at https://dx.doi.org/10.21037/tau-21-581
Conflicts of Interest: All authors have completed the ICMJE uniform disclosure form (available at https://dx.doi.org/10.21037/tau-21-581). The authors have no conflicts of interest to declare.
Ethical Statement: The authors are accountable for all aspects of the work in ensuring that questions related to the accuracy or integrity of any part of the work are appropriately investigated and resolved. The study was conducted in accordance with the Declaration of Helsinki (as revised in 2013). The ethical approval was not required because the data we used were obtained from public databases. Because of the retrospective nature of the research, the requirement for informed consent was waived.
Open Access Statement: This is an Open Access article distributed in accordance with the Creative Commons Attribution-NonCommercial-NoDerivs 4.0 International License (CC BY-NC-ND 4.0), which permits the non-commercial replication and distribution of the article with the strict proviso that no changes or edits are made and the original work is properly cited (including links to both the formal publication through the relevant DOI and the license). See: https://creativecommons.org/licenses/by-nc-nd/4.0/.
References
- Sung H, Ferlay J, Siegel RL, et al. Global Cancer Statistics 2020: GLOBOCAN Estimates of Incidence and Mortality Worldwide for 36 Cancers in 185 Countries. CA Cancer J Clin 2021;71:209-49. [Crossref] [PubMed]
- Ljungberg B, Albiges L, Abu-Ghanem Y, et al. European Association of Urology Guidelines on Renal Cell Carcinoma: The 2019 Update. Eur Urol 2019;75:799-810. [Crossref] [PubMed]
- Brookman-May SD, May M, Shariat SF, et al. Time to recurrence is a significant predictor of cancer-specific survival after recurrence in patients with recurrent renal cell carcinoma--results from a comprehensive multi-centre database (CORONA/SATURN-Project). BJU Int 2013;112:909-16. [Crossref] [PubMed]
- Fendler A, Bauer D, Busch J, et al. Inhibiting WNT and NOTCH in renal cancer stem cells and the implications for human patients. Nat Commun 2020;11:929. [Crossref] [PubMed]
- Gerlinger M, Rowan AJ, Horswell S, et al. Intratumor heterogeneity and branched evolution revealed by multiregion sequencing. N Engl J Med 2012;366:883-92. [Crossref] [PubMed]
- Gu L, Li H, Wang Z, et al. A systematic review and meta-analysis of clinicopathologic factors linked to oncologic outcomes for renal cell carcinoma with tumor thrombus treated by radical nephrectomy with thrombectomy. Cancer Treat Rev 2018;69:112-20. [Crossref] [PubMed]
- Vuong L, Kotecha RR, Voss MH, et al. Tumor Microenvironment Dynamics in Clear-Cell Renal Cell Carcinoma. Cancer Discov 2019;9:1349-57. [Crossref] [PubMed]
- Young MD, Mitchell TJ, Vieira Braga FA, et al. Single-cell transcriptomes from human kidneys reveal the cellular identity of renal tumors. Science 2018;361:594-9. [Crossref] [PubMed]
- Butler A, Hoffman P, Smibert P, et al. Integrating single-cell transcriptomic data across different conditions, technologies, and species. Nat Biotechnol 2018;36:411-20. [Crossref] [PubMed]
- Lall S, Sinha D, Bandyopadhyay S, et al. Structure-Aware Principal Component Analysis for Single-Cell RNA-seq Data. J Comput Biol 2018; Epub ahead of print. [Crossref] [PubMed]
- Pont F, Tosolini M, Fournié JJ. Single-Cell Signature Explorer for comprehensive visualization of single cell signatures across scRNA-seq datasets. Nucleic Acids Res 2019;47:e133 [Crossref] [PubMed]
- Aran D, Looney AP, Liu L, et al. Reference-based analysis of lung single-cell sequencing reveals a transitional profibrotic macrophage. Nat Immunol 2019;20:163-72. [Crossref] [PubMed]
- Zhang X, Lan Y, Xu J, et al. CellMarker: a manually curated resource of cell markers in human and mouse. Nucleic Acids Res 2019;47:D721-8. [Crossref] [PubMed]
- Qiu X, Mao Q, Tang Y, et al. Reversed graph embedding resolves complex single-cell trajectories. Nat Methods 2017;14:979-82. [Crossref] [PubMed]
- Yu G, Wang LG, Han Y, et al. clusterProfiler: an R package for comparing biological themes among gene clusters. OMICS 2012;16:284-7. [Crossref] [PubMed]
- Nagashima K, Sato Y. Information criteria for Firth's penalized partial likelihood approach in Cox regression models. Stat Med 2017;36:3422-36. [Crossref] [PubMed]
- Hu J, Chen Z, Bao L, et al. Single-Cell Transcriptome Analysis Reveals Intratumoral Heterogeneity in ccRCC, which Results in Different Clinical Outcomes. Mol Ther 2020;28:1658-72. [Crossref] [PubMed]
- Chevrier S, Levine JH, Zanotelli VRT, et al. An Immune Atlas of Clear Cell Renal Cell Carcinoma. Cell 2017;169:736-749.e18. [Crossref] [PubMed]
- Znaor A, Lortet-Tieulent J, Laversanne M, et al. International variations and trends in renal cell carcinoma incidence and mortality. Eur Urol 2015;67:519-30. [Crossref] [PubMed]
- Frew IJ, Moch H. A clearer view of the molecular complexity of clear cell renal cell carcinoma. Annu Rev Pathol 2015;10:263-89. [Crossref] [PubMed]
- Leibovich BC, Lohse CM, Cheville JC, et al. Predicting Oncologic Outcomes in Renal Cell Carcinoma After Surgery. Eur Urol 2018;73:772-80. [Crossref] [PubMed]
- Clark DJ, Dhanasekaran SM, Petralia F, et al. Integrated Proteogenomic Characterization of Clear Cell Renal Cell Carcinoma. Cell 2019;179:964-983.e31. [Crossref] [PubMed]
- Liu XD, Hoang A, Zhou L, et al. Resistance to Antiangiogenic Therapy Is Associated with an Immunosuppressive Tumor Microenvironment in Metastatic Renal Cell Carcinoma. Cancer Immunol Res 2015;3:1017-29. [Crossref] [PubMed]
- Tegos T, Tegos K, Dimitriadou A, et al. Current and emerging first-line systemic therapies in metastatic clear-cell renal cell carcinoma. J BUON 2019;24:1340-53. [PubMed]
- Li Z, You Q, Zhang X. Small-Molecule Modulators of the Hypoxia-Inducible Factor Pathway: Development and Therapeutic Applications. J Med Chem 2019;62:5725-49. [Crossref] [PubMed]
- German NJ, Yoon H, Yusuf RZ, et al. PHD3 Loss in Cancer Enables Metabolic Reliance on Fatty Acid Oxidation via Deactivation of ACC2. Mol Cell 2016;63:1006-20. [Crossref] [PubMed]
- Miikkulainen P, Högel H, Rantanen K, et al. HIF prolyl hydroxylase PHD3 regulates translational machinery and glucose metabolism in clear cell renal cell carcinoma. Cancer Metab 2017;5:5. [Crossref] [PubMed]
- Sato E, Torigoe T, Hirohashi Y, et al. Identification of an immunogenic CTL epitope of HIFPH3 for immunotherapy of renal cell carcinoma. Clin Cancer Res 2008;14:6916-23. [Crossref] [PubMed]
- Kim KH, Lee HH, Yoon YE, et al. Prolyl hydroxylase-3 is a novel renal cell carcinoma biomarker. Investig Clin Urol 2019;60:425-31. [Crossref] [PubMed]
- Kronenberg G, Gertz K, Uhlemann R, et al. Reduced Hippocampal Neurogenesis in Mice Deficient in Apoptosis Repressor with Caspase Recruitment Domain (ARC). Neuroscience 2019;416:20-9. [Crossref] [PubMed]
- Stanley RF, Piszczatowski RT, Bartholdy B, et al. A myeloid tumor suppressor role for NOL3. J Exp Med 2017;214:753-71. [Crossref] [PubMed]
- Gobe GC, Ng KL, Small DM, et al. Decreased apoptosis repressor with caspase recruitment domain confers resistance to sunitinib in renal cell carcinoma through alternate angiogenesis pathways. Biochem Biophys Res Commun 2016;473:47-53. [Crossref] [PubMed]
- Klatte T, Rossi SH, Stewart GD. Prognostic factors and prognostic models for renal cell carcinoma: a literature review. World J Urol 2018;36:1943-52. [Crossref] [PubMed]