A single-sample mRNA molecular classification of bladder cancer predicting prognosis and response to immunotherapy
Introduction
Bladder cancer (BC/BLCA) is one of the most common tumors in the United States, with 81,400 estimated new cases and 17,980 estimated deaths in 2020 (1). At present, there are several evaluation methods to differentiate BC, including the tumour, node, metastasis (TNM) classification system and the pathological grading standard (2,3). However, the great heterogeneity of BC leads to different prognoses, although it is defined as the same stage. It has been reported that the tumor microenvironment (TME) acts as an important factor in tumor initiation, progression, and metastasis (4). Thus, more researchers have focused on the impact of TME components, including immune cells, stromal cells, endothelial cells and cancer-associated fibroblasts (5).
As an immunogenic cancer, the crosstalk between cancer cells and immune cells was gradually recognized in BC. Cancer cells attempt to survive by escaping immune supervision and destruction (6), while immunotherapy plays an important role in harnessing immune cells within or outside the TME, further attacking cancer cells (7). Based on these theories, BC at early and advanced stages can benefit from immunotherapy, bacillus Calmette-Guérin (BCG) intravesical instillation and anti-PD-1/PD-L1 immune checkpoint blockade (ICB), respectively (8). However, there are still a group of patients with nonmuscle-invasive bladder cancer (NMIBC) showing no response to BCG (9), and only 25% of advanced BCs respond to anti-PD-1/PD-L1 ICB (10). As a result, identifying those individuals sensitive to immunotherapy appeared to be essential.
Recently, several studies emphasized the clinical significance of the molecular stratification of muscle-invasive bladder cancer (MIBC), reporting that specific subgroups of MIBC showed better chemotherapy and immunotherapy responses (11-13). According to these findings, Kamoun et al. (14) integrated six classification systems on MIBC and raised a new classification containing six subtypes: luminal papillary, luminal nonspecified, luminal unstable, stroma-rich, basal/squamous, and neuroendocrine-like. Based on this, we performed a more comprehensive and concise grouping standard relying on the immune microenvironment of BC, aiming at establishing immune-related molecular classification to guide the use of immunotherapy. We present the following article in accordance with the STROBE reporting checklist (available at https://tau.amegroups.com/article/view/10.21037/tau-21-887/rc).
Methods
Data acquisition and processing
RNA-seq data of BLCA were downloaded from The Cancer Genome Atlas (TCGA; http://portal.gdc.cancer.gov) project. Samples were obtained and processed according to the protocols from NCI’s Biospecimen Research Database (https://brd.nci.nih.gov/brd/). All genes were transformed into a gene symbol matrix with the use of Ensembl ID data from the Ensembl database (http://asia.ensembl.org/index.html). After matching genes from BLCA and genes from the nCounter® PanCancer Immune Profiling Panel (http://www.nanostring.com/products/gene-expression-panels/gene-expression-panels-overview/hallmarks-cancer-gene-expression-panel-collection/pancancer-immune-profiling-panel), immune genes were screened to conduct cluster analysis. Additionally, phenotype and clinical data were acquired from the TCGA database. Another gene expression dataset of BC was used for validation from the International Cancer Genome Consortium (ICGC; http://dcc.icgc.org/). All data, including sequencing data and relevant clinical data, have been collected and published online, and we conducted this bioinformatic analysis based on these data. The study was conducted in accordance with the Declaration of Helsinki (as revised in 2013).
Unsupervised clustering to obtain immune clusters
First, 770 genes from the nCounter® PanCancer Immune Profiling Panel were matched with genes obtained from the TCGA database (BLCA), selecting 758 immune genes for subsequent analyses. Then, we conducted hierarchical clustering of the patient correlation matrix using correlation as the clustering distance and ward. D as linkage via the R package pheatmap v1.0.12, while the cutree function was used to identify clusters. To identify the optimal number of clusters, we used silhouette analysis of KMeans using the cluster R package. Based on the proper number of clusters, the associations of clinical data and clusters were evaluated; thus, the degree of malignancy could be identified. In addition, we selected two genes with a high frequency of mutation, including KMT2D and TP53, as well as other clustering standards (15), such as methylation, lncRNA, mRNA and reversed-phase protein array (RPPA) clusters, to perform variation analysis compared with our immune clusters.
Nanodissect analysis and lymphoid and myeloid scores
As the tumor immune microenvironment might play an important role in tumor recurrence or progression, we calculated the mRNA expression-based stiffness index (mRNAsi) to explore the similarity of tumor cells and stem cells. Additionally, analyses of the StromalScore, ImmuneScore and ESTIMATEScore were also conducted to explain the association of immune clusters and the immune microenvironment. The algorithm Nanodissect (http://nano.princeton.edu) was used to predict lymphoid and myeloid infiltration, reflecting the average expression of the respective genes in a sample.
Validation with survival data
To confirm that our immune clusters have great value in grouping individuals with different prognoses, we conducted Kaplan-Meier estimator and log-rank tests using the functions Surv, survfit, and survdiff (R package survival v2.42-3). Survival data from the TCGA database and the ICGA database were used for validation.
Binomial logistic regression to predict new immune clusters
With the use of glmnet v2.0-16 R package, we performed binomial logistic regression to predict new immune clusters. Not only is this predictor method highly efficient for smaller cohorts, but it also allows the assignment of a class to single samples without depending on unsupervised clustering. We used gene expression datasets to develop the regression, predicting the categorical response of the two possible outcomes: distributed to the worse prognosis group or not. The prediction efficacy was measured by a relative operating characteristic (ROC) curve using the R package pROC. As the number of clusters was simplified to only two, analysis of clinical features was performed to explain the significance of new immune clusters. Additionally, the risk score of the samples was calculated depending on coefficients from binomial logistic regression. Analyses of clinical characteristics and clusters were performed based on the risk score.
Immune infiltration assessment
To evaluate the effects of immune cells infiltrating the TME, we used the CIBERSORT algorithm to assess immune cell infiltration. With the use of this deconvolution algorithm and reference of gene expression values containing 547 genes, 22 immune cell type proportions from large tumor samples could be predicted via support vector regression. This algorithm provided a P value for each sample to maintain reliability. The CIBERSORT software package was obtained from the developers, with the default signature matrix at 1,000 permutations.
Gene set enrichment analysis (GSEA) and single-sample GSEA (GSVA)
GSEA was performed using the C2 dataset and hallmark dataset, which were downloaded from The Molecular Signatures Database (MsigDB) (https://www.gsea-msigdb.org/gsea/msigdb). Enrichment was assessed by hypergeometric testing.
Gene set analysis was carried out using the GSVA R package v1.30.0. After collecting gene sets for various epithelial mesenchymal transition (EMT), stem cell, proliferation, and cell cycle-related pathways, the enrichment score for each sample was obtained using the gene expression profile.
Independent role of specific types of immune cells
To assess which type of immune cells played an important role in prognosis, we performed a multivariate Cox regression of survival outcomes testing the independent effect of each type. According to the average expression level of each type, these cells were stratified into a high-infiltration group and a low-infiltration group. Moreover, the association between survival outcomes and infiltration level was explored.
Analyses of single nucleotide polymorphisms (SNPs) and copy number variation (CNV) in clusters
SNP data of BC were obtained from the TCGA database, and we analysed SNP mutations using the maftools R package. Level 3 CNV data were downloaded from Fire Browse (http://firebrowse.org/). Analysis of CNV was performed relying on the GISTIC2 module in the Genepattern platform, while we chose hg19 to analyse the Reference Genome File.
The effect of immunotherapy on different immune clusters
The association between immune clusters and response to immunotherapy was predicted by calculating the tumor immune dysfunction and exclusion (TIDE) score using TIDE software (http://tide.dfci.harvard.edu/). This method synthesized the mechanisms of immune evasion and precisely predicted the effects of immune checkpoint inhibitors; thus, we identified a specific group of patients who benefited from immunotherapy.
Statistical analysis
After separating into different clusters, two pair comparisons were performed with the use of unpaired t-test, while multiple comparisons of characteristics were performed with the use of Kruskal-Wallis test. In addition, survival analyses were performed with Kaplan-Meier method. In the comparison of demography baseline of two clusters (clusters B&D vs. clusters A&C), F test was performed. As for multivariate analysis, Cox regressions were performed to investigate contribution of each immune cell type and each gene signature in promoting specific cluster.
Results
Expression differences of immune clusters in BC
RNA-seq data of BC from the TCGA database were matched with the expression of 770 genes from the nCounter® PanCancer Immune Profiling array, an array designed to profile immune infiltration in solid tumors. A total of 758 overlapping immune genes were selected for the subsequent analyses. Based on the expression of these immune genes, patients were divided into various subgroups. Depending on the elbow method, 4 clusters captured the best segmentation (Figure S1). From the heatmap of gene expression, patients in cluster C demonstrated the lowest expression levels of immune genes, while cluster A showed the highest expression levels (Figure 1). Comparing the gene mutation status of BC, we found that TP52 and KMT2D were more likely to be mutated. In our cluster analysis, the highest proportion of these two genes was found in cluster A (Figure 2). Additionally, in the comparisons of our clusters and other current grouping standards, including methylation, lncRNA, mRNA and RPPA clusters, great heterogeneity was also observed among our clusters.
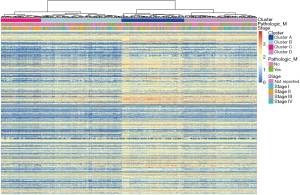
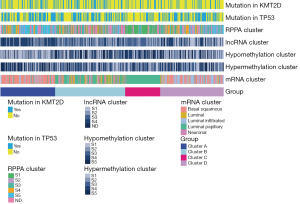
To explore the characteristics of tumors in different clusters, analysis of mRNAsi was performed. We found that the four clusters were significantly correlated with mRNAsi (P<0.0001), of which cluster C had the highest score (Figure 3A). In the analyses of StromalScore, ImmuneScore and ESTIMATEScore, obvious differences were discovered among clusters, with cluster C accompanying the lowest level (Figure 3B-3D). From these primary results, we concluded that clusters A–D reflect distinct immune infiltration and therefore could be defined as immune clusters.
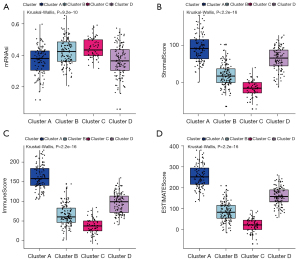
Distinct prognosis and recombination of clusters
In the survival analysis, patients in different clusters had distinct survival outcomes (P=0.012) (Figure 4A). However, cluster B and cluster D demonstrated similar prognoses; thus, we regarded these two clusters as one group (clusters B&D). In the three newly created clusters (cluster A, clusters B&D, cluster C), heterogeneity still existed, with a P value equal to 0.0041 (Figure 4B). For an additional layer of validation, clinical data and gene expression data of 295 BC patients obtained from the ICGC database were used to perform similar analyses. Additionally, a four-cluster grouping was regarded as the best segmentation (Figure 5A), among which cluster C had the best survival outcomes (Figure 5B) with the lowest expression level of immune genes (Figure S2). After redefining clusters B&D, a tendency toward better survival outcomes was observed in cluster C (P=0.058) (Figure 5C).

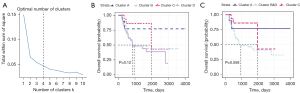
Predicting immune clusters with binomial logistic regression
To develop a general method that could predict the classification of patients precisely and sensitively, a novel prediction classifier was constructed without having to rely on unsupervised clustering. Based on the binomial logistic regression penalized by the lasso method, two clusters (clusters A&C and clusters B&D) with different survival outcomes were obtained. In the lasso regression including 758 genes, 72 key genes were selected to stratify subgroups of BC patients; thus, this group of genes could be regarded as prognostic indicators. Our model predicted the immune clusters with moderate efficacy [area under the curve (AUC) =0.777] (Figure 6A). We found that 65% of the samples assigned to clusters A and C by clustering were predicted to be A and C by the model, while 90.2% of the samples assigned to clusters B and D through clustering were found in clusters B&D using the lasso method (Figure 6B), slightly decreasing the number of samples with poor prognosis. As unsupervised clustering is less reliable in small cohorts, we hypothesized that the Lasso-derived classification would be a better prognostic factor than the clustering method. In the subsequent validation using clinical data, survival analysis showed a more significant difference compared with that of the clustering method, with a P value equal to 0.0089 (Figure 6C). Furthermore, in the analysis of the risk score using the new lasso-derived classification, clusters B&D presented a higher risk score (P<0.001) (Figure 6D).
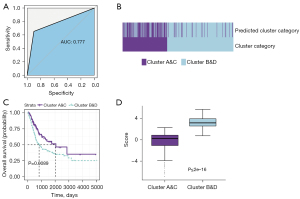
Immune clusters and clinical characteristics
To explore the association between the two clusters and clinical characteristics, we selected several factors potentially related to prognosis. The results showed that clusters B&D had a slightly higher proportion in men and smoking individuals, while the age of patients in the two clusters presented no significant differences (Figure 7A-7C). For tumor stages, clusters B&D had a significantly higher grade status and a higher proportion of T3 stage (Figure 7D,7E), although the clinical T stage distribution showed no significance (P=0.15). Additionally, in the analysis of risk scores stratified by two clusters, clusters B&D had significantly higher risk scores regardless of smoking status and dissection parts than clusters A&C (Figure 8A,8B). However, focusing on the microsatellite instability (MSI) score, no obvious difference was found (P=0.57) (Figure 8C). Combining the previous clustering standard with our immune-related clustering standard, the poor prognosis cluster (B&D) had a higher proportion of luminal and neuronal subtypes (Figure 8D).
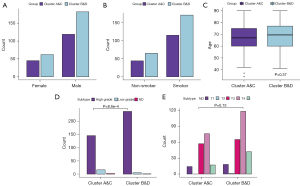
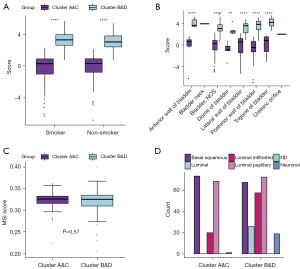
The association of clusters and immune infiltration
In the analysis containing 22 types of immune cells, activated and resting dendritic cells, M0 macrophages, activated mast cells, resting NK cells, activated CD4 memory T cells and CD8 T cells showed distinct expression levels in the two clusters (Figure 9A,9B). In the multivariate regression including all these immune cells, activated CD4 memory T cells and CD8 T cells could be regarded as protective factors, with higher infiltration indicating better prognosis. High infiltration of M0 macrophages, M2 macrophages and neutrophils led to worse survival outcomes (Figure 9C). These results were validated in the Kaplan-Meier survival analysis (Figure 10).
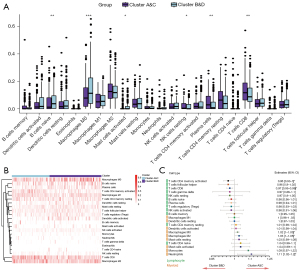
Function of enrichment in clusters
To evaluate functional differences between the two immune clusters, we performed GSEA to reveal potential pathways. GSEA showed that upregulated genes in clusters B&D were mainly enriched in metabolism-related functions, and the most enriched processes in C2 and hallmark collections are denoted (Figure 11A,11B). Through unsupervised clustering of GSVA enrichment scores, two mutually exclusive gene signatures in BC were identified. Samples in clusters A&C were enriched in HALLMARK_DNA_REPAI and HALLMARK_XENOBIOTIC_METABOLISM, while those in clusters B&D were enriched in NOTCH_PATHWAY and DNA_REPLICATION (Figure 11C). In the generalized linear model, metabolism and apoptosis positively contributed to clusters A&C, while proliferation indicated a strong association with clusters B&D (Figure 11D). Overall, combining the results from survival analysis, proliferation probably led to worse prognosis.
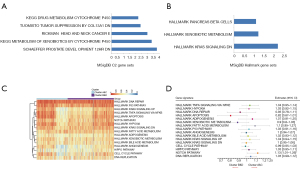
Analyses of SNPs and CNVs
First, in the CNV analysis, we discovered that two clusters (clusters A&C vs. clusters B&D) represented significant differences (Figure 12A). In addition, differences in copy number GISTIC scores between the two clusters were also observed (Figure 12B,12C). With SNP data, we found that the genes with the highest frequency of mutation were TP53 and TTN, accounting for over 40% of mutations in all samples. In the comparison of the two clusters, clusters B&D showed a higher proportion of mutations (Figure 12D).
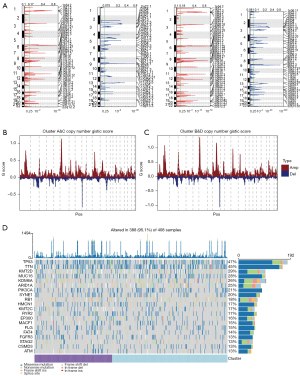
Immune clusters and response to immunotherapy
According to the analysis of the TIDE score, the two clusters showed a strong trend to difference, with a P value equal to 0.053 (Figure 13A). In another analysis of the predicted percentage of immunotherapy benefit, clusters A&C were significantly more likely to obtain benefits from immunotherapy (P=0.023) (Figure 13B).
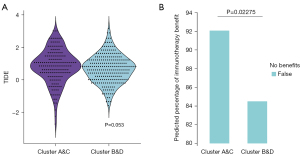
Discussion
As the TME plays an important role in tumorigenesis or progression, we provided a novel immune-related subtype in BC with relevance for prognosis and response to immunotherapy. Through unsupervised clustering with immune-related gene expression, we identified four clusters associated with distinct levels of immune infiltration, specific immune microenvironments and different prognoses.
Although there were several gene signatures to stratify BC patients (16-23), the majority of these studies mainly focused on genes rather than individuals. This approach of classification might lead to certain deficiencies. For a specific BC patient, those high-risk genes and low-risk genes related to survival outcomes probably had interactions. As a result, this unsupervised clustering method based on the expression levels of a group of genes might be ideal when grouping patients. In contrast, binomial logistic regression could assign patients more practically. In our study, we merged two clusters with similar survival outcomes and further refined the grouping standard, developing a simple method predicting whether a sample falls in the poor prognosis cluster (clusters B&D) or not (clusters A&C). With moderate prediction value and a more significant survival difference, this new clustering method could be regarded as a better prognostic indicator.
Through functional enrichment and phenotypic characterization of the immune clusters, we also identified two mutually exclusive states in BC, one associated with metabolism and the other with proliferation. Evidence has shown that highly proliferative tumor cells rely on oxygen supply; in this circumstance, aerobic glycolysis is promoted (24). However, on account of rapid proliferation and reduced mitochondrial function, tumor cell growth demands plenty of oxygen, and a hypoxic microenvironment is induced (25). During this process, hypoxia-inducible factor 1α (HIF-1α) plays an important role in maintaining the survival of hypoxic tumor cells (26) and upregulating a number of genes involved in glycolysis (27). Perhaps more importantly, many tumors can adapt to metabolic changes in the microenvironment and thus have a bimodal metabolic capacity (28). In our analysis, metabolic function enrichment indicated the primary state of tumor growth, with abundant oxygen supply and less aggressive features. As a result, the clinical stages and pathological phenotypes in clusters A&C were superior to those in clusters B&D. Additionally, enrichment of the function of hypoxia helped to explain clusters B&D, probably reflecting the process of overcoming hypoxia and entering rapid proliferation, further causing a worse prognosis.
Using CIBERSORT to infer specific immune cell infiltration, we found that the proliferation state was highly correlated with the infiltration of mast cells, M2 macrophages, monocytes, and neutrophils. A previous study showed that immune contexture presented a strong relationship with cancer prognosis, of which CD8+ T cells and regulatory T cells indicated a positive prognosis, while macrophages, in particular subtype M2, presented a negative prognosis in BC (29). In addition, tumor-infiltrating mast cells indicated worse survival outcomes and higher pathological grades with higher infiltration (30,31), which contributed to the explanation of worse prognosis in clusters B&D. Neutrophils play a complex role, including tumor initiation, growth, proliferation and metastatic spreading (32,33), and it was reported that an elevated neutrophil count was an adverse prognostic factor in metastatic renal cell carcinoma (34). As various types of immune cells interact and play complex roles, a single type of immune cell cannot be evaluated independently.
As an important tumor suppressor gene, TP53 arrested the cell cycle in response to DNA damage, further allowing DNA repair and maintaining genomic integrity (35). Mutant TP53 limited the process of TP53-mediated apoptosis when recognizing DNA damage. Previous studies showed that a higher mutation burden of TP53 led to radiation resistance (36,37). As a result, with a higher mutant TP53 proportion, clusters B&D showed both worse survival outcomes and lower radiation sensitivity. However, in two studies of lung adenocarcinoma and advanced non-small-cell lung cancer, TP53 mutation and KRAS mutation patients obtained more survival benefits through PD-1 blockade immunotherapy (38,39). Due to tumor heterogeneity, this correlation may not exist in BC. In addition, considering functional enrichment and immune infiltration content, TP53 could not be regarded as an independent prognostic factor.
Robertson and colleagues created a detailed molecular classification of MIBC (15). Among the five subtypes (luminal papillary, luminal infiltrated, luminal, basal squamous and neuronal), luminal and neuronal phenotypes presented worse survival outcomes. In our analysis, almost all of these two types were allocated to clusters B&D, which verified the robustness of our results. Kamoun et al. (14) summarized present classification standards and reported that neuroendocrine-like tumors had the worst prognosis but had an association with a potential response to radiotherapy and immune checkpoint inhibitors (40-42). Because of its low proportion, the tendency that cluster B&D responded worse to immunotherapy could not be reversed.
Our analysis synthesized tumor mutation burden, immune infiltration, functional enrichment, SNPs and CNVs to create a novel classification approach. In contrast, we focused on immune-related gene expression and simplified current standards to evaluate the TME from multiple dimensions. Thus, clinicians could select those potentially benefiting from immunotherapy and make valuable decisions. Finally, we found that the profiltration phenotype led to worse prognosis, providing potential therapeutic targets.
There are several limitations of our study. First, data were acquired from retrospective collections and varied in size, composition, and gene expression technology. Second, our molecular classification was established based on gene expression and was further validated using clinical characteristics and survival outcomes. In this way, potential confounding effects could be ignored. Third, whether tumor growth was slowed by inhibiting profiltration-related pathways also needs mechanistic experiments to confirm its efficacy. Fourth, the response to immunotherapy was predicted and lacks relevant clinical data to reveal an immediate correlation; thus, large sample size clinical trials are necessary to validate our results. More empirical validation evidence for the prognostic role of the novel clustering criterion is needed in real-world practice.
Conclusions
Using various characteristics of the immune microenvironment of the BC, we performed a detailed analysis and created a novel clustering criterion based on immune-related genes. Depending on the single-sample mRNA classifier, this classification standard was more appropriate for clinical application. Thus, patients with a worse response to immunotherapy could be selected wisely, further promoting valuable medical decision making.
Acknowledgments
The authors acknowledge Ian Charles Tobias for reviewing the manuscript. We would like to thank Chengfei Liu from the Department of Urologic Surgery, UC Davis School of Medicine for his help in polishing our paper.
Funding: This work was supported by the National Key Research and Development Program of China (grant No. SQ2017YFC0908003), National Natural Science Foundation of China (grant Nos. 81702536, 81770756), Sichuan Science and Technology Program (grant No. 2017HH0063), China Postdoctoral Science Foundation (grant No. 2017M612971), PostDoctor Research Project, West China Hospital, Sichuan University (grant No. 2018HXBH085), and National Clinical Research Center for Geriatrics, West China Hospital, Sichuan University (grant No. Z2018C01).
Footnote
Reporting Checklist: The authors have completed the STROBE reporting checklist. Available at https://tau.amegroups.com/article/view/10.21037/tau-21-887/rc
Peer Review File: Available at https://tau.amegroups.com/article/view/10.21037/tau-21-887/prf
Conflicts of Interest: All authors have completed the ICMJE uniform disclosure form (available at https://tau.amegroups.com/article/view/10.21037/tau-21-887/coif). The authors have no conflicts of interest to declare.
Ethical Statement: The authors are accountable for all aspects of the work in ensuring that questions related to the accuracy or integrity of any part of the work are appropriately investigated and resolved. The study was conducted in accordance with the Declaration of Helsinki (as revised in 2013).
Open Access Statement: This is an Open Access article distributed in accordance with the Creative Commons Attribution-NonCommercial-NoDerivs 4.0 International License (CC BY-NC-ND 4.0), which permits the non-commercial replication and distribution of the article with the strict proviso that no changes or edits are made and the original work is properly cited (including links to both the formal publication through the relevant DOI and the license). See: https://creativecommons.org/licenses/by-nc-nd/4.0/.
References
- Siegel RL, Miller KD, Jemal A. Cancer statistics, 2020. CA Cancer J Clin 2020;70:7-30. [Crossref] [PubMed]
- Sobin LH, Gospodarowicz MK, Wittekind C, et al. TNM classification of malignant tumors. UICC International Union Against Cancer. 7th edition. Hoboken, NJ, USA: Wiley-Blackwell, 2010.
- WHO. WHO Classification of Tumours of the Urinary System and Male Genital Organs. 4th edition. Lyon: WHO, 2016.
- Hinshaw DC, Shevde LA. The Tumor Microenvironment Innately Modulates Cancer Progression. Cancer Res 2019;79:4557-66. [Crossref] [PubMed]
- Hanahan D, Weinberg RA. Hallmarks of cancer: the next generation. Cell 2011;144:646-74. [Crossref] [PubMed]
- Lei X, Lei Y, Li JK, et al. Immune cells within the tumor microenvironment: Biological functions and roles in cancer immunotherapy. Cancer Lett 2020;470:126-33. [Crossref] [PubMed]
- Yost KE, Satpathy AT, Wells DK, et al. Clonal replacement of tumor-specific T cells following PD-1 blockade. Nat Med 2019;25:1251-9. [Crossref] [PubMed]
- Song D, Powles T, Shi L, et al. Bladder cancer, a unique model to understand cancer immunity and develop immunotherapy approaches. J Pathol 2019;249:151-65. [Crossref] [PubMed]
- Herr HW, Dalbagni G. Defining bacillus Calmette-Guerin refractory superficial bladder tumors. J Urol 2003;169:1706-8. [Crossref] [PubMed]
- Gopalakrishnan D, Koshkin VS, Ornstein MC, et al. Immune checkpoint inhibitors in urothelial cancer: recent updates and future outlook. Ther Clin Risk Manag 2018;14:1019-40. [Crossref] [PubMed]
- Rosenberg JE, Hoffman-Censits J, Powles T, et al. Atezolizumab in patients with locally advanced and metastatic urothelial carcinoma who have progressed following treatment with platinum-based chemotherapy: a single-arm, multicentre, phase 2 trial. Lancet 2016;387:1909-20. [Crossref] [PubMed]
- Seiler R, Ashab HAD, Erho N, et al. Impact of Molecular Subtypes in Muscle-invasive Bladder Cancer on Predicting Response and Survival after Neoadjuvant Chemotherapy. Eur Urol 2017;72:544-54. [Crossref] [PubMed]
- Mariathasan S, Turley SJ, Nickles D, et al. TGFβ attenuates tumour response to PD-L1 blockade by contributing to exclusion of T cells. Nature 2018;554:544-8. [Crossref] [PubMed]
- Kamoun A, de Reyniès A, Allory Y, et al. A Consensus Molecular Classification of Muscle-invasive Bladder Cancer. Eur Urol 2020;77:420-33. [Crossref] [PubMed]
- Robertson AG, Kim J, Al-Ahmadie H, et al. Comprehensive Molecular Characterization of Muscle-Invasive Bladder Cancer. Cell 2017;171:540-556.e25. [PubMed]
- Jiang P, Gu S, Pan D, et al. Signatures of T cell dysfunction and exclusion predict cancer immunotherapy response. Nat Med 2018;24:1550-8. [Crossref] [PubMed]
- Quan J, Zhang W, Yu C, et al. Bioinformatic identification of prognostic indicators in bladder cancer. Biomark Med 2020;14:1243-54. [Crossref] [PubMed]
- Chen X, Jin Y, Gong L, et al. Bioinformatics Analysis Finds Immune Gene Markers Related to the Prognosis of Bladder Cancer. Front Genet 2020;11:607. [Crossref] [PubMed]
- Wang Y, Chen L, Yu M, et al. Immune-related signature predicts the prognosis and immunotherapy benefit in bladder cancer. Cancer Med 2020;9:7729-41. [Crossref] [PubMed]
- Qiu H, Hu X, He C, et al. Identification and Validation of an Individualized Prognostic Signature of Bladder Cancer Based on Seven Immune Related Genes. Front Genet 2020;11:12. [Crossref] [PubMed]
- Jiang W, Zhu D, Wang C, et al. An immune relevant signature for predicting prognoses and immunotherapeutic responses in patients with muscle-invasive bladder cancer (MIBC). Cancer Med 2020;9:2774-90. [Crossref] [PubMed]
- Das S, Camphausen K, Shankavaram U. Cancer-Specific Immune Prognostic Signature in Solid Tumors and Its Relation to Immune Checkpoint Therapies. Cancers (Basel) 2020;12:2476. [Crossref] [PubMed]
- Song BN, Kim SK, Mun JY, et al. Identification of an immunotherapy-responsive molecular subtype of bladder cancer. EBioMedicine 2019;50:238-45. [Crossref] [PubMed]
- Boroughs LK, DeBerardinis RJ. Metabolic pathways promoting cancer cell survival and growth. Nat Cell Biol 2015;17:351-9. [Crossref] [PubMed]
- Bensaad K, Harris AL. Hypoxia and metabolism in cancer. Adv Exp Med Biol 2014;772:1-39. [Crossref] [PubMed]
- Brahimi-Horn MC, Pouysségur J. Hypoxia in cancer cell metabolism and pH regulation. Essays Biochem 2007;43:165-78. [Crossref] [PubMed]
- Denko NC. Hypoxia, HIF1 and glucose metabolism in the solid tumour. Nat Rev Cancer 2008;8:705-13. [Crossref] [PubMed]
- Woolbright BL, Ayres M, Taylor JA 3rd. Metabolic changes in bladder cancer. Urol Oncol 2018;36:327-37. [Crossref] [PubMed]
- Fridman WH, Zitvogel L, Sautès-Fridman C, et al. The immune contexture in cancer prognosis and treatment. Nat Rev Clin Oncol 2017;14:717-34. [Crossref] [PubMed]
- Liu Z, Zhu Y, Xu L, et al. Tumor stroma-infiltrating mast cells predict prognosis and adjuvant chemotherapeutic benefits in patients with muscle invasive bladder cancer. Oncoimmunology 2018;7:e1474317. [Crossref] [PubMed]
- Kim JH, Kang YJ, Kim DS, et al. The relationship between mast cell density and tumour grade in transitional cell carcinoma of the bladder. J Int Med Res 2011;39:1675-81. [Crossref] [PubMed]
- Swierczak A, Mouchemore KA, Hamilton JA, et al. Neutrophils: important contributors to tumor progression and metastasis. Cancer Metastasis Rev 2015;34:735-51. [Crossref] [PubMed]
- Coffelt SB, Wellenstein MD, de Visser KE. Neutrophils in cancer: neutral no more. Nat Rev Cancer 2016;16:431-46. [Crossref] [PubMed]
- Heng DY, Xie W, Regan MM, et al. Prognostic factors for overall survival in patients with metastatic renal cell carcinoma treated with vascular endothelial growth factor-targeted agents: results from a large, multicenter study. J Clin Oncol 2009;27:5794-9. [Crossref] [PubMed]
- Zilfou JT, Lowe SW. Tumor suppressive functions of p53. Cold Spring Harb Perspect Biol 2009;1:a001883. [Crossref] [PubMed]
- Hinata N, Shirakawa T, Zhang Z, et al. Radiation induces p53-dependent cell apoptosis in bladder cancer cells with wild-type- p53 but not in p53-mutated bladder cancer cells. Urol Res 2003;31:387-96. [Crossref] [PubMed]
- Leszczynska KB, Foskolou IP, Abraham AG, et al. Hypoxia-induced p53 modulates both apoptosis and radiosensitivity via AKT. J Clin Invest 2015;125:2385-98. [Crossref] [PubMed]
- Dong ZY, Zhong WZ, Zhang XC, et al. Potential Predictive Value of TP53 and KRAS Mutation Status for Response to PD-1 Blockade Immunotherapy in Lung Adenocarcinoma. Clin Cancer Res 2017;23:3012-24. [Crossref] [PubMed]
- Assoun S, Theou-Anton N, Nguenang M, et al. Association of TP53 mutations with response and longer survival under immune checkpoint inhibitors in advanced non-small-cell lung cancer. Lung Cancer 2019;132:65-71. [Crossref] [PubMed]
- Pawlik TM, Keyomarsi K. Role of cell cycle in mediating sensitivity to radiotherapy. Int J Radiat Oncol Biol Phys 2004;59:928-42. [Crossref] [PubMed]
- Horsman MR, Overgaard J. The impact of hypoxia and its modification of the outcome of radiotherapy. J Radiat Res 2016;57:i90-8. [Crossref] [PubMed]
- Kim J, Kwiatkowski D, McConkey DJ, et al. The Cancer Genome Atlas Expression Subtypes Stratify Response to Checkpoint Inhibition in Advanced Urothelial Cancer and Identify a Subset of Patients with High Survival Probability. Eur Urol 2019;75:961-4. [Crossref] [PubMed]