Identifying a hypoxia related score to predict the prognosis of bladder cancer: a study with The Cancer Genome Atlas (TCGA) database
Introduction
Bladder cancer is currently the 10th most frequent and common cancer worldwide, with about 200,000 deaths and 549,000 new cases being recorded in 2018 (1). Transitional cell carcinoma accounts for 90% of all bladder cancers (2). Clinically, bladder cancer is divided into metastatic bladder cancer, muscle-invasive bladder cancer (MIBC) and non-muscle-invasive bladder cancer (TaT1, CIS) (NMIBC). Several strategies such as immunotherapy have been employed in the treatment of bladder cancer, leading to a decline in bladder cancer-related mortality rates (3). However, due to the genetic instability associated with bladder cancer, there is need to improve treatment efficacy by identifying other potential therapeutic targets.
The tumor microenvironment (TME) is one of the critical regulators of cancer progression and metastasis (4). In addition, the hypoxia state that is frequently associated with the TME plays a critical role in cancer genetic instability and prognosis (5). Hypoxia is related to tumor necrosis and pronounced hypoxia has been observed in human bladder cancer tissues (6). Hypoxia can influence the effect of radiotherapy on MIBC. Moreover, chemoresistance of bladder cancer cells is also associated with hypoxia by activation of HIF-1α-associated autophagy (7). Hypoxia can also influence the genetic instability and malignant progression of MIBC, which are related to metastasis (8).
Since hypoxia plays an essential role in bladder cancer, assessing and targeting hypoxia would be beneficial for the clinical management of bladder cancer. Hypoxia-modifying therapy combined with radiotherapy has been seen to improve the survival of high-risk bladder cancer patients (9). In addition, different types of biomarkers, such as miR-210 and hypoxia-inducible factor (HIF)-1α, have been found to reflect the hypoxic state of bladder cancer (10,11). There have been a lot of prognostic biomarkers for bladder cancer. Clinicopathologic characteristics like presence of carcinoma in situ, lymphovascular invasion and micropapillary histology have been regarded as prognostic markers for NMIBC (12). Nomograms, fluorescence in situ hybridization (FISH), and many molecular biomarkers including cell cycle regulators, cell adhesion molecules have been proved as prognostic markers in previous studies. It still remains a great challenge for urologic doctors to discover which bladder cancer cases are at higher risk in prognosis and may benefit from early, more radical treatment. Recently, bioinformatics has been utilized to construct hypoxia-related models to predict the survival of cancer cases (13,14). There are also studies about identifying hypoxia-related prognostic model for bladder cancer (15,16). Different bioinformatic analysis technologies have been used to discover potential hypoxia related biomarkers. The findings of these studies pointed out some potential biomarkers and models, but there is still a long way to go for wider clinical applications. More studies are needed for enriching this research field using updating bioinformatic technologies and different verification.
In this study, gene expression profiles for bladder cancer cases obtained from The Cancer Genome Atlas (TCGA) database (https://cancergenome.nih.gov) were used to calculate the hypoxia-related score. We used this score to explore the relationship between hypoxia and outcomes of bladder cancer patients. We also established a new hypoxia-related model from the TCGA data in a new way and assessed its ability to predict outcomes for bladder cancer using data from the Gene Expression Omnibus (GEO) database (https://www.ncbi.nlm.nih.gov/geo). Findings from this study may give potential insights for clinical decision making and treatment of bladder cancer. Figure 1 shows the study workflow. We present the following article in accordance with the REMARK reporting checklist (available at https://dx.doi.org/10.21037/tau-21-569).
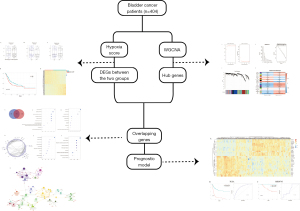
Methods
Database
We downloaded the gene expression profiles and clinical characteristics of bladder cancer cases from the TCGA database (March 2020). We excluded the bladder cancer cases without pathological diagnosis. The study was conducted in accordance with the Declaration of Helsinki (as revised in 2013).
Hypoxia score calculation
We utilized a 26-gene hypoxia signature and a gene set variation analysis (GSVA) to compute the hypoxia score (17,18). There is evidence indicating that the 26-gene hypoxia signature is a measure of tumor hypoxia. GSVA is recognized as a gene set enrichment tool for RNA-seq data that assesses variation of pathway activity. The GSVA algorithm was used to evaluate the GSVA score to reveal the hypoxia status of each cancer case. The cancer cases were grouped into low- and high-hypoxia score groups using the survminer package in R based on an optimal cut-off value. The P value of survival curves was minimized with such grouping. In addition, we applied a t-test to evaluate the variations between these two groups in other clinical characteristics.
Differentially expressed genes (DEGs) identification
We identified DEGs between low- and high- hypoxia score groups using the Bioconductor package, edgeR, with the fold change (|fold change| ≥1.5) and adj. P<0.05. We then used the pheatmap package in R to generate heatmaps for the DEGs. The overlapping DEGs were subjected to further analysis.
Weighted gene co-expression network analysis (WGCNA) used to hypoxia-related genes identification
We generated co-expression networks using the WGCNA package in R (19). We then selected the top 9,829 genes for further analysis based on the standard deviation. We chose the soft threshold value, β =3 for the highest mean connectivity. We defined the interpretation of gene expression profile using module eigengenes (ME), then associated it with hypoxia feature. Genes of the module with the highest correlation were considered to be hypoxia-related genes.
Construction of PPI network and functional enrichment analysis
We utilized the online Venn diagram analysis tool to identify the overlapping genes between DEGs and hypoxia-related genes (https://bioinformatics.psb.ugent.be/webtools/Venn/). Thereafter, we constructed a PPI network using the STRING database (20), And visualized the PPI network using Cytoscape software (21). Cytoscape ClueGo and CluePedia were used to visualize the interaction network of biological concept enrichment analysis. We used the clusterprofiler package in R for functional enrichment analysis and KEGG pathway enrichment analysis (22). We set the false discovery rate (FDR) at 0.05.
Hypoxia-related signature construction and external validation
We applied LASSO (the least absolutes shrinkage and selection operator) in inferring the overlapping genes in multivariate Cox regression analysis with R package glmnet. The pheatmap package in R was used to generate the heatmap of selected genes. We used the regression coefficients obtained from the multivariate Cox regression to calculate the hypoxia risk scores using gene expression multiplied by a linear combination of the regression coefficients. Using the survminer package in R, we grouped the cancer cases to low- and high-hypoxia risk groups based on the optimal cut-off value. We also used the ROCR package in R to conduct the Kaplan-Meier analysis and ROC curves. Finally, we used the GSE69795 dataset downloaded from the GEO database to validate the hypoxia-related signature model.
Statistical analysis
The t-test was applied for comparisons as appropriate. The LASSO regression and multivariate Cox regression analyses were applied for hypoxia-related signature construction. The Kaplan-Meier survival curve and log-rank test were used for survival analysis. ROC curves were presented to evaluate the accuracy of the model. Statistical analyses were conducted using R software 3.6.3. A two-sided P<0.05 was considered statistically significant.
Results
Evaluation of hypoxia score and comparison of gene expression profiles
After exclusion of bladder cancer cases without follow-up information or survival time, 404 bladder cancer cases were included for further analysis.
The hypoxia scores ranged from −0.733 to 0.717, with the optimal cut-off value of −0.3 being used to group the bladder cancer cases into low- and high- hypoxia score groups (Figure S1). Figure 2 shows that there was no significant difference in hypoxia scores when the cancer cases were grouped based on the TNM tumor stage (Figure 2A) and the absence or presence of distant metastatic lesions (M0, M1) (Figure 2C). However, the hypoxia score was significantly lower in cases without lymph node metastasis (n=0) (P=0.009), shown in Figure 2B. Results of Kaplan-Meier analysis showed in Figure 2D that patients with higher hypoxia scores had a significantly poor overall survival (log-rank test P=0.017). Figure 2E shows the heatmap displaying distinct gene expression profiles of patients separately grouped into low- and high- hypoxia score groups.
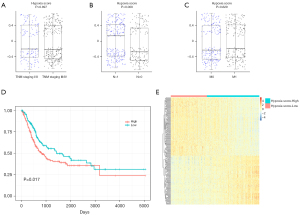
DEGs expression analysis was conducted to identify the link between gene expression and hypoxia score. A total of 270 DEGs were identified, with 123 of the genes being downregulated and 147 of the genes being upregulated.
Identification of hypoxia-related genes using WGCNA
The top 9,829 genes of 19,658 genes were selected based on the standard deviation (Figure 3A-3C). Co-expression network analysis identified 20 gene modules. Figure 3D showed the further correlation analysis between ME and hypoxia score. And the blue module had the highest correlation to hypoxia (module-trait relationships =−0.45, P<0.001). The 1,313 genes in the blue module were thus considered to be hypoxia-related genes.
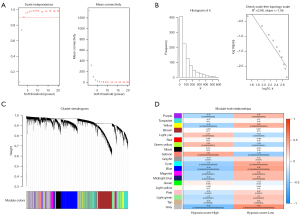
Overlap genes for PPI network construction and functional enrichment analysis
Figure 4A shows that 170 genes overlapped between DEGs and hypoxia-related genes. We constructed PPI network using the STRING tool with confidence >0.4 to probe the interactions among the 170 overlapping genes, shown in Figure 4B. The network involved 108 nodes and 166 edges, while KRT5, CD44, SNAI2, COL17A1, and AR were found as remarkable nodes with more connections with other nodes.
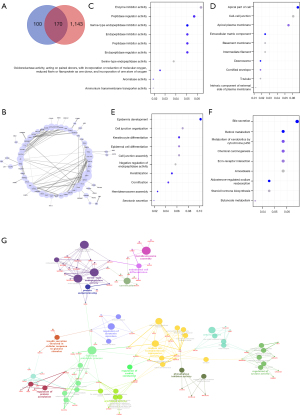
We also conducted GO and KEGG analysis to assess the biological significance of the overlapped genes. The genes were enriched in 11 cellular component (CC) terms, 29 molecular function (MF) terms, and 127 biological process (BP) terms. The top GO terms, including enzyme inhibitor activity, peptidase regulator activity, apical part of cell, cell-cell junction, cell junction organization, and epidermis development, are shown in Figure 4C-4E. KEGG analysis showed that the overlapping genes were involved in 9 pathways (Figure 4F), including metabolism of xenobiotics by cytochrome P450, retinol metabolism, etc.
In Figure 4G, we further used ClueGO Cytoscape plug-in to identify enriched pathways for overlapped genes and determine interconnection between each gene cluster. Serine-type endopeptidase activity, regulation of protein acetylation, hormone metabolic process, sodium ion transmembrane transporter activity, regulation of serotonin secretion, aromatase activity, phosphatase inhibitor activity, regulation of cyclase activity, and urea transport were enriched for BPs. Genes such as F3, COL7A1, TMPRSS2, SLC5A7, SULT1E1, SDR16C5, SNAI2, CYP1A1, TESC, UPK3A, PDZD3, and ADORA2B, were also enriched.
Identification of prognostic markers and construction of a prognostic risk model
LASSO algorithms were used to identify the prognostic markers and a total of 29 genes were selected to construct a 29-gene signature model. The genes include ACSM6, AHNAK2, AIM2, ARHGEF4, B3GAT1, BARX2, BHMT, CASQ1, CCL15, CDH3, CPA4, CYP4B1, DSC3, EREG, FOSL1, FREM1, GSDMC, HES2, HNF1B, HTR7, IGDCC3, KLK6, PLIN5, PTPRZ1, SCUBE2, SERPINB2, SH3RF2, SLC14A1, and SLC30A2. Figure 5A shows the heatmap of the gene expression patterns of the selected genes.
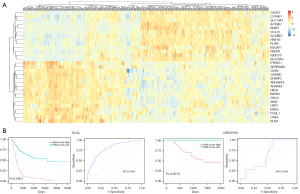
In the established model, the risk score =(−0.0448363)* Expression (ACSM6) + 0.1245315* Expression (AHNAK2) + (−0.1105080)* Expression (AIM2) + (−0.0070491)* Expression (ARHGEF4) + (−0.0008498)* Expression (B3GAT1) + 0.0402872* Expression (BARX2) + (−0.0338227)* Expression (BHMT) + (−0.1052397)* Expression (CASQ1) + (−0.2276052)* Expression (CCL15) + (−0.1386354)* Expression (CDH3) + 0.1193280* Expression (CPA4) + 0.1602697* Expression (CYP4B1) + (−0.0056484) * Expression (DSC3) + 0.0444021* Expression (EREG) + 0.1306001* Expression (FOSL1) + 0.2226840* Expression (FREM1) + (−0.2024668) * Expression (GSDMC) + 0.0534852* Expression (HES2) + (−0.2341762) * Expression (HNF1B) + 0.1087251* Expression (HTR7) + 0.1687725* Expression (IGDCC3) + 0.0050696* Expression (KLK6) + (−0.0605225) * Expression (PLIN5) + 0.0359618* Expression (PTPRZ1) + 0.1501826* Expression (SCUBE2) + 0.1281557* Expression (SERPINB2) + (−0.1867163) * Expression (SH3RF2) + 0.0372838* Expression (SLC14A1) + 0.0223318* Expression (SLC30A2).
The prognostic risk model was assessed using ROC curve and Kaplan-Meier analysis. In the TCGA cohort, the model had an accuracy of 0.802 (95% CI: 0.759–0.844). Bladder cancer cases with low-risk scores had significantly longer overall survival (P<0.0001), shown in Figure 5B. The survival rate of patients in low-risk group was much higher than those in high-risk group (71.06% vs. 23.66%). The patients in low-risk group hadn’t reach a median survival time, while the patients in high-risk group had a median survival time for 1.36 years. Adjusting for confounding clinical variables including age, gender, TNM staging, Cox proportional hazards regression analysis showed that the risk model was an independent better predictor of prognosis for bladder cancer with hazard ratio (HR)= 1.789 (95% CI: 1.585–2.019) (P<0.001) (Table 1). The prognostic value of the risk model was validated using an external dataset GSE69795. Consistently, the results showed that high-risk score cancer cases had significantly shorter overall survival (P=0.0079) and the AUC was 0.631 (95% CI: 0.449–0.813) (Figure 5C).
Table 1
Parameter | Univariate analysis | Multivariate analysis | |||||
---|---|---|---|---|---|---|---|
HR | 95% CI | P | HR | 95% CI | P | ||
Age | 1.846 | (1.261–2.710) | 0.002 | 1.612 | (1.095–2.373) | 0.015 | |
Gender | 0.841 | (0.609–1.162) | 0.294 | 0.841 | (0.606–1.168) | 0.302 | |
T staging | 1.967 | (1.408–2.746) | <0.001 | 1.355 | (0.955–1.921) | 0.089 | |
N staging | 2.053 | (1.527–2.761) | <0.001 | 1.423 | (1.042–1.943) | 0.027 | |
M staging | 1.470 | (1.092–1.978) | 0.011 | 1.179 | (0.873–1.593) | 0.284 | |
Risk score | 1.904 | (1.697–2.135) | <0.001 | 1.789 | (1.585–2.019) | <0.001 |
HR, hazard ratio.
Discussion
Previous studies have indicated that hypoxia is a distinguishing feature in solid tumors. Hypoxia profoundly affects the mitochondria and mitochondrial metabolism in cancer cells. The HIF family is very important in the adaption of cancer cell metabolism to hypoxia (23). It has been shown that HIFs enhance the activation of genes associated with cancer metastases in melanoma. HIF-1α and HIF-2α can promote melanoma metastasis by regulating cell invasion and extracellular matrix remodeling (24). In pancreatic ductal adenocarcinoma, the hypoxic microenvironment can induce spatial transcriptome changes with different expression patterns of hypoxia-related genes (25). In non-small cell lung cancer, hypoxia also induces higher expression of antizyme inhibitors 2 (AZIN2) to contribute to the development of cisplatin resistance, by strengthening the epithelial-mesenchymal transition (EMT) (26). On the other hand, it has been demonstrated that hyperbaric oxygen promotes immune responses in solid tumors and disrupts hypoxia-mediated immunosuppression (27).
Bladder cancer hypoxia is associated with genetic instability, EMT, inhibited apoptosis, and cancer progression (8). Hypoxia has been shown to affect immunotherapy by altering molecular markers, immune cell trafficking, and angiogenesis to cause immunosuppression through a HIF-1-dependent signature (28). Evidence shows that high levels of hypoxia are associated with poor prognosis in bladder cancer. The level of hypoxia in bladder cancer can be evaluated by assessing tumor necrosis during histopathology, microRNA expression, protein expression of CAIX, HIF-1α, GLUT-1, OPN, and mRNA signatures. Recently, a four gene hypoxia-related model and a seven gene hypoxia-related model have been separately established (15,16). In this study, we used WGCNA analysis to identify hypoxia-feature genes in bladder cancer. The genes that overlapped between DEGs and Hub genes with hypoxia features were then selected for further functional analysis and construction of a new signature.
In this study, we identified 170 hypoxia-related overlapped genes. The PPI network significantly included KRT5, CD44, SNAI2, COL17A1, and AR. KPT5 has been proved to be associated with the rates of lymph nodule metastasis and lymphovascular invasion in urothelial bladder cancer (29). CD44 encodes a cell-surface glycoprotein which is associated with cell-cell interactions, cell adhesion, and migration. Attenuating CD44 expression inhibits invasion of bladder cancer (30). SNAI2 is associated with EMT in bladder cancer. Up-regulated AR enhances the cell proliferation and metastasis of bladder cancer in males (31). However, the role of COL17A1 in bladder cancer has not been reported. Functional enrichment analysis exhibited that the selected genes were exactly related to enzyme inhibitor activity, cell-cell junction, and cell junction organization. ClueGO analysis showed that genes binned into serine-type endopeptidase activity, regulation of protein acetylation, hormone metabolic process, aromatase activity, sodium ion transmembrane transporter activity, and phosphatase inhibitor activity. These results revealed that hypoxia-related energy metabolism is involved in TME condition and cancer development.
Several approaches for predicting outcome of bladder cancer based on TME hypoxia have been established (15,16). Our study established a 29-gene hypoxia-related signature with an accuracy of 0.802 (95% CI: 0.759–0.844). AHNAK2 is a member of the AHNAK family that has been identified as a new prognostic biomarker for bladder cancer cases with radical cystectomy (32). AIM2 plays a part in tumorigenic reversion and cell proliferation. The differential expression of ARHGEF4 in NMIBC was reported (33). CDH13 is hypermethylated in many types of cancer, and is used for representing the integrity of basal cell layers in the study of the luminal class of urothelial tumor (34). CYP4B1 genotypes might affect the risk of Japanese bladder cancer (35). DSC3 was discovered as an independent prognostic biomarker of tumor progression in a study comparing progressive MIBC and de novo MIBC (36). FOSL1 was highly expressed in non-papillary urothelial bladder cancer, and FOSL1-regulated transcripts were strongly enriched in the transition from NMIBC to MIBC (37). Under hypoxic conditions, the transcription of GSDMC was enhanced with PD-L1 mediation, switching apoptosis to tumor pyroptosis and facilitating tumor necrosis (38). KLK6 was identified as a prognostic gene for MIBC (39). Increased expression of SCUBE2, as a luminal marker of urothelial carcinoma, was found to be significantly associated with better disease-free survival (40). The expression of SERPINB2 was proved incrementally expressed in cisplatin-resistant bladder cancer cell lines (41). SLC14A1 was identified to be a urinary bladder cancer susceptibility gene (42). There are no reports on the association between bladder cancer and the ACSM6, B3GAT1, BARX2, BHMT, CASQ1, CCL15, CPA4, EREG, FREM1, HES2, HNF1B, HTR7, IGDCC3, PLIN5, PTPRZ1, SH3RF2, and SLC30A2 gene. Many of these genes have been shown to be functionally expressed in other cancers.
There are several limitations to the study. Although the results obtained using the TCGA database were validated using a GEO dataset, the risk for selection bias could not be avoided. Collecting all clinical information of the bladder cancer cases is impossible, either. The findings from this study are descriptive, and further experiments are needed to confirm the findings and clarify the exact degree of hypoxia in the TME of bladder cancer. Third, we did not compare the different prognostic predicting tools of bladder cancer. Further clinical trials and comparisons between different tools are required to objectively evaluate the prediction power of the hypoxia-related risk model. Despite these limitations, the findings presented here are novel and we demonstrated that the hypoxia-related risk model is effective.
Our findings suggested that the hypoxia score, which reflected the degree of hypoxia in the TME, was related to the prognosis of bladder cancer. Besides, we established a hypoxia-related prognostic risk model based on the 29 genes we identified as hypoxia-related genes. The hypoxia-related model could be applied as a potential biomarker in bladder cancer cases, and the identified genes could be recognized as potential therapeutic targets for bladder cancer. These may assist in the evaluation of bladder cancer prognosis and decision making in clinical practice in the future.
Acknowledgments
Funding: This study accepted the support from Tibetan Natural Science Foundation (grant No. XZ2017ZR-ZY019).
Footnote
Reporting Checklist: The authors have completed the REMARK reporting checklist. Available at https://dx.doi.org/10.21037/tau-21-569
Conflicts of Interest: All authors have completed the ICMJE uniform disclosure form (available at https://dx.doi.org/10.21037/tau-21-569). QZ serves as an unpaid editorial board member of Translational Andrology and Urology from May 2021 to April 2023. The other authors have no conflicts of interest to declare.
Ethical Statement: The authors are accountable for all aspects of the work in ensuring that questions related to the accuracy or integrity of any part of the work are appropriately investigated and resolved. The study was conducted in accordance with the Declaration of Helsinki (as revised in 2013).
Open Access Statement: This is an Open Access article distributed in accordance with the Creative Commons Attribution-NonCommercial-NoDerivs 4.0 International License (CC BY-NC-ND 4.0), which permits the non-commercial replication and distribution of the article with the strict proviso that no changes or edits are made and the original work is properly cited (including links to both the formal publication through the relevant DOI and the license). See: https://creativecommons.org/licenses/by-nc-nd/4.0/.
References
- Bray F, Ferlay J, Soerjomataram I, et al. Global cancer statistics 2018: GLOBOCAN estimates of incidence and mortality worldwide for 36 cancers in 185 countries. CA Cancer J Clin 2018;68:394-424. [Crossref] [PubMed]
- Murphy WM, Soloway MS, Jukkola AF, et al. Urinary cytology and bladder cancer. The cellular features of transitional cell neoplasms. Cancer 1984;53:1555-65. [Crossref] [PubMed]
- Wołącewicz M, Hrynkiewicz R, Grywalska E, et al. Immunotherapy in Bladder Cancer: Current Methods and Future Perspectives. Cancers (Basel) 2020;12:1181. [Crossref] [PubMed]
- McAllister SS, Weinberg RA. The tumour-induced systemic environment as a critical regulator of cancer progression and metastasis. Nat Cell Biol 2014;16:717-27. [Crossref] [PubMed]
- LaGory EL, Giaccia AJ. The ever-expanding role of HIF in tumour and stromal biology. Nat Cell Biol 2016;18:356-65. [Crossref] [PubMed]
- Roudnicky F, Dieterich LC, Poyet C, et al. High expression of insulin receptor on tumour-associated blood vessels in invasive bladder cancer predicts poor overall and progression-free survival. J Pathol 2017;242:193-205. [Crossref] [PubMed]
- Mao X. Hypoxia-Induced Autophagy Enhances Cisplatin Resistance in Human Bladder Cancer Cells by Targeting Hypoxia-Inducible Factor-1α. J Immunol Res 2021;2021:8887437. [Crossref] [PubMed]
- Lodhi T, Song YP, West C, et al. Hypoxia and its Modification in Bladder Cancer: Current and Future Perspectives. Clin Oncol (R Coll Radiol) 2021;33:376-90. [Crossref] [PubMed]
- Eustace A, Irlam JJ, Taylor J, et al. Necrosis predicts benefit from hypoxia-modifying therapy in patients with high risk bladder cancer enrolled in a phase III randomised trial. Radiother Oncol 2013;108:40-7. [Crossref] [PubMed]
- Irlam-Jones JJ, Eustace A, Denley H, et al. Expression of miR-210 in relation to other measures of hypoxia and prediction of benefit from hypoxia modification in patients with bladder cancer. Br J Cancer 2016;115:571-8. [Crossref] [PubMed]
- Hunter BA, Eustace A, Irlam JJ, et al. Expression of hypoxia-inducible factor-1α predicts benefit from hypoxia modification in invasive bladder cancer. Br J Cancer 2014;111:437-43. [Crossref] [PubMed]
- Henning GM, Barashi NS, Smith ZL. Advances in Biomarkers for Detection, Surveillance, and Prognosis of Bladder Cancer. Clin Genitourin Cancer 2021;19:194-8. [Crossref] [PubMed]
- Dao Trong P, Rösch S, Mairbäurl H, et al. Identification of a Prognostic Hypoxia-Associated Gene Set in IDH-Mutant Glioma. Int J Mol Sci 2018;19:2903. [Crossref] [PubMed]
- Xiao K, Tan J, Yuan J, et al. Prognostic value and immune cell infiltration of hypoxic phenotype-related gene signatures in glioblastoma microenvironment. J Cell Mol Med 2020;24:13235-47. [Crossref] [PubMed]
- Sun X, Zhou Z, Zhang Y, et al. Identification and validation of a hypoxia-related prognostic and immune microenvironment signature in bladder cancer. Cancer Cell Int 2021;21:251. [Crossref] [PubMed]
- Jiang M, Ren L, Chen Y, et al. Identification of a Hypoxia-Related Signature for Predicting Prognosis and the Immune Microenvironment in Bladder Cancer. Front Mol Biosci 2021;8:613359. [Crossref] [PubMed]
- Eustace A, Mani N, Span PN, et al. A 26-gene hypoxia signature predicts benefit from hypoxia-modifying therapy in laryngeal cancer but not bladder cancer. Clin Cancer Res 2013;19:4879-88. [Crossref] [PubMed]
- Hänzelmann S, Castelo R, Guinney J. GSVA: gene set variation analysis for microarray and RNA-seq data. BMC Bioinformatics 2013;14:7. [Crossref] [PubMed]
- Langfelder P, Horvath S. WGCNA: an R package for weighted correlation network analysis. BMC Bioinformatics 2008;9:559. [Crossref] [PubMed]
- Szklarczyk D, Franceschini A, Wyder S, et al. STRING v10: protein-protein interaction networks, integrated over the tree of life. Nucleic Acids Res 2015;43:D447-52. [Crossref] [PubMed]
- Shannon P, Markiel A, Ozier O, et al. Cytoscape: a software environment for integrated models of biomolecular interaction networks. Genome Res 2003;13:2498-504. [Crossref] [PubMed]
- Yu G, Wang LG, Han Y, et al. clusterProfiler: an R package for comparing biological themes among gene clusters. OMICS 2012;16:284-7. [Crossref] [PubMed]
- Infantino V, Santarsiero A, Convertini P, et al. Cancer Cell Metabolism in Hypoxia: Role of HIF-1 as Key Regulator and Therapeutic Target. Int J Mol Sci 2021;22:5703. [Crossref] [PubMed]
- Hanna SC, Krishnan B, Bailey ST, et al. HIF1α and HIF2α independently activate SRC to promote melanoma metastases. J Clin Invest 2013;123:2078-93. [Crossref] [PubMed]
- Sun H, Zhang D, Huang C, et al. Hypoxic microenvironment induced spatial transcriptome changes in pancreatic cancer. Cancer Biol Med 2021;18:616-30. [Crossref] [PubMed]
- Shi Q, Chen Q, Zhou Z, et al. Hypoxia-induced antizyme inhibitors 2 regulates cisplatin resistance through epithelia-mesenchymal transition pathway in non-small cell lung cancer. Pulm Pharmacol Ther 2021;69:102048. [Crossref] [PubMed]
- Liu X, Ye N, Liu S, et al. Hyperbaric Oxygen Boosts PD-1 Antibody Delivery and T Cell Infiltration for Augmented Immune Responses Against Solid Tumors. Adv Sci (Weinh) 2021;8:e2100233. [Crossref] [PubMed]
- Augustin RC, Delgoffe GM, Najjar YG. Characteristics of the Tumor Microenvironment That Influence Immune Cell Functions: Hypoxia, Oxidative Stress, Metabolic Alterations. Cancers (Basel) 2020;12:3802. [Crossref] [PubMed]
- Eckstein M, Wirtz RM, Gross-Weege M, et al. mRNA-Expression of KRT5 and KRT20 Defines Distinct Prognostic Subgroups of Muscle-Invasive Urothelial Bladder Cancer Correlating with Histological Variants. Int J Mol Sci 2018;19:3396. [Crossref] [PubMed]
- Luo Y, Tian Z, Hua X, et al. Isorhapontigenin (ISO) inhibits stem cell-like properties and invasion of bladder cancer cell by attenuating CD44 expression. Cell Mol Life Sci 2020;77:351-63. [Crossref] [PubMed]
- Ding G, Yu S, Cheng S, et al. Androgen receptor (AR) promotes male bladder cancer cell proliferation and migration via regulating CD24 and VEGF. Am J Transl Res 2016;8:578-87. [PubMed]
- Koguchi D, Matsumoto K, Shimizu Y, et al. Prognostic Impact of AHNAK2 Expression in Patients Treated with Radical Cystectomy. Cancers (Basel) 2021;13:1748. [Crossref] [PubMed]
- Kitchen MO, Bryan RT, Emes RD, et al. Quantitative genome-wide methylation analysis of high-grade non-muscle invasive bladder cancer. Epigenetics 2016;11:237-46. [Crossref] [PubMed]
- Bernardo C, Eriksson P, Marzouka NA, et al. Molecular pathology of the luminal class of urothelial tumors. J Pathol 2019;249:308-18. [Crossref] [PubMed]
- Sasaki T, Horikawa M, Orikasa K, et al. Possible relationship between the risk of Japanese bladder cancer cases and the CYP4B1 genotype. Jpn J Clin Oncol 2008;38:634-40. [Crossref] [PubMed]
- Carrasco R, Izquierdo L, van der Heijden AG, et al. Differential gene expression profile between progressive and de novo muscle invasive bladder cancer and its prognostic implication. Sci Rep 2021;11:6132. [Crossref] [PubMed]
- Gatta LB, Melocchi L, Bugatti M, et al. Hyper-Activation of STAT3 Sustains Progression of Non-Papillary Basal-Type Bladder Cancer via FOSL1 Regulome. Cancers (Basel) 2019;11:1219. [Crossref] [PubMed]
- Hou J, Zhao R, Xia W, et al. PD-L1-mediated gasdermin C expression switches apoptosis to pyroptosis in cancer cells and facilitates tumour necrosis. Nat Cell Biol 2020;22:1264-75. [Crossref] [PubMed]
- Zhang PB, Huang ZL, Xu YH, et al. Systematic analysis of gene expression profiles reveals prognostic stratification and underlying mechanisms for muscle-invasive bladder cancer. Cancer Cell Int 2019;19:337. [Crossref] [PubMed]
- Ottley EC, Pell R, Brazier B, et al. Greater utility of molecular subtype rather than epithelial-to-mesenchymal transition (EMT) markers for prognosis in high-risk non-muscle-invasive (HGT1) bladder cancer. J Pathol Clin Res 2020;6:238-51. [Crossref] [PubMed]
- Taoka Y, Matsumoto K, Ohashi K, et al. Protein expression profile related to cisplatin resistance in bladder cancer cell lines detected by two-dimensional gel electrophoresis. Biomed Res 2015;36:253-61. [Crossref] [PubMed]
- Rafnar T, Vermeulen SH, Sulem P, et al. European genome-wide association study identifies SLC14A1 as a new urinary bladder cancer susceptibility gene. Hum Mol Genet 2011;20:4268-81. [Crossref] [PubMed]