Diagnostic and prognostic role of basic leucine zipper transcription factor in kidney renal clear cell carcinoma
Introduction
Kidney cancer, also known as renal cell carcinoma (RCC) has a high incidence, with more than 400,000 new cases reported worldwide in 2018 alone, and approximately 30% of patients presenting with advanced disease (1). Kidney renal clear cell carcinoma (KIRC) is the major histological subtype of RCC, accounting for ~80% of all RCC cases (2). Effective treatment of metastatic RCC remains a major medical concern, because these tumors are refractory to standard therapies and their prognosis is poor (3). Therefore, identification of novel effective biomarkers for prognosis of KIRC is important to the management of this disease. In recent years, new immunotherapies and targeted medicine have become the focus for management of metastatic KIRC. For example, mTORC1 inhibitors have been approved for treatment of metastatic kidney cancer (4). Moreover, metastatic RCC is now commonly treated using vascular endothelial growth factor inhibitors (5), and immune checkpoint inhibitors targeting the PD-1 signaling pathway have become the first- or second-line of treatment for metastatic urothelial carcinoma and metastatic RCC (6).
Basic leucine zipper transcription factor (BATF), a member of the highly conserved activator protein-1 (AP1) superfamily (7), which includes the Fos, Jun, and Atf proteins (8), has been extensively studied. Notably, the BATF activating transcription factor-like (Batf) plays a key role in transcriptional programming of multiple effector T cells, and also regulates growth of the T-helper 17 (Th17) and T-follicular helper (Tfh) cells (9). Previous studies have shown that the BATF gene regulates the function of T cells; its upregulation has been associated with T cell exhaustion (10). Additional evidence has demonstrated that BATF not only influences the development of different types of cancers, but also correlates with PDCD1 expression, and suppressing the function of CD8+ T cells in colorectal cancer (11). Apart from these roles, BATF has been associated with cell proliferation and apoptosis in non-small cell lung cancer (NSCLC) A549 cells (12). Previous studies have also demonstrated BATF’s importance as a member of the regulatory network in anaplastic large cell lymphoma, as evidenced by its pathogenic and therapeutic indications (13). Augmented expression of BATF reportedly mediated inhibition of AP-1 activity, reduced proliferation, and downregulated expression of cyclin D in the human multiple myeloma cell line RPMI8226 (14). Moreover, BATF overexpression was associated with increased migration and invasiveness of the human breast cancer cell lines, T47D and MCF-7 (15). Based on all this evidence, we hypothesized that BATF could be a potential biomarker for both diagnosis and prognosis of KIRC patients.
The underlying mechanism of action of BATF in immune cell infiltration remains unclear. Considering BATF’s important role in the occurrence and development of several types of carcinomas, we hypothesized that BATF may be upregulated in KIRC patients. Prior to this study, we analyzed patterns of BATF expression in pan-cancers, and KIRC cases. Generally, the development and progression of malignant tumors requires interaction with other cells in the tumor microenvironment, especially immune cells (16). Immune infiltration of tumors is closely associated with clinical outcome in renal cell carcinoma (RCC). The importance of immune infiltration for prognosis has been recognized. Many kinds of immune cells were found with important roles in the prognosis of KIRC. Therefore, we investigated levels of immune cell infiltration of BATF in KIRC by investigating the levels of BATF mRNA expression using data from The Cancer Genome Atlas (TCGA) database. We present the following article in accordance with the REMARK reporting checklist (available at https://tau.amegroups.com/article/view/10.21037/tau-21-1130/rc).
Methods
Datasets
The transcriptome dataset used in this study was downloaded from the TCGA website (https://tcga-data.nci.nih.gov/tcga/). The dataset comprised all 33 enrolled cancer types involved in the pan-cancer analysis, including KIRC. We downloaded profiles of BATF transcriptional expression, which included 539 KIRC cases alongside 72 cases of matched adjacent tissues. In addition, we downloaded the corresponding clinical information for these patients, including TNM stage, pathologic stage, histologic grade, primary therapy outcome, age, sex, race, and laterality, as well as overall survival (OS), progression-free interval (PFI), and disease-specific survival (DSS). We also downloaded RNA-seq data [in transcripts per million (TPM) format] from the TCGA and Genotype-Tissue Expression (GTEx) databases that are uniformly processed by Toil from UCSC Xena (https://xenabrowser.net/datapages/) (17). The median RNA expression value was regarded as the cutoff, and used to stratify patients into either the high or low expression group, while the area under the curve (AUC) of the receiver-operating characteristic (ROC) curves was used as qualitative and quantitative performance indicator. To investigate BATF’s prognostic value in KIRC, we generated survival curves using Kaplan-Meier (K-M) for OS. Next, we used univariate and multivariate Cox regression analyses to determine the dependency of every clinical factor and the relationship between BATF and OS, then established a nomogram model based on the results to predict the prognosis of KIRC patients. Using these identified prognostic factors (P<0.05 that significantly affected survival rates in KIRC patients), we constructed a nomogram for predicting 1-, 3- and 5-year survival rates in KIRC patients. We used the concordance index (C-index) to evaluate the discriminative ability of the nomogram. A C-index or AUC of 0.5 indicates a discrimination ability that is no better than chance, whereas that of 1.0 indicates a perfect discrimination ability.
The downloaded data, which was in level 3 HTSeq-fragments per kilobase per million (FPKM) format, was converted into TPM format prior to subsequent analysis. The study was conducted in accordance with the Declaration of Helsinki (as revised in 2013). Because all the data were downloaded from a public database (TCGA), we did not need ethical approval.
Construction of protein-protein interaction (PPI) networks and functional enrichment analysis
A PPI network of differentially expressed genes was constructed using the Search Tool for the Retrieval of Interacting Genes (STRING) database [https://string-db.org/ (version 11.5)] (18,19). Notably, both physical interactions and functional associations were involved, with a minimum interaction score of 0.4 (default setting). The interactions from STRING, which comprise both direct (physical) and indirect (functional) associations, are collected from genomic contexts, co-expression, high-throughput experiments, and reports across the literature. Next, we performed gene ontology (GO) enrichment analysis and Kyoto Encyclopedia of Genes and Genomes (KEGG) pathway analysis of co-expression genes. Enrichment analyses targeted ontologies/pathway gene sets, including biological processes (BP), cellular components, molecular function (MF), and KEGG pathways. Moreover, we applied the Spearman correlation coefficient to determine the relationship between BATF and co-expressed molecular mechanisms in KIRC.
Determination of BATF’s role in immune cell infiltration
The Tumor Immune Estimation Resource (TIMER) is an online database used for systematic analysis of immune cell infiltrates across many cancers (20,21). In the present study, we applied the algorithm implemented in TIMER Version 2.0 to estimate the infiltration of six important immune cells, namely B cells, CD4+ T cells, CD8+ T cells, neutrophils, macrophages, and dendritic cells, followed by correlation with BATF expression in KIRC.
Determination of the relationship between BATF expression and tumor infiltrating immune cells (TIICs)
The Tumor-Immune System Interaction Database (TISIDB), an online web portal for analyzing the interaction between tumors and the immune system, integrates multiple heterogeneous datasets (http://cis.hku.hk/TISIDB/index.php) (22). In this study, we used the TISIDB database to generate a heatmap and depict the interaction between BATF expression and TIICs across human cancers. Next, we used the Gene Set Variation Analysis (GSVA) database, based on gene expression profiles, to infer the relative abundance of TILs. The immune-related signatures of 28 TIICs types from Charoentong et al.’s study (23) from TISIDB revealed gene expression profiles, GSVA depicted the relative abundance of TIICs, and Spearman’s correlation test was used to determine the relationship between BATF expression and TIICs.
Statistical analysis
All statistical analyses were performed using packages implemented in R software (version 3.6.3). Differences in expression were visualized using the ggplot2 package (version 3.3.3) in R. The Mann-Whitney U-test (Wilcoxon signed rank test) and paired t-test were used to determine differences in the pan-cancer group, as well as between KIRC and paired normal kidney tissues. The pROC package (version 1.17.0.1) in R was used to generate ROC curves and depict BATF’s diagnostic value. In addition, the survminer package (version 0.4.9) was used to generate K-M curves, and also perform univariate and multivariate Cox regression analyses, as well as log-rank tests; the survival package (version 3.2-10) was used to assess the effect of BATF on survival (24). Moreover, to determine the relationship between BATF expression and corresponding clinical information, the data were first tested for normal distribution using the Shapiro-Wilk normality test (α=0.05, P>0.05), recommended for analysis of variance (ANOVA). Statistical analyses were performed using t-tests with a Welch correction for unequal variances or an ANOVA followed by a post-hoc analysis; P values were adjusted using either a Bonferroni correction when variances were not significantly different or a Games-Howell correction if variances were significantly different. Next, we used the Bioconductor/R rms (version 6.2-0) package to construct nomogram models based on logistic regression modeling. Gene identifiers and GO annotations in both datasets were performed using the “ClusterProfiler” package (v3.14.3) and visualized using the “ggplot2” package. Conversion of these datasets from Entrez IDs to gene symbols was achieved by annotating them using the org.Hs.eg.db package (v3.10.0) (25). All statistical analyses were two-sided, and data followed by P<0.05 were considered statistically significant.
Results
Profiles of BATF expression in the pan-cancer dataset
Results from unpaired analysis revealed a total of 18,102 cases across 33 different cancers types. Two cancer types, namely mesothelioma (MESO) and uveal melanoma (UVM), were excluded due to lack of data from control subjects, and SARC was excluded because it had less than three cases in the normal group. Finally, 17,672 samples across 30 cancer types were included in our analysis. Results from the unpaired analysis, showing significant upregulation of BATF in 27 of 30 different cancers relative to normal tissues, are displayed in Figure 1A. For the paired analysis, a total of 1,340 cases across 18 different cancer types were involved. BATF was also significantly upregulated in 11 of 18 cancer types, relative to normal tissues (Figure 1B). Overall, these results indicated that BATF mRNA was significantly upregulated across different cancer types relative to normal adjacent tissues.
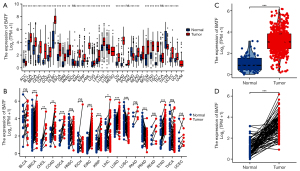
Patterns of BATF expression in KIRC patients
Baseline characteristics of the KIRC patients from TCGA are presented in Table 1. Results from unpaired analyses showed that BATF mRNA was significantly upregulated in KIRC (n=539) relative to adjacent normal kidney tissues (n=72) (Figure 1C, Mann-Whitney U-test, P<0.001). Similarly, results from the paired analysis revealed significant BATF expression in KIRC (n=72) relative to adjacent normal kidney tissues (n=72) (3.216±1.072 vs. 1.034±0.729, respectively, P<0.001) (Figure 1D). Collectively, these results indicated that BATF mRNA was significantly upregulated in KIRC relative to normal adjacent tissues.
Table 1
Characteristic | Level | Low BATF expression | High BATF expression | P value |
---|---|---|---|---|
n | 269 | 270 | ||
T stage, n (%) | T1 | 165 (30.6) | 113 (21.0) | <0.001 |
T2 | 30 (5.6) | 41 (7.6) | ||
T3 | 71 (13.2) | 108 (20.0) | ||
T4 | 3 (0.6) | 8 (1.5) | ||
N stage, n (%) | N0 | 117 (45.5) | 124 (48.2) | 0.279 |
N1 | 5 (1.9) | 11 (4.3) | ||
M stage, n (%) | M0 | 227 (44.9) | 201 (39.7) | 0.001 |
M1 | 25 (4.9) | 53 (10.5) | ||
Pathologic stage, n (%) | Stage I | 165 (30.8) | 107 (20.0) | <0.001 |
Stage II | 25 (4.7) | 34 (6.3) | ||
Stage III | 51 (9.5) | 72 (13.4) | ||
Stage IV | 27 (5.0) | 55 (10.3) | ||
Primary therapy outcome, n (%) | PD | 6 (4.1) | 5 (3.4) | 0.051 |
SD | 6 (4.1) | 0 (0) | ||
PR | 2 (1.4) | 0 (0) | ||
CR | 66 (44.9) | 62 (42.2) | ||
Sex, n (%) | Female | 102 (18.9) | 84 (15.6) | 0.116 |
Male | 167 (31.0) | 186 (34.5) | ||
Race, n (%) | Asian | 3 (0.6) | 5 (0.9) | 0.589 |
Black or African American | 31 (5.8) | 26 (4.9) | ||
White | 230 (43.2) | 237 (44.5) | ||
Age, n (%) | ≤60 | 131 (24.3) | 138 (25.6) | 0.636 |
>60 | 138 (25.6) | 132 (24.5) | ||
Histologic grade, n (%) | G1 | 10 (1.9) | 4 (0.8) | <0.001 |
G2 | 137 (25.8) | 98 (18.5) | ||
G3 | 96 (18.1) | 111 (20.9) | ||
G4 | 19 (3.6) | 56 (10.5) | ||
Laterality, n (%) | Left | 114 (21.2) | 138 (25.7) | 0.047 |
Right | 155 (28.8) | 131 (24.3) | ||
OS event, n (%) | Alive | 198 (36.7) | 168 (31.2) | 0.006 |
Dead | 71 (13.2) | 102 (18.9) | ||
PFI event, n (%) | Alive | 203 (37.7) | 175 (32.5) | 0.009 |
Dead | 66 (12.2) | 95 (17.6) | ||
DSS event, n (%) | Alive | 225 (42.6) | 195 (36.9) | <0.001 |
Dead | 37 (7.0) | 71 (13.4) | ||
Age, median [IQR] | 61 [52, 72] | 60 [52, 69] | 0.326 |
KIRC, kidney renal clear cell carcinoma; BATF, basic leucine zipper transcription factor; PD, progressive disease; SD, stable disease; CR, complete response; PR, partial response; OS, overall survival; PFI, progression-free interval; DSS, disease-specific survival; IQR, interquartile range.
Clinical significance of BATF in KIRC patients
Next, we analyzed the relationship between patterns of KIRC mRNA expression with clinicopathological characteristics of 539 KIRC samples with the aim of ascertaining BATF’s clinical significance in KIRC. A summary of the demographic, clinical, and pathological characteristics of all 539 KIRC patients is shown in Table 1. As shown in Table 1 and Figure 2A-2I, high BATF expression in patients was significantly associated with distant metastasis (P=0.001), higher T stage (P<0.001), higher pathologic stage (P<0.001), higher histologic grade (P<0.001), as well as poor OS (P=0.006), PFI (P=0.009), and DSS (P<0.001).
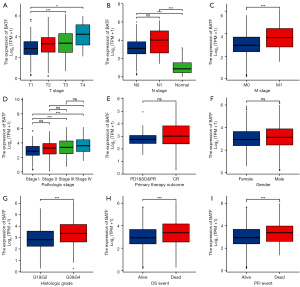
Conversely, we found no statistically significant correlation between BATF expression and other clinicopathological characteristics, including lymph node metastasis (N stage, P=0.279), sex (P=0.116), race (P=0.589), and age (P=0.636). Overall, these results showed that BATF was associated with higher TNM stage, higher pathological grade/stage, and poor prognosis for KIRC.
Diagnosis of BATF in KIRC
ROC curves showed that BATF had an AUC value of 0.942 [95% confidence interval (CI): 0.917–0.968], with a cutoff value of 2.033, while accuracy, sensitivity, and specificity of adjacent controls were 87.2%, 91.7%, and 86.6%, respectively (Figure 3A). The positive and negative predictive values were 47.8% and 98.7%, respectively. Overall, these results suggested that BATF could be a good diagnostic indicator in KIRC.
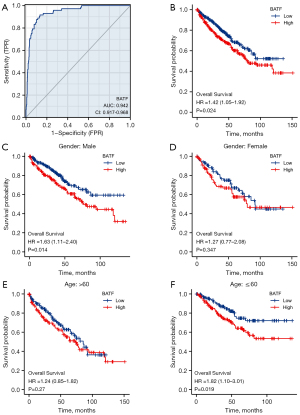
Association of BATF expression with shorter OS
Next, we generated K-M curves to evaluate the relationship between BATF expression and OS, and found that high BATF expression was associated with significantly shorter OS (log-rank test P=0.024, Figure 3B).
OS results from subgroup analysis
We categorized patients into subgroups according to sex (male vs. female) and age [younger (≤60 years) vs. older (>60 years)], then applied log-rank test and Cox regression analyses. Results from subgroup analyses according to sex revealed significant differences between the high and low BATF expression groups in male patients [hazard ratio (HR) =1.63, 95% CI: 1.11–2.40, P=0.014, Figure 3C], whereas no statistically significant differences were found between the groups of female patients (P=0.34, Figure 3D). Based on age, the K-M curves showed that high BATF expression in older (>60 years) patients was not significantly associated with worse OS than in the low BATF group (P=0.27) (Figure 3E), whereas statistically significant differences were observed in younger (≤60 years) patients (HR =1.82, 95% CI: 1.10–3.01, P=0.019, Figure 3F). Overall, these data suggested that BATF upregulation may be a predictor of poor prognosis in KIRC.
Cox regression and nomogram model
Results from univariate Cox analysis revealed that T stage, N stage, M stage, age, and BATF expression were all significantly associated with OS and DFS (Table 2). In contrast, sex was not significantly associated with either survival rate. Statistically significant risk factors from univariate analysis were used in the multivariate Cox analysis, with results indicating that age, TNM stage and BATF were significant independent risk factors for OS (Table 2). Based on these results, we constructed a nomogram model for predicting survival rates at 1, 3, and 5 years (Figure 4), and it had excellent predictive power, as evidenced by a C-index of 0.769 (95% CI: 0.745–0.793).
Table 2
Characteristic | Total (N) | Univariate analysis | Multivariate analysis | |||
---|---|---|---|---|---|---|
Hazard ratio (95% CI) | P value | Hazard ratio (95% CI) | P value | |||
T stage | 539 | |||||
T1 | 278 | Reference | ||||
T2 | 71 | 1.515 (0.908–2.526) | 0.112 | 0.824 (0.396–1.715) | 0.605 | |
T3 | 179 | 3.354 (2.373–4.742) | <0.001 | 2.092 (1.233–3.550) | 0.006 | |
T4 | 11 | 10.829 (5.467–21.451) | <0.001 | 1.989 (0.534–7.414) | 0.306 | |
N stage | 257 | |||||
N0 | 241 | Reference | ||||
N1 | 16 | 3.453 (1.832–6.508) | <0.001 | 2.060 (0.819–5.177) | 0.124 | |
M stage | 506 | |||||
M0 | 428 | Reference | ||||
M1 | 78 | 4.389 (3.212–5.999) | <0.001 | 3.128 (1.866–5.244) | <0.001 | |
Sex | 539 | |||||
Female | 186 | Reference | ||||
Male | 353 | 0.930 (0.682–1.268) | 0.648 | |||
Age | 539 | |||||
≤60 | 269 | Reference | ||||
>60 | 270 | 1.765 (1.298–2.398) | <0.001 | 1.800 (1.160–2.793) | 0.009 | |
BATF | 539 | |||||
Low | 270 | Reference | ||||
High | 269 | 1.445 (1.067–1.957) | 0.017 | 1.073 (0.693–1.663) | 0.751 |
BATF, basic leucine zipper transcription factor.
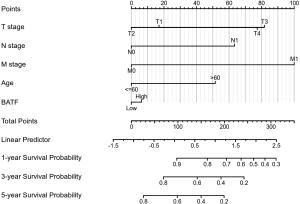
PPI networks and functional annotations
An interactive map between BATF and its 10 co-expression genes was generated using the STRING database, and the corresponding PPI network was constructed (Figure 5A). As shown in Figure 5B, enrichment analyses revealed that changes in the BPs of BATF were associated with differentiation of T-helper cells, CD4+, and alpha-beta T-cells involved in the immune response, and alpha-beta T-cell activation involved in the immune response. KEGG pathway analysis results indicated that these related 10 genes as shown in PPI network were involved in Th17 cell differentiation, inflammatory bowel disease, as well as regulation of PD-L1 expression and PD-1 checkpoint pathways in cancer.
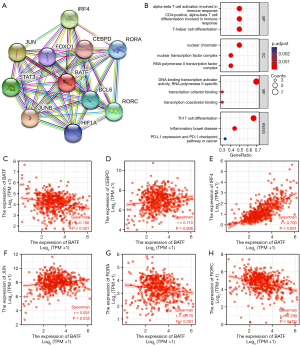
Correlating the profiles of BATF expression with co-expressed MFs in KIRC revealed no statistically significant differences in BCL6 (P=0.322), JUNB (P=0.084) or STAT3 (P=0.257) (Figure 5C-5H). However, Spearman correlation revealed a significant negative relationship between BATF expression and FOXO1 (r=−0.190, P<0.001), HIF1A (r=−0.089, P=0.039), RORA (r=−0.170, P<0.001), and RORC (r=−0.290, P<0.001), but a positive correlation with CEBPD (r=0.110, P=0.008), IRF4 (R=0.700, P<0.001), and JUN (r=0.091, P=0.035).
Relationship between BATF expression and immune cell infiltration in KIRC
The relationship between BATF expression with immune cell infiltration levels in KIRC patients is depicted using 7 scatterplots in Figure 6A,6B. Purity-corrected partial Spearman’s rho value and statistical significance are shown. Levels of BATF expression against tumor purity are displayed in the left-most panel: tumor purity (r=−0.345, P=2.3e-15), B cell (r=0.392, P=2.86e-18), CD8+ T cell (r=0.514, P=5.92e-31), CD4+ T cell (r=0.28, P=9.87e-10), macrophages (r=0.215, P=4.40e-06), neutrophils (r=0.418, P=7.79e-21), and dendritic cells (r=0.569, P=1.98e-40). Results of the correlation between BATF expression and the 28 types of TIICs across different cancers are depicted in a heatmap (Figure 6C). Moreover, BATF expression was closely correlated with abundance of CD56dim cells (r=0.448, P<2.2e-16), CD8+ T cells (r=0.322, P=3.1e-14), Tcm CD4+ T cells (r=0.588, P<2.2e-16), monocytes (r=0.369, P<2.2e-16), myeloid-derived suppressor cells (r=0.776, P<2.2e-16), and Treg cells (r=0.448, P<2.2e-16) (Figure 6D-6I). Collectively, these results indicated that BATF plays an important role in immune cell infiltration in KIRC.
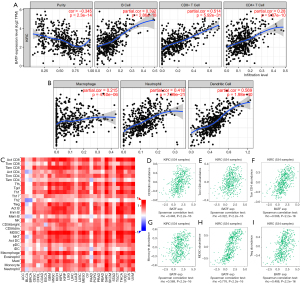
Discussion
Results of the present study showed that BATF is significantly upregulated in KIRC and pan-cancer tissues, relative to adjacent normal tissues. This upregulation is significantly associated with the clinicopathological characteristics of KIRC patients, including higher TNM stage, higher pathologic stage and higher histologic grade. In addition, ROC curves suggested that BATF could be a diagnostic indicator for KIRC patients, and the K-M curves and results from univariate analysis indicated that upregulation of BATF mRNA correlates with poor prognosis of KIRC patients. Our results further indicated that BATF plays an important role in immune cell infiltration in KIRC.
BATF is a transcription factor that plays an important role in regulating differentiation and function in many lymphocyte lineages (8). Previous studies have shown that BATF plays an oncogenic role in many cancers, such as lung cancer (12), lymphoma (13), myeloma (14), colon cancer (26), breast cancer (15), and KIRC (27). Additional evidence has also associated BATF upregulation with poor prognosis of patients with various cancers (27-29). In the present study our results revealed that BATF mRNA was significantly upregulated in various carcinomas, including KIRC, which was consistent with findings from a previous study that showed BATF associated with higher clinical TNM stage (28). Although previous reports suggested that BATF could be an important prognostic sign of poor clinical outcomes in patients with several kinds of cancer (27,28), this role in KIRC had remained unclear.
BATF was first reported by Schraml et al. in 2009, as an AP-1 transcription factor that regulated Th17 differentiation (30), and in the following year Betz et al. found it coordinated multiple aspects of B and T cell functions (8). Recently, Feng et al. performed in vitro experiments that revealed BATF acting as an oncogene in NSCLC (12). Overall, these findings suggest that BATF is a potential biomarker for cancer treatment. Our results revealed that BATF is significantly upregulated in KIRC relative to normal kidney tissues, suggesting that this gene could be used in the diagnosis of KIRC. To validate this finding and ascertain its clinical value, we generated ROC curves and found that BATF could effectively predict the prognosis of KIRC patients, as evidenced by sensitivity, specificity, and accuracy values of 91.7%, 86.6% and 87.2%, respectively, suggesting that BATF could be a potential diagnostic signature for KIRC.
Several recent studies have revealed that BATF is significantly correlated to poor OS of patients with many kinds of cancers. For instance, several DNA methylation-driven genes, including BATF, were found to have excellent prognostic value in colorectal carcinoma and clear cell RCC (27,28), and BATF was also found to have a potential role in predicting OS rates of patients with endometrial cancer (31). Results from logistic regression analysis in all 539 patients in the present study revealed that high BATF expression was significantly associated with distant metastasis, higher T stage, higher pathologic stage, and higher histologic grade, indicating that this gene plays a crucial role in KIRC development. In addition, K-M curves, and log-rank test results indicated that high BATF expression was significantly associated with a shorter OS rate of patients. Interestingly, results from subgroup OS analysis indicated that BATF expression was significantly associated with poor OS rates of male patients and those younger than 60 years, but not significantly correlated with female patients and those older than 60 years. These results indicated that BATF is a reliable biomarker for poor prognosis of young, male KIRC patients.
Previous studies have shown that BATF plays a key role in the occurrence and development of several cancers. For instance, knocking down BATF inhibited the proliferation and induced apoptosis of A549 cells in NSCLC (12). In myeloma, BATF-mediated reduction in AP-1 activity resulted in decreased proliferation of RPMI8226 cells (14). In the present study, PPI networks revealed that BATF was significantly co-expressed with FOXO1, HIF1A, RORA, RORC, CEBPD, IRF4, and JUN, suggesting that it could also be an important factor in Th17 cell differentiation, inflammatory bowel disease, and PD-L1 expression and PD-1 checkpoint pathway in cancer. However, these findings need to be validated experimentally.
BATF was first reported to regulate multiple aspects of B and T cell functions by Betz et al. (8). Since then, subsequent studies have demonstrated that this gene is significantly upregulated in human TIICs, and is accompanied by a significant effect on their anti-tumor function (32). Previous studies have also shown that BATF is a checkpoint in a transcriptional circuit that ensures proper coordination of effector CD8+ T-cell differentiation (33), although the relationship between BATF expression and immune cell infiltration has not been shown until now. Results of the present study revealed that six tumor-infiltrating immune cells, namely B cells, CD8+ cells, CD4+ T cells, macrophages, neutrophils, and dendritic cells, were significantly associated with BATF expression in KIRC. In addition, we observed a relationship between genotype-immunophenotype and predictors of response to checkpoint blockade (23). Furthermore, BATF expression strongly correlated with abundance of several important TIICs, such as CD56dim cells, CD8+ T cells, Tcm CD4+ T cells, monocytes, myeloid-derived suppressor cells, and Treg cells in KIRC. Taken together, these results indicated that BATF plays a crucial role in immune cell infiltration of KIRC.
This study had several limitations. Firstly, the observational nature of the study implies that it was biased to issues inherent in clinical practice. Secondly, all data were obtained from public online databases, although this proportion still represents most patients with KIRC, this subset is still not generalizable to the entire KIRC population. Lastly, we did not validate BATF’s prognostic significance in KIRC using in vivo and in vitro experiments.
In summary, this is the first study describing the relationship between BATF upregulation and its association with clinicopathological parameters in KIRC. Our results indicated that BATF is an important indicator for diagnosis and poor prognosis of KIRC patients. Furthermore, our results indicated that BATF expression correlates with immune cell infiltration in KIRC patients, and could be playing an important role in suppressing tumor progression. It may help providing a new perspective and help for the diagnosis, prognosis and treatment choice of KIRC patients.
Acknowledgments
Funding: None.
Footnote
Reporting Checklist: The authors have completed the REMARK reporting checklist Available at https://tau.amegroups.com/article/view/10.21037/tau-21-1130/rc
Conflicts of Interest: All authors have completed the ICMJE uniform disclosure form (available at https://tau.amegroups.com/article/view/10.21037/tau-21-1130/coif). The authors have no conflicts of interest to declare.
Ethical Statement: The authors are accountable for all aspects of the work in ensuring that questions related to the accuracy or integrity of any part of the work are appropriately investigated and resolved. The study was conducted in accordance with the Declaration of Helsinki (as revised in 2013).
Open Access Statement: This is an Open Access article distributed in accordance with the Creative Commons Attribution-NonCommercial-NoDerivs 4.0 International License (CC BY-NC-ND 4.0), which permits the non-commercial replication and distribution of the article with the strict proviso that no changes or edits are made and the original work is properly cited (including links to both the formal publication through the relevant DOI and the license). See: https://creativecommons.org/licenses/by-nc-nd/4.0/.
References
- Cella D, Grünwald V, Nathan P, et al. Quality of life in patients with advanced renal cell carcinoma given nivolumab versus everolimus in CheckMate 025: a randomised, open-label, phase 3 trial. Lancet Oncol 2016;17:994-1003. [Crossref] [PubMed]
- Scelo G, Purdue MP, Brown KM, et al. Genome-wide association study identifies multiple risk loci for renal cell carcinoma. Nat Commun 2017;8:15724. [Crossref] [PubMed]
- Petrella BL, Vincenti MP. Interleukin-1β mediates metalloproteinase-dependent renal cell carcinoma tumor cell invasion through the activation of CCAAT enhancer binding protein β. Cancer Med 2012;1:17-27. [Crossref] [PubMed]
- Voss MH, Hsieh JJ. Therapeutic Guide for mTOuRing through the Braided Kidney Cancer Genomic River. Clin Cancer Res 2016;22:2320-2. [Crossref] [PubMed]
- Hsieh JJ, Chen D, Wang PI, et al. Genomic Biomarkers of a Randomized Trial Comparing First-line Everolimus and Sunitinib in Patients with Metastatic Renal Cell Carcinoma. Eur Urol 2017;71:405-14. [Crossref] [PubMed]
- Zhu J, Armstrong AJ, Friedlander TW, et al. Biomarkers of immunotherapy in urothelial and renal cell carcinoma: PD-L1, tumor mutational burden, and beyond. J Immunother Cancer 2018;6:4. [Crossref] [PubMed]
- Liao J, Humphrey SE, Poston S, et al. Batf promotes growth arrest and terminal differentiation of mouse myeloid leukemia cells. Mol Cancer Res 2011;9:350-63. [Crossref] [PubMed]
- Betz BC, Jordan-Williams KL, Wang C, et al. Batf coordinates multiple aspects of B and T cell function required for normal antibody responses. J Exp Med 2010;207:933-42. [Crossref] [PubMed]
- Pham D, Moseley CE, Gao M, et al. Batf Pioneers the Reorganization of Chromatin in Developing Effector T Cells via Ets1-Dependent Recruitment of Ctcf. Cell Rep 2019;29:1203-1220.e7. [Crossref] [PubMed]
- Quigley M, Pereyra F, Nilsson B, et al. Transcriptional analysis of HIV-specific CD8+ T cells shows that PD-1 inhibits T cell function by upregulating BATF. Nat Med 2010;16:1147-51. [Crossref] [PubMed]
- Yang R, Cheng S, Luo N, et al. Distinct epigenetic features of tumor-reactive CD8+ T cells in colorectal cancer patients revealed by genome-wide DNA methylation analysis. Genome Biol 2019;21:2. [Crossref] [PubMed]
- Feng Y, Pan L, Zhang B, et al. BATF acts as an oncogene in non-small cell lung cancer. Oncol Lett 2020;19:205-10. [PubMed]
- Schleussner N, Merkel O, Costanza M, et al. The AP-1-BATF and -BATF3 module is essential for growth, survival and TH17/ILC3 skewing of anaplastic large cell lymphoma. Leukemia 2018;32:1994-2007. [Crossref] [PubMed]
- Gil M, Pak HK, Park SJ, et al. Engagement of CD99 Reduces AP-1 Activity by Inducing BATF in the Human Multiple Myeloma Cell Line RPMI8226. Immune Netw 2015;15:260-7. [Crossref] [PubMed]
- Zhang Z, Lin M, Wang J, et al. Calycosin inhibits breast cancer cell migration and invasion by suppressing EMT via BATF/TGF-β1. Aging (Albany NY) 2021;13:16009-23. [Crossref] [PubMed]
- Acs B, Ahmed FS, Gupta S, et al. An open source automated tumor infiltrating lymphocyte algorithm for prognosis in melanoma. Nat Commun 2019;10:5440. [Crossref] [PubMed]
- Vivian J, Rao AA, Nothaft FA, et al. Toil enables reproducible, open source, big biomedical data analyses. Nat Biotechnol 2017;35:314-6. [Crossref] [PubMed]
- Kathuria A, Lopez-Lengowski K, Vater M, et al. Transcriptome analysis and functional characterization of cerebral organoids in bipolar disorder. Genome Med 2020;12:34. [Crossref] [PubMed]
- Szklarczyk D, Gable AL, Nastou KC, et al. The STRING database in 2021: customizable protein-protein networks, and functional characterization of user-uploaded gene/measurement sets. Nucleic Acids Res 2021;49:D605-12. [Crossref] [PubMed]
- Li T, Fan J, Wang B, et al. TIMER: A Web Server for Comprehensive Analysis of Tumor-Infiltrating Immune Cells. Cancer Res 2017;77:e108-10. [Crossref] [PubMed]
- Li B, Severson E, Pignon JC, et al. Comprehensive analyses of tumor immunity: implications for cancer immunotherapy. Genome Biol 2016;17:174. [Crossref] [PubMed]
- Ru B, Wong CN, Tong Y, et al. TISIDB: an integrated repository portal for tumor-immune system interactions. Bioinformatics 2019;35:4200-2. [Crossref] [PubMed]
- Charoentong P, Finotello F, Angelova M, et al. Pan-cancer Immunogenomic Analyses Reveal Genotype-Immunophenotype Relationships and Predictors of Response to Checkpoint Blockade. Cell Rep 2017;18:248-62. [Crossref] [PubMed]
- Liu J, Lichtenberg T, Hoadley KA, et al. An Integrated TCGA Pan-Cancer Clinical Data Resource to Drive High-Quality Survival Outcome Analytics. Cell 2018;173:400-416.e11. [Crossref] [PubMed]
- Yu G, Wang LG, Han Y, et al. clusterProfiler: an R package for comparing biological themes among gene clusters. OMICS 2012;16:284-7. [Crossref] [PubMed]
- Dai L, Cui X, Zhang X, et al. SARI inhibits angiogenesis and tumour growth of human colon cancer through directly targeting ceruloplasmin. Nat Commun 2016;7:11996. [Crossref] [PubMed]
- Hu M, Xie J, Hou H, et al. Prognostic Value of DNA Methylation-Driven Genes in Clear Cell Renal Cell Carcinoma: A Study Based on Methylation and Transcriptome Analyses. Dis Markers 2020;2020:8817652. [Crossref] [PubMed]
- Huang H, Fu J, Zhang L, et al. Integrative Analysis of Identifying Methylation-Driven Genes Signature Predicts Prognosis in Colorectal Carcinoma. Front Oncol 2021;11:629860. [Crossref] [PubMed]
- Ma J, Zheng B, Goswami S, et al. PD1Hi CD8+ T cells correlate with exhausted signature and poor clinical outcome in hepatocellular carcinoma. J Immunother Cancer 2019;7:331. [Crossref] [PubMed]
- Schraml BU, Hildner K, Ise W, et al. The AP-1 transcription factor Batf controls T(H)17 differentiation. Nature 2009;460:405-9. [Crossref] [PubMed]
- Cheng Y, Li X, Dai Y, et al. Identification of an immune-related risk signature and nomogram predicting the overall survival in patients with endometrial cancer. J Gynecol Oncol 2021;32:e30. [Crossref] [PubMed]
- Moon EK, Ranganathan R, Eruslanov E, et al. Blockade of Programmed Death 1 Augments the Ability of Human T Cells Engineered to Target NY-ESO-1 to Control Tumor Growth after Adoptive Transfer. Clin Cancer Res 2016;22:436-47. [Crossref] [PubMed]
- McLane LM, Abdel-Hakeem MS, Wherry EJ. CD8 T Cell Exhaustion During Chronic Viral Infection and Cancer. Annu Rev Immunol 2019;37:457-95. [Crossref] [PubMed]
(English Language Editor: K. Brown)