Overexpressing IFITM family genes predict poor prognosis in kidney renal clear cell carcinoma
Introduction
Renal cell cancer (RCC) is the most prevalent form of kidney cancer and is notorious for its metastatic potential (1). According to the pathological classification by the International Society of Urological Pathology Vancouver Consensus Statement, RCC is categorized into clear cell, and types I and II papillary and chromophobe subtypes (2). Clear cell RCC or kidney renal clear cell carcinoma (KIRC), accounting for 75% of RCC cases, is one of the most aggressive (3).
KIRC stands out as one of the most immune infiltrated tumors in pan-cancer comparisons (4). Recently immune checkpoint inhibitors (ICIs) have proven highly efficacious in this disease (5). Tumor microenvironment (TME) heavily impacts KIRC cell biology and affects tumor prognosis and treatment response (4). Tumor-infiltrating cells of TME could demonstrate either tumor-suppressive or tumor-promoting effects, depending on distinct tumor models (6). Unlike most other cancer types, overall high CD8 T cell infiltration in KIRC was associated with worse prognosis (7). So, it is important to clarify the heterogeneous tumor microenvironment of KIRC.
Interferon-induced transmembrane proteins (IFITMs) are reported to be interacted with immune cell infiltration (8). IFITMs are a family of effector proteins that impede pathogenic virus entry into host cells. IFITM-1, IFITM-2 and IFITM-3 are the most common isoforms. IFITM-1 is mainly located on the cytoplasmic membrane for entry prevention of the virus, while IFITM-2 and IFITM-3 localize to the endosomal compartment to block fusion pore formation. IFITMs markedly enhance the antiviral activity of monoclonal antibodies against hepatitis C virus and thus might prevent the development of hepatocellular carcinoma (9). IFITM-3 was discovered to be a prognostic indicator for acute myeloid leukemia (10). Also, IFITM2 has been demonstrated to promote gastric cancer progression by promoting cell migration, invasion, and inducing EMT in GC cells (11). However, the role of IFITMs in KIRC and the interactions of IFITMs with TME have been rarely reported and await further clarification. In the present study, we investigated the role of IFITMs in KIRC. Various bioinformatics tools were utilized to investigate their potential biological functions, prognostic values, and regulatory network, as well as the effect on immune cell infiltration. The results of this study provide preliminary evidence of the oncogenic effect, and interacting relationships of IFITMs. We present the following article in accordance with the REMARK reporting checklist (available at https://dx.doi.org/10.21037/tau-21-848).
Methods
Cell cultures
The KIRC cell line (Caki-1) and normal kidney cell line (HK-2) were kindly donated by Dr. Zhiliang Chen. Caki-1 cells were cultured in McCoy’s 5a Modified Medium (Boster, China) and HK-2 was maintained in Dulbecco’s Modified Eagle Medium/Nutrient Mixture F-12 (Gibco). Both media contained 1% penicillin and streptomycin (HyClone), as well as 10% fetal bovine serum (FBS, Gemini). All cells were cultured in a humidified 5% CO2 environment at 37 °C.
Quantitative reverse transcription polymerase chain reaction (qRT-PCR)
Total RNA was extracted from cells using the EZ-press RNA Purification Kit (EZBioscience, Guangzhou, China). The RNA purity was evaluated by A260:A280 ratio using a NanoDrop spectrophotometer (ND-2000c). cDNA was synthesized using the PrimeScript RT Reagent Kit (Takara, Japan). qRT-PCR was completed on a LightCycler 480 (Roche), using TB Green Premix Ex Taq (Takara Bio). Denaturation was set at 95 °C for 30 s with 40 total cycles and a second step at 95 °C for 5 s. The expression levels of genes were normalized to that of GAPDH and determined by the Ct –△△2t method. Gene-specific primers were listed in the Table 1.
Table 1
Gene | Forward sequence (5'- 3') | Reverse sequence (5'- 3') |
---|---|---|
IFITM-1 | GGCTTCATAGCATTCGCCTACTC | AGATGTTCAGGCACTTGGCGGT |
IFITM-2 | CATCCCGGTAACCCGATCAC | CACGGAGTACGCGAATGCTA |
IFITM-3 | CATCCCAGTAACCCGACCG | TGTTGAACAGGGACCAGACG |
GAPDH | GGAGCGAGATCCCTCCAAAAT | GGCTGTTGTCATACTTCTCATGG |
Western blot
Cells were lysed with buffer (CWbio, China) on ice, and proteins (20 mg) were separated on 10% sodium dodecyl sulfate/polyacrylamide gel electrophoresis gel (Beyotime, China), before being transferred to polyvinylidene difluoride membranes (Millipore, Burlington, MA, USA). Membranes were blocked by 5% bovine serum albumin (MRC, China) for 1 h at room temperature and then incubated with IFITM-1/-2/-3 antibody (1:1,000, Santa Cruz Biotechology, CA, USA) or GAPDH (1:8,000, Cell Signaling Technology) overnight at 4 °C. After washing three times with TBST, membranes were blocked with horseradish peroxidase conjugated secondary antibodies (CWbio, China). Subsequently, protein expression was examined by a G: BOX ChemiXT4 Imaging System (Bio-Rad) with an enhanced chemiluminescence kit (Merck Millipore, Germany).
Oncomine analysis
Oncomine (www.oncomine.org) is a comprehensive resource for counting gene expression signatures, clusters and gene-set modules (12). We used the Oncomine dataset to analyze the mRNA expression level in various cancer and related normal tissues. A P value cutoff was set at 0.001, and the log-fold change threshold was set at 2. The gene rank threshold was defined as the top 10%. The study was conducted in accordance with the Declaration of Helsinki (as revised in 2013).
Expression Atlas analysis
The expression of IFITMs in the KIRC cell line was analyzed by Expression Atlas (13) (https://www.ebi.ac.uk/gxa/home). The expression level in TPM (Transcript per million, TPM) was set at 0.5.
Human Protein Atlas analysis
The immunohistochemical profiles of normal kidney tissue and KIRC were derived from the Human Protein (14) Atlas (https://www.proteinatlas.org/). The antibodies used for IFITM-1 (https://www.proteinatlas.org/ENSG00000185885-IFITM1/pathology/renal+cancer#ihc), IFITM-2 (https://www.proteinatlas.org/ENSG00000185201-IFITM2/pathology/renal+cancer), and IFITM-3 (https://www.proteinatlas.org/ENSG00000142089-IFITM3/pathology/renal+cancer) were HPA004810, HPA004337, and HPA004337 respectively. Clinical information of the patients can be obtained from the website.
UALCAN database analysis
The UALCAN database (15) is a comprehensive web resource for studying cancer data (http://ualcan.path.uab.edu/index.html). We used it to evaluate the expression of IFITMs in KIRC based on different subtypes.
TISIDB database analysis
The TISIDB database (http://cis.hku.hk/TISIDB/index.php) is a valuable resource for cancer immunology research and therapy (16). We used it to analyze the expression of IFITMs in KIRC based on different grades and immune subtypes. We also explored the correlation between IFITMs and immune genes.
Kaplan-Meier survival analysis
We used KM Plotter for the survival analysis (17) to assess the effect of IFITMs and related immune genes on survival of KIRC (https://kmplot.com/analysis/index.php?p=background). The samples were split into high and low expression groups by the best cutoff value of mRNA expression of IFITMs.
TIMER2.0 database analysis
The TIMER2.0 database (http://timer.cistrome.org/) (18) is a web-based tool for systematic analysis of immune infiltrates across various cancer types. We explored the association between levels of IFITM gene expressions (log2 TPM), the abundance of infiltrating immune cells and the clinical relevance. Tumor purity was used for P value correction.
LinkedOmics analysis
The LinkedOmics database (19) (http://www.linkedomics.org/login.php) is a cancer-associated multi-dimensional dataset. We chose the RNAseq data type, HiSeq RNA platform and Pearson's correlation coefficient for analysis. We then used the LinkInterpreter module to perform gene set enrichment analysis (GSEA) analysis, which included gene ontology (GO) analysis, Kyoto Encyclopedia Genes and Genomes (KEGG) pathway, kinase target, miRNA target and transcription target. The minimum number of genes (size) was set at 3 and simulations were set at 500.
Database for annotation, visualization, and integrated discovery database analysis
The top 50 co-expressing co-occurrence genes with IFITMs were obtained from cBioPortal (20) (https://www.cbioportal.org/) for functional enrichment analysis. These three gene lists were put into Metascape (21) (https://metascape.org/gp/index.html#/main/step1) to draw a protein-protein interaction (PPI) network and into Network Analyst (22) (https://www.networkanalyst.ca/) for transcription factor–gene interactions and gene-miRNA interactions analyses.
TCGA portal
We obtained the relevance between IFITM mRNA expressions and the mutation rate of 9 hub genes in KIRC from the TCGA portal (23) (http://tcgaportal.org/index.html).
Statistical analysis
All statistical analyses were performed using SPSS 20.0 software (IBM, USA). Two-tailed Student’s t-tests were used for comparisons between groups. One-way ANOVA with Bonferroni’s test was utilized for multiple comparisons. A two-sided P value <0.05 was considered statistically significant.
Results
Expression levels of IFITM family genes in KIRC
From the Oncomine database, we discovered that the expression levels of IFITMs were higher in cancerous tissues than in normal tissues for various types of malignancies, but especially kidney cancer (Figure 1A; specific data are shown in Table 2). The expression profile in KIRC was quite unanimous among IFITM-1, IFITM-2 and IFITM-3, suggesting an important relationship between IFITM and KIRC. Different KIRC cell lines also confirmed the significantly higher expression of these three genes than in normal renal epithelial cells (Figure 1B). As for the translational level, the Human Protein Atlas demonstrated positive expression of IFITM using immunohistochemistry (Figure 1C). We validated the higher expression of IFITM-1/-2/-3 in the KIRC cell line than in the normal kidney cell line by qPCR (Figure 1D) and western blot (Figure 1E).
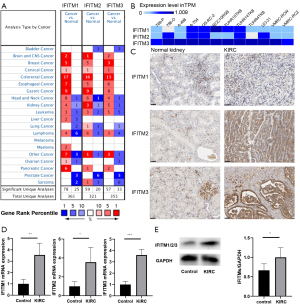
Table 2
Gene | Fold change | P value | t-test | Ref |
---|---|---|---|---|
IFITM -1 | 2.608 | 1.12E-04 | 9.735 | Higgins et al., 2003 (24) |
2.125 | 4.14E-05 | 5.229 | Lenburg et al., 2003 (25) | |
2.293 | 2.31E-06 | 6.531 | Gumz et al., 2007 (26) | |
2.227 | 1.13E-11 | 8.983 | Jones et al., 2005 (27) | |
IFITM-2 | 2.436 | 5.78E-04 | 9.329 | Higgins et al., 2003 (24) |
1.921 | 3.41E-04 | 4.221 | Lenburg et al., 2003 (25) | |
3.168 | 8.84E-08 | 8.442 | Gumz et al., 2007 (26) | |
1.836 | 2.87E-05 | 6.831 | Yusenko et al., 2009 (28) | |
IFITM-3 | 1.748 | 1.34E-06 | 7.174 | Lenburg et al., 2003 (25) |
2.603 | 2.43E-08 | 9.263 | Gumz et al., 2007 (26) | |
1.779 | 4.59E-05 | 7.178 | Yusenko et al., 2009 (28) |
IFITM, interferon-inducible transmembrane; KIRC, kidney renal clear cell carcinoma.
Clinical significance of IFITM in RCC
KIRC can be further subdivided into two subtypes, ccA and ccB, which are characterized by excessive angiogenesis and better prognosis, and by abundant macrophage infiltration and worse prognosis, respectively. From the UALCAN database, these subtypes exhibited a high level of IFITM family expression (Figure 2A). Moreover, the expression of the three IFITM members positively correlated with tumor grade (Figure 2B). By applying the Kaplan-Meier method, we found that each of the FITM isoform negatively correlated with overall survival in KIRC patients (Figure 2C). And the diagnostic role of IFITMs in KIRC was assessed by receiver operating characteristic (ROC) curve analysis (based on TCGA normal kidney samples and KIRC samples). The area under the ROC curve (AUC) analysis displayed that each of the IFITM isoform (IFITM1, IFITM2 and IFITM3) was sensitive and specific for the diagnosis of KIRC (AUC =0.7721, 0.7280, 0.8292, respectively) (Figure 2D).
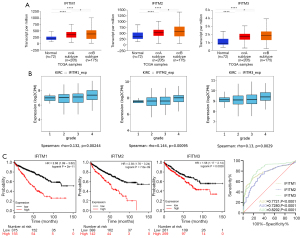
Correlation of IFITMs with immune infiltrates in KIRC
For the different immune subtypes, the expression level of the IFITM family was lowest in the immunologically quiet subtype and highest in the tumor growth factor (TGF)-β dominant subtype (Figure 3A). The correlations between the IFITMs and the immune genes are listed in Table 3. The TIMER2.0 database was used to analyze the relationships between the IFITM family and TIL in KIRC. It showed that cancer associated fibroblasts (CAFs), endothelial cells (ECs), myeloid cells, dendritic cells and natural killer (NK) cell infiltrations significantly correlated with the expression of IFITM-1, -2 and -3 (Figure 3B). CAFs and ECs were further analyzed for their role in survival. Notably, the infiltration of CAFs was significant for survival only when IFITMs expression was low, whereas an abundance of ECs positively correlated with KIRC survival irrespective of the expression level of IFITMs (Figure 3C).
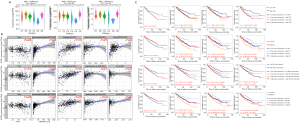
Table 3
Immune genes | Spearman rho | Overall survival in KIRC | ||||
---|---|---|---|---|---|---|
IFITM-1 | IFITM-2 | IFITM-3 | HR | Log-rank P | ||
Immunoinhibitors | ||||||
TGFB1 | 0.499 | 0.526 | 0.505 | 1.67 (1.23–2.27) | 0.00092 | |
LGALS9 | 0.485 | 0.387 | 0.446 | 1.96 (1.45–2.65) | 6.90E-06 | |
ADORA2A | 0.448 | 0.283 | 0.337 | 0.7 (0.52–0.94) | 0.017 | |
Immunostimulators | ||||||
TNFRSF4 | 0.486 | 0.553 | 0.534 | 0.75 (0.54–1.04) | 0.083 | |
TNFRSF8 | 0.476 | 0.401 | 0.422 | 1.64 (1.21–2.22) | 0.0012 | |
TNFRSF17 | 0.443 | 0.448 | 0.382 | 1.54 (1.12–2.11) | 0.0075 | |
TNFRSF18 | 0.472 | 0.4 | 0.465 | 0.45 (0.34–0.61) | 9.20E-08 | |
CXCR4 | 0.469 | 0.447 | 0.417 | 1.67 (1.24–2.26) | 0.00068 | |
Chemokines | ||||||
CCL5 | 0.434 | 0.264 | 0.364 | 0.57 (0.42–0.78) | 0.00038 | |
Receptors | ||||||
CCR10 | 0.432 | 0.469 | 0.446 | 1.55 (1.13–2.12) | 0.006 |
Data from TISIDB and KM Plotter. HR, Hazard Ratio; IFITM, interferon-inducible transmembrane; KIRC, kidney renal clear cell carcinoma.
GSEA and regulation network of IFITMs in KIRC
GSEA delineated the enrichment profile of IFITMs in KIRC, showing that biological processes such as the response to type I interferon, extracellular structure organization and vasculogenesis were significantly enriched in the GO analysis (Figure 4A), whereas NK cell cytotoxicity, ribosome activity and cytokine-cytokine receptor interaction were enriched in the KEGG analysis (Figure 4B). Furthermore, cBioPortal provided a series of genes that co-expressed with IFITMs, with which we were able to develop a PPI network (Figure 5A). To explore the upstream regulation relationship of IFITMs, we utilized the ChIP-seq data from the ENCODE database to define the transcription factor-gene interactions (Figure 5B). The miRNA-gene interactions of IFITMs and the co-expressing genes were also analyzed using TarBase and miRTarBase (Figure 5C). As can be seen in Table 4, the putative miRNAs that bound to the 3’-UTR of IFITMs were miR-433, miR-302c and miR-323.
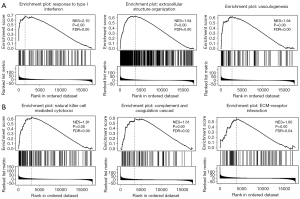
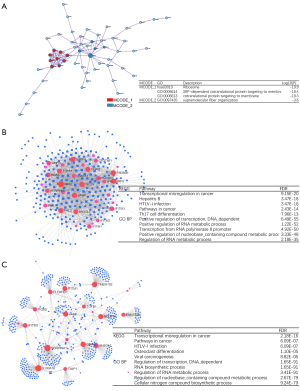
Table 4
Gene | Enriched category | Gene set | Leading edge number | P value | FDR |
---|---|---|---|---|---|
IFITM-1 | miRNA target | GGGGCCC, MIR-296 | 34 | 0 | 0.012 |
ATCATGA, MIR-433 | 25 | 0 | 0.101 | ||
ATGTTAA, MIR-302C | 65 | 0 | 0.141 | ||
TAATGTG, MIR-323 | 59 | 0 | 0.171 | ||
CCAGGGG, MIR-331 | 32 | 0.005 | 0.173 | ||
Kinase target | Kinase_LCK | 24 | 0 | 0 | |
Transcription factor | V$CREL_01 | 97 | 0 | 0.001 | |
V$IRF7_01 | 102 | 0 | 0.002 | ||
V$NERF_Q2 | 106 | 0 | 0.002 | ||
V$ELF1_Q6 | 99 | 0 | 0.002 | ||
V$SRF_Q6 | 86 | 0 | 0.003 | ||
IFITM-2 | miRNA target | TAATGTG, MIR-323 | 53 | 0 | 0 |
ATCATGA, MIR-433 | 40 | 0 | 0 | ||
ATGTTAA, MIR-302C | 87 | 0 | 0 | ||
ATTACAT, MIR-380-3P | 25 | 0 | 0.009 | ||
TACAATC, MIR-508 | 21 | 0 | 0.011 | ||
Kinase target | Kinase_PAK1 | 17 | 0 | 0.005 | |
Transcription factor | V $ STAT5B_01 | 70 | 0 | 0.002 | |
V $ SRF_Q5_01 | 77 | 0 | 0.002 | ||
V $ LFA1_Q6 | 78 | 0 | 0.003 | ||
GGGNNTTTCC_V $ NFKB_Q6_01 | 46 | 0 | 0.003 | ||
V $ SP1_Q6_01 | 65 | 0 | 0.004 | ||
IFITM-3 | miRNA target | TGAATGT, MIR-181A, MIR-181B, MIR-181C, MIR-181D | 176 | 0 | 0 |
ATTACAT, MIR-380-3P | 29 | 0 | 0 | ||
TAATGTG, MIR-323 | 68 | 0 | 0 | ||
ATCATGA, MIR-433 | 40 | 0 | 0 | ||
ATGTTAA, MIR-302C | 78 | 0 | 0 | ||
CATTTCA, MIR-203 | 88 | 0 | 0.001 | ||
Kinase target | Kinase_PAK1 | 50 | 0 | 0 | |
Kinase_IKBKB | 28 | 0 | 0.024 | ||
Transcription factor | V $ SP1_Q6_01 | 72 | 0 | 0.004 | |
GGGNNTTTCC_V $ NFKB_Q6_01 | 54 | 0 | 0.004 | ||
V $ LFA1_Q6 | 79 | 0 | 0.004 | ||
V $ STAT5B_01 | 82 | 0 | 0.004 | ||
V $ NERF_Q2 | 73 | 0 | 0.004 |
Data from LinkedOmics. IFITM, interferon-inducible transmembrane; KIRC, kidney renal clear cell carcinoma; FDR, false discovery rate.
Correlation of the expression if IFITMs with significantly mutated genes in KIRC
Nine hub genes have found to be related to KIRC, including VHL, PMRM1, and SETD2. As the IFITM1–3 mRNA expression increased, the mutation rate of PBRM1, SETD2 and PTEN mRNA increased. As the IFITM-1 and IFITM-3 mRNA expression increased, the mutation rate of TP53 mRNA increased (Figure 6).
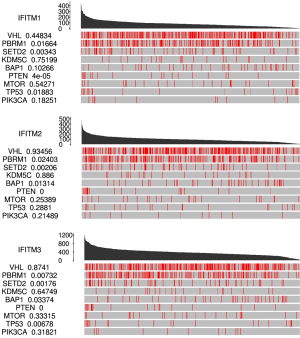
Discussion
IFITMs, initially identified as interferon-stimulated genes (ISGs), are a family of small homologous proteins that confer restrictions on viral entry (29). Recent discoveries demonstrated that IFITMs are expressed in human lymphocytes and influence T cell differentiation and are involved in cytokine signaling regulation (30). Because IFITMs are transcriptional targets of Wnt and Hedgehog signaling (31,32), it is possible that they also play an essential role in carcinogenesis. Indeed, the IFITM family is a prognostic indicator for colorectal cancer, gallbladder cancer and acute myeloid leukemia (10,31,33). Their effect on KIRC, however, is seldom discussed.
In this study, we utilized TCGA data and multiple online analysis tools to delineate the role of IFITMs in KIRC and found that IFITM proteins 1, 2 and 3 were all overexpressed in cancerous tissues compared with adjacent normal tissues at both the transcriptional and translational level. Kaplan-Meier analysis showed that higher expression of IFITMs was associated with a worse prognosis. Moreover, enrichment analysis demonstrated that biological processes such as the response to type I interferon, extracellular structure organization and carcinogenesis were highly enriched in the GO analysis. In contrast, NK cell cytotoxicity, ribosome activity and cytokine-cytokine receptor interaction were enriched in the KEGG analysis. The regulation network was further established based on the miRNA-mRNA and PPI interaction prediction tools. As a result, we found that miR-433, miR-302c and miR-323 possessed a putative binding sequence to the 3'-UTR of IFITMs. For an evaluation of protein interaction, interferon regulatory factor 9 (IRF9) and interferon alpha inducible protein 6 (IFI6) were predicted to be targets of IFITMs. IRF9 is a transcription factor that mediates type I IFN signaling, leading to tyrosine phosphorylation of STAT1 and STAT2, thus activating the JAK/STAT pathway (34). IFI6 is also induced by interferon and regulates tumor cell apoptosis (35). Together with IFITMs, IRF9 and IFI6 might constitute part of the innate immune response against KIRC. Sure enough, the host immunity toward KIRC was quite active according to our findings from the immune cell infiltration analysis, in which myeloid cells, dendritic cells and NK cell infiltrations significantly correlated with the expression of IFITMs. Cytokine-cytokine receptor interaction and JAK/STAT pathway activation by IFITMs and its targets might be contributed to recruit immune cell infiltration into tumor microenvironment of KIRC.
As for the different immune subtypes, the expression of IFITMs was markedly lower in the immunologically quiet subtype and higher in the TGF-β dominant subtype, indicating that the level of IFITMs might represent the level of immune activity. As a common sense, more immune cell infiltration was beneficial for prognosis. But in the present study, we found that high expression of IFITMs indicated a poor prognosis and more immune cell infiltration. It might be on this account that tumor-infiltrating cells acted a multifaceted role (tumor-suppressive or tumor-enhanced effects) depending on the different cancer types (6). Unlike most other cancer types, high CD8 T cell infiltration in KIRC predicted poor outcomes. The CD8 TILs were found to be abundant in KIRC, but they were functionally and metabolically impaired, and lost tumor-killing activities, thereby suppressed its antitumor immunity in KIRC (7).
Targeted therapies directed against VEGFR and mammalian target of rapamycin (mTOR) were approved for RCC as early as 2005 (36). These regimens were developed based on the observation that VHL was often altered in sporadic RCC (37). Although these regimens were designated to address altered VHL biology, treatment response was not dependent on the absence or presence of VHL alterations (38). Biomarkers for treatment response were later revealed, as the alterations in chromatin-remodeling genes such as PBRM1, BAP1, SETD2 and EZH2 were found to be closely associated with clinical outcomes (39-42). Among them, PBRM1, BAP1 and SETD2 are all localized in close proximity on the short arm of chromosome 3. PBRM1 is the major component of the PBAF (Polybromo-associated BAF) complex. Although PBRM1 has been reported to be a tumor suppressor in KIRC by regulating cellular proliferation and differentiation, the mutation of this gene is the second most common somatic alteration in KIRC and is detected in ≈30% of patients (43,44). The loss of PBRM1 might upregulate HIF and STAT3 and thus strengthen immune surveillance against cancer (45). SETD2 is a histone H3 K36 methyltransferase that modulates chromatin biology and regulates gene transcription and DNA repair. Mutation of SETD2 invariably coexists with mutation of PBRM1 (46). Evidence revealed that the level of mutation positively correlates with stages of the disease, suggesting a preventive role against tumor metastasis of the wild-type SETD2 (47). BAP1 is a two-hit tumor suppressor gene. Unlike SETD2, the simultaneous loss of both BAP1 and PBRM1 is seen in only 3% of cases (48). In addition, there is almost no overlap between the gene signatures of BAP1and PBRM1 mutation, suggesting a mutually exclusive oncogenic mechanism (40). According to our correlation analysis of IFITM expressions and gene mutations, the mutations of PBRM1, BAP1 and SETD2 were all significantly correlated with the three IFITM genes’ expression. These results further suggested that IFITMs might play a crucial role in the oncogenesis of KIRC and could be a potential surrogate marker for treatment response to targeted therapies.
We have several limitations to our study. Firstly, the oncogenic role and regulation interaction networks of IFITM have yet to be validated by additional cellular and animal experiments in further study. Additionally, correlation analysis between the expression of IFITMs and the treatment response to targeted therapies is warranted with real-world data.
Conclusions
The discoveries in our study help our understanding of the role of IFITMs in the oncogenesis of KIRC and suggest avenues for further research on new diagnostic and therapeutic targets of KIRC.
Acknowledgments
Funding: This work was supported by grants from the Natural Science Foundation of Guangdong Province (2020A1515010115), the Fundamental Research Funds for the Central University (20ykpy109, 19ykpy103).
Footnote
Reporting Checklist: The authors have completed the REMARK reporting checklist. Available at https://dx.doi.org/10.21037/tau-21-848
Data Sharing Statement: Available at https://dx.doi.org/10.21037/tau-21-848
Conflicts of Interest: All authors have completed the ICMJE uniform disclosure form (available at https://dx.doi.org/10.21037/tau-21-848). All authors reported that this work was supported by grants from the Natural Science Foundation of Guangdong Province (2020A1515010115) and the Fundamental Research Funds for the Central University (20ykpy109, 19ykpy103). The authors have no other conflicts of interest to declare.
Ethical Statement: The authors are accountable for all aspects of the work in ensuring that questions related to the accuracy or integrity of any part of the work are appropriately investigated and resolved. The study was conducted in accordance with the Declaration of Helsinki (as revised in 2013).
Open Access Statement: This is an Open Access article distributed in accordance with the Creative Commons Attribution-NonCommercial-NoDerivs 4.0 International License (CC BY-NC-ND 4.0), which permits the non-commercial replication and distribution of the article with the strict proviso that no changes or edits are made and the original work is properly cited (including links to both the formal publication through the relevant DOI and the license). See: https://creativecommons.org/licenses/by-nc-nd/4.0/.
References
- Barata PC, Rini BI. Treatment of renal cell carcinoma: Current status and future directions. CA Cancer J Clin 2017;67:507-24. [Crossref] [PubMed]
- Srigley JR, Delahunt B, Eble JN, et al. The International Society of Urological Pathology (ISUP) Vancouver Classification of Renal Neoplasia. Am J Surg Pathol 2013;37:1469-89. [Crossref] [PubMed]
- Hsieh JJ, Le VH, Oyama T, et al. Chromosome 3p Loss-Orchestrated VHL, HIF, and Epigenetic Deregulation in Clear Cell Renal Cell Carcinoma. J Clin Oncol 2018; Epub ahead of print. [Crossref] [PubMed]
- Vuong L, Kotecha RR, Voss MH, et al. Tumor Microenvironment Dynamics in Clear-Cell Renal Cell Carcinoma. Cancer Discov 2019;9:1349-57. [Crossref] [PubMed]
- Motzer RJ, Tannir NM, McDermott DF, et al. Nivolumab plus Ipilimumab versus Sunitinib in Advanced Renal-Cell Carcinoma. N Engl J Med 2018;378:1277-90. [Crossref] [PubMed]
- Şenbabaoğlu Y, Gejman RS, Winer AG, et al. Tumor immune microenvironment characterization in clear cell renal cell carcinoma identifies prognostic and immunotherapeutically relevant messenger RNA signatures. Genome Biol 2016;17:231. [Crossref] [PubMed]
- Siska PJ, Beckermann KE, Mason FM, et al. Mitochondrial dysregulation and glycolytic insufficiency functionally impair CD8 T cells infiltrating human renal cell carcinoma. JCI Insight 2017;2:e93411 [Crossref] [PubMed]
- Diamond MS, Farzan M. The broad-spectrum antiviral functions of IFIT and IFITM proteins. Nat Rev Immunol 2013;13:46-57. [Crossref] [PubMed]
- Wrensch F, Ligat G, Heydmann L, et al. Interferon-Induced Transmembrane Proteins Mediate Viral Evasion in Acute and Chronic Hepatitis C Virus Infection. Hepatology 2019;70:1506-20. [Crossref] [PubMed]
- Liu Y, Lu R, Cui W, et al. High IFITM3 expression predicts adverse prognosis in acute myeloid leukemia. Cancer Gene Ther 2020;27:38-44. [Crossref] [PubMed]
- Xu L, Zhou R, Yuan L, et al. IGF1/IGF1R/STAT3 signaling-inducible IFITM2 promotes gastric cancer growth and metastasis. Cancer Lett 2017;393:76-85. [Crossref] [PubMed]
- Rhodes DR, Kalyana-Sundaram S, Mahavisno V, et al. Oncomine 3.0: genes, pathways, and networks in a collection of 18,000 cancer gene expression profiles. Neoplasia 2007;9:166-80. [Crossref] [PubMed]
- Papatheodorou I, Fonseca NA, Keays M, et al. Expression Atlas: gene and protein expression across multiple studies and organisms. Nucleic Acids Res 2018;46:D246-51. [Crossref] [PubMed]
- Uhlen M, Zhang C, Lee S, et al. A pathology atlas of the human cancer transcriptome. Science 2017;357:eaan2507 [Crossref] [PubMed]
- Chandrashekar DS, Bashel B, Balasubramanya SAH, et al. UALCAN: A Portal for Facilitating Tumor Subgroup Gene Expression and Survival Analyses. Neoplasia 2017;19:649-58. [Crossref] [PubMed]
- Ru B, Wong CN, Tong Y, et al. TISIDB: an integrated repository portal for tumor-immune system interactions. Bioinformatics 2019;35:4200-2. [Crossref] [PubMed]
- Nagy Á, Lánczky A, Menyhárt O, et al. Validation of miRNA prognostic power in hepatocellular carcinoma using expression data of independent datasets. Sci Rep 2018;8:9227. [Crossref] [PubMed]
- Li T, Fu J, Zeng Z, et al. TIMER2.0 for analysis of tumor-infiltrating immune cells. Nucleic Acids Res 2020;48:W509-14 [Crossref] [PubMed]
- Vasaikar SV, Straub P, Wang J, et al. LinkedOmics: analyzing multi-omics data within and across 32 cancer types. Nucleic Acids Res 2018;46:D956-63. [Crossref] [PubMed]
- Cerami E, Gao J, Dogrusoz U, et al. The cBio cancer genomics portal: an open platform for exploring multidimensional cancer genomics data. Cancer Discov 2012;2:401-4. [Crossref] [PubMed]
- Zhou Y, Zhou B, Pache L, et al. Metascape provides a biologist-oriented resource for the analysis of systems-level datasets. Nat Commun 2019;10:1523. [Crossref] [PubMed]
- Zhou G, Soufan O, Ewald J, et al. NetworkAnalyst 3.0: a visual analytics platform for comprehensive gene expression profiling and meta-analysis. Nucleic Acids Res 2019;47:W234-41 [Crossref] [PubMed]
- Xu S, Feng Y, Zhao S. Proteins with Evolutionarily Hypervariable Domains are Associated with Immune Response and Better Survival of Basal-like Breast Cancer Patients. Comput Struct Biotechnol J 2019;17:430-40. [Crossref] [PubMed]
- Higgins JP, Shinghal R, Gill H, et al. Gene expression patterns in renal cell carcinoma assessed by complementary DNA microarray. Am J Pathol 2003;162:925-32. [Crossref] [PubMed]
- Lenburg ME, Liou LS, Gerry NP, et al. Previously unidentified changes in renal cell carcinoma gene expression identified by parametric analysis of microarray data. BMC Cancer 2003;3:31. [Crossref] [PubMed]
- Gumz ML, Zou H, Kreinest PA, et al. Secreted frizzled-related protein 1 loss contributes to tumor phenotype of clear cell renal cell carcinoma. Clin Cancer Res 2007;13:4740-9. [Crossref] [PubMed]
- Jones J, Otu H, Spentzos D, et al. Gene signatures of progression and metastasis in renal cell cancer. Clin Cancer Res 2005;11:5730-9. [Crossref] [PubMed]
- Yusenko MV, Zubakov D, Kovacs G. Gene expression profiling of chromophobe renal cell carcinomas and renal oncocytomas by Affymetrix GeneChip using pooled and individual tumours. Int J Biol Sci 2009;5:517-27. [Crossref] [PubMed]
- Brass AL, Huang IC, Benita Y, et al. The IFITM proteins mediate cellular resistance to influenza A H1N1 virus, West Nile virus, and dengue virus. Cell 2009;139:1243-54. [Crossref] [PubMed]
- Yánez DC, Sahni H, Ross S, et al. IFITM proteins drive type 2 T helper cell differentiation and exacerbate allergic airway inflammation. Eur J Immunol 2019;49:66-78. [Crossref] [PubMed]
- Andreu P, Colnot S, Godard C, et al. Identification of the IFITM family as a new molecular marker in human colorectal tumors. Cancer Res 2006;66:1949-55. [Crossref] [PubMed]
- Furmanski AL, Barbarulo A, Solanki A, et al. The transcriptional activator Gli2 modulates T-cell receptor signalling through attenuation of AP-1 and NFκB activity. J Cell Sci 2015;128:2085-95. [Crossref] [PubMed]
- Li D, Yang Z, Liu Z, et al. DDR2 and IFITM1 Are Prognostic Markers in Gallbladder Squamous Cell/Adenosquamous Carcinomas and Adenocarcinomas. Pathol Oncol Res 2019;25:157-67. [Crossref] [PubMed]
- Fink K, Martin L, Mukawera E, et al. IFNβ/TNFα synergism induces a non-canonical STAT2/IRF9-dependent pathway triggering a novel DUOX2 NADPH oxidase-mediated airway antiviral response. Cell Res 2013;23:673-90. [Crossref] [PubMed]
- Qadir F, Aziz MA, Sari CP, et al. Transcriptome reprogramming by cancer exosomes: identification of novel molecular targets in matrix and immune modulation. Mol Cancer 2018;17:97. [Crossref] [PubMed]
- Rini BI, Small EJ. Biology and clinical development of vascular endothelial growth factor-targeted therapy in renal cell carcinoma. J Clin Oncol 2005;23:1028-43. [Crossref] [PubMed]
- Garcia JA, Rini BI. Recent progress in the management of advanced renal cell carcinoma. CA Cancer J Clin 2007;57:112-25. [Crossref] [PubMed]
- Ricketts CJ, De Cubas AA, Fan H, et al. The Cancer Genome Atlas Comprehensive Molecular Characterization of Renal Cell Carcinoma. Cell Rep 2018;23:313-326.e5. [Crossref] [PubMed]
- Ho TH, Choueiri TK, Wang K, et al. Correlation Between Molecular Subclassifications of Clear Cell Renal Cell Carcinoma and Targeted Therapy Response. Eur Urol Focus 2016;2:204-9. [Crossref] [PubMed]
- Peña-Llopis S, Vega-Rubín-de-Celis S, Liao A, et al. BAP1 loss defines a new class of renal cell carcinoma. Nat Genet 2012;44:751-9. [Crossref] [PubMed]
- Sato Y, Yoshizato T, Shiraishi Y, et al. Integrated molecular analysis of clear-cell renal cell carcinoma. Nat Genet 2013;45:860-7. [Crossref] [PubMed]
- Adelaiye-Ogala R, Budka J, Damayanti NP, et al. EZH2 Modifies Sunitinib Resistance in Renal Cell Carcinoma by Kinome Reprogramming. Cancer Res 2017;77:6651-66. [Crossref] [PubMed]
- Varela I, Tarpey P, Raine K, et al. Exome sequencing identifies frequent mutation of the SWI/SNF complex gene PBRM1 in renal carcinoma. Nature 2011;469:539-42. [Crossref] [PubMed]
- Hodges C, Kirkland JG, Crabtree GR. The Many Roles of BAF (mSWI/SNF) and PBAF Complexes in Cancer. Cold Spring Harb Perspect Med 2016;6:a026930 [Crossref] [PubMed]
- Nargund AM, Pham CG, Dong Y, et al. The SWI/SNF Protein PBRM1 Restrains VHL-Loss-Driven Clear Cell Renal Cell Carcinoma. Cell Rep 2017;18:2893-906. [Crossref] [PubMed]
- Peña-Llopis S, Christie A, Xie XJ, et al. Cooperation and antagonism among cancer genes: the renal cancer paradigm. Cancer Res 2013;73:4173-9. [Crossref] [PubMed]
- Hsieh JJ, Chen D, Wang PI, et al. Genomic Biomarkers of a Randomized Trial Comparing First-line Everolimus and Sunitinib in Patients with Metastatic Renal Cell Carcinoma. Eur Urol 2017;71:405-14. [Crossref] [PubMed]
- Joseph RW, Kapur P, Serie DJ, et al. Clear Cell Renal Cell Carcinoma Subtypes Identified by BAP1 and PBRM1 Expression. J Urol 2016;195:180-7. [Crossref] [PubMed]