Construction of diagnostic and subtyping models for renal cell carcinoma by genome-wide DNA methylation profiles
Introduction
Renal cell carcinoma (RCC) is one of the most common urological cancers originating from the renal epithelium; it accounts for >90% of kidney cancers (1,2). Approximately 30% of patients already have locally advanced or metastatic disease, and 1 of 3 patients will die from this disease, highlighting its poor prognosis (3). RCC is classified into several subtypes by pathology, including kidney renal clear cell carcinoma (KIRC), kidney renal papillary cell carcinoma (KIRP), chromophobe, collecting duct, and unclassified RCC. KIRC and KIRP are the two most common subtypes, representing nearly 90% of RCCs (2,3). However, RCC, including its subtypes, cannot be diagnosed accurately, and an accurate and timely diagnosis of RCC is important. Thus, there is a need for a high-sensitivity method to diagnose RCC.
DNA methylation typically refers to cytosine methylation, which is a DNA modification that is generally related to transcriptional silencing (4,5). There are different methylation patterns between disease and normal samples, and changes in the expression of genes could promote oncogenesis (6-8). Understanding epigenetic changes is a promising approach for better diagnosing renal carcinoma. The CpG-based methylation profile may be of use as a biomarker in the diagnosis of RCC. DNA methylation has been observed as a hallmark in many types of urologic cancers, including KIRC, bladder urothelial carcinoma (BLCA) and prostate adenocarcinoma (PRAD) (9-12). However, the methylation site status has not been used as a marker to identify different RCC subtypes.
In this study, we analyzed the frequently observed RCC subtypes (KIRC and KIRP) from an integrated and comparative perspective and found that methylation markers could distinguished KIRC and KIRP in the diagnosis of RCC. We compared the differential methylation profiles of KIRC and KIRP cancer tissues with matched normal tissues by analyzing 485,000 CpG sites. We identified 15 methylation markers specific to KIRC and KIRP with high sensitivity and accuracy, and then we verified these 15 methylation markers in the testing group. Using these 15 sites, we calculated the diagnostic score and subtyping score to distinguish normal tissues, RCC tissues and different RCC subtypes. Through the scoring evaluation system, we obtained good classification performance, and a scoring system is more intuitive and practicable for clinical application. Importantly, 10 of 15 methylation marker-associated genes also had value in predicting prognosis. These results show that DNA methylation may be used as a biomarker in the diagnosis of renal cell cancer and to discern KIRC from KIRP.
We present the following article in accordance with the STARD reporting checklist (available at https://dx.doi.org/10.21037/tau-21-674).
Methods
Data sources
The 450K methylation array data were obtained from The Cancer Genome Atlas (TCGA, https://www.cancer.gov/about-nci/organization/ccg/research/structural-genomics/tcga) datasets for the training group of KIRC samples (n=216), KIRP (n=183), and matched adjacent normal tissue samples (n=136), as well as the testing group of KIRC samples (n=108), KIRP samples (n=92) and matched normal samples (n=69). The study was conducted in accordance with the Declaration of Helsinki (as revised in 2013).
The taining and testing datasets
The datasets of KIRC, KIRP and normal tissue were randomly divided into a training group and a testing group in a 2:1 ratio, in which the normal tissue group was a combination of KIRP-adjacent normal tissue and KIRC-adjacent normal tissue.
Building the diagnostic and subtyping models
All analysis was conducted in R version 4.0.3. First, the noise signal was removed from the training group using the ‘Limma moderated t-test’ with the R package ‘limma’ (version 3.44.3). Then, the least absolute shrinkage and selection operator (LASSO) method was applied by the R package ‘glmnet’ (version 4.1.1), with multinomial distribution and 10-fold cross-validation. Finally, a diagnostic prediction model was constructed using a logistic regression method.
All statistical tests were two-sided. P<0.05 was considered to be statistically significant.
T-SNE analysis and PCA
t-Distributed stochastic neighbor embedding (t-SNE) analysis was performed by the R package t-sne (version 0.1-3). PCA was performed by R function princomp and visualized by the first two principal components.
The diagnostic score and subtyping score
We defined the diagnostic score as the negative logarithmic multiplication of beta values of cg20740711 and cg22274117. We calculated the mean values of cg06215107, cg08223003, cg12496156, cg22571393, cg03290131, cg08163918, cg09643398, cg16284684, cg23264429 and cg24170040 as value 1 and the mean values of cg05548488, cg16283183 and cg23528791 as value 2. We defined the subtyping score as the 10-fold subtraction of value 1 and value 2.
Gene enrichment analysis
GO enrichment was analyzed online by DAVID 6.8 (https://david.ncifcrf.gov).
ROC curve
The R package pROC (version 1.14.0) was used for ROC analysis to compare the abilities of various models to distinguish normal tissues, KIRC and KIRP by area under the curve (AUC) analysis.
Survival analysis
In this study, survival analysis was conducted online by GEPIA (https://gepia.cancer-pku.cn/), with data from TCGA and GTEx. Kaplan-Meier Plotter was used to generate the survival curve.
Statistical analysis
We used R software (version 4.0.3) to perform all statistical analyses. In this study, Student’s t-test was used to calculate the global methylation levels of KIRC, KIRP and their adjacent tissues, and the difference in DNA methylation between KIRC normal and KIRP normal tissues. All p values are based on two-sided statistical analysis, and P<0.05 was considered to indicate statistical significance.
Results
Landscape of DNA methylation for the different subtypes of RCC
The methylation profiles of KIRC samples (n=324), KIRP samples (n=275) and adjacent nontumor samples of each subtype of RCC (KIRC normal n=160, KIRP normal n=45) were obtained from The Cancer Genome Atlas (TCGA) database. We used tSNE and PCA to visualize the genome-wide DNA methylation of different samples and found that the DNA methylation patterns showed pathologic differences (Figure 1A,1B). In addition, we found that there was no obvious difference between the adjacent normal tissue of the two types of RCCs.
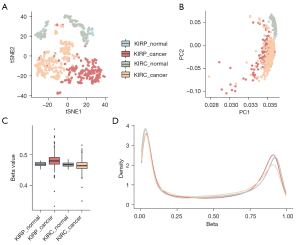
Furthermore, we calculated the global methylation levels of KIRC, KIRP and their adjacent tissues. Compared to normal tissue, KIRP exhibited global hypermethylation (P=5.2×10−7, Student’s t-test). However, KIRC exhibited hypomethylation globally (P=8.0×10−4, compared with normal, Student’s t-test, Figure 1C). Based on the DNA methylation level of normal tissue, the opposite changing direction was altered in, when comparing KIRC with KIRP tissues. These results may indicate different mechanisms of development for the two RCC subtypes that originate from the same organ. At the same time, we found no significant difference between the adjacent tissues of the two subtypes at the global DNA methylation level, which was consistent with the results in Figure 1A,1B. The DNA methylation levels of all target sites in the array demonstrated the known bimodal distribution in KIRC, KIRP and their adjacent tissue (Figure 1D), and we observed that the low-methylation peak was decreased in cancer samples compared with the adjacent normal tissues. However, the high-methylation peak was decreased in KIRC and increased in KIRP, which was consistent with the change in direction of genome-wide methylation.
In addition, we also found that the two types of normal tissues exhibited similar bimodal distribution patterns. Since there was no difference in the genome-wide methylation level between the two types of normal tissues, we merged the two types of normal tissues in the following study.
Establishment of the model to discriminate RCC subtypes
To establish and validate the model to distinguish different subtypes of RCC, we randomly split the three types of samples into training and testing groups at a 2:1 ratio. After splitting, we obtained a training group containing 535 samples and a testing group containing 269 samples. Then, we attempted to build the model using the ‘limma moderated t-test’ method with the training group. The research route is shown in Figure 2.
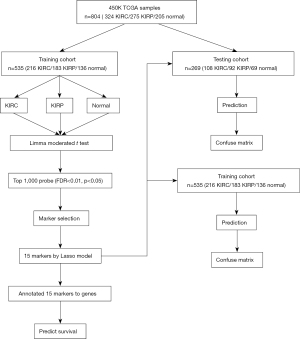
The ‘Limma moderated t-test’ method was employed with empirical Bayes for removing the noise signal, and the Benjamini-Hochberg procedure was used to restrict P<0.05 and the false discovery rate (FDR) at a significance level of 0.01 (13). The top 1,000 sites of the ‘Limma moderated t-test’ results were selected according to the P value between KIRC and normal tissue. The same processes were performed for comparison between KIRP and normal tissue and between KIRC and KIRP. Merging all sites in three comparisons, we obtained 2,603 sites for further study.
We used the combination of 2,603 sites to classify the samples in the training group and testing group, and the results were illustrated by an unsupervised heatmap and t-SNE cluster analysis. We found that the 2603 sites were able to discriminate cancer from normal renal tissues. Furthermore, the model could distinguish KIRC and KIRP in both the training and testing groups (Figure 3).
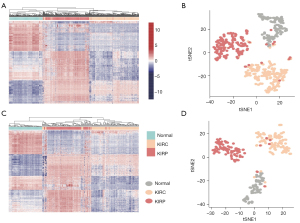
Identification of the RCC subtypes by 15 sites
We used the least absolute shrinkage and selection operator (LASSO) method with multinomial distribution and 10-fold cross-validation to further decrease the number of sites for discrimination (Figure 4A). After LASSO, we obtained 15 methylation sites that could identify KIRC, KIRP and normal tissues (Table 1). More specifically, cg20740711 and cg22274117 can be used to distinguish between normal tissue and cancer tissue. The hypermethylation of cg06215107, cg08223003, cg12496156 and cg22571393, combined with the hypomethylation of cg05548488, cg16283183 and cg23528791, indicated KIRP. Similarly, the hypomethylation of cg03290131, cg08163918, cg09643398, cg16284684, cg23264429 and cg24170040 indicated KIRC.
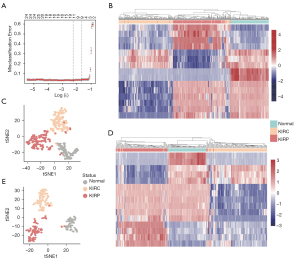
Table 1
Marker | Chromosome | Start | End | Coefficient | |
---|---|---|---|---|---|
Normal | cg20740711 | chr2 | 112692702 | 112692703 | 2.548 |
cg22274117 | chr6 | 16713382 | 16713383 | 2.018 | |
KIRC | cg09643398 | chr3 | 187736694 | 187736695 | –0.017 |
cg23264429 | chr10 | 88882246 | 88882247 | –0.104 | |
cg03290131 | chr10 | 110504073 | 110504074 | –0.29 | |
cg16284684 | chr7 | 1124427 | 1124428 | –0.474 | |
cg24170040 | chr14 | 33946550 | 33946551 | –0.951 | |
cg08163918 | chr12 | 57234871 | 57234872 | –1.119 | |
KIRP | cg06215107 | chr4 | 150583573 | 150583574 | 1.586 |
cg22571393 | chr13 | 112843994 | 112843995 | 0.249 | |
cg08223003 | chr8 | 133273077 | 133273078 | 0.191 | |
cg12496156 | chr2 | 217801325 | 217801326 | 0.062 | |
cg16283183 | chr3 | 45677313 | 45677314 | –0.099 | |
cg05548488 | chr3 | 50621041 | 50621042 | –0.212 | |
cg23528791 | chr6 | 154478529 | 154478530 | –1.06 |
KIRC, kidney renal clear cell carcinoma; KIRP, kidney renal papillary cell carcinoma.
To test these 15 sites as potential diagnostic markers, we calculated the classification accuracy. Among the training group of 535 samples, the classification sensitivity reached 95.63%, 94.44% and 100% in KIRP, KIRC and normal tissues, respectively. The total sensitivity reached 96.26% (Table 2). Unsupervised hierarchical clustering and tSNE of these 15 markers were performed, and the results were visualized (Figure 4B,4C). Next, we tested the discrimination performance of these 15 markers in the testing group of 269 samples, and the classification sensitivity reached 92.39%, 95.37% and 100% in KIRP, KIRC and normal tissues, respectively (Table 3). Unsupervised hierarchical clustering and tSNE of these 15 markers were performed, and the results were visualized (Figure 4D,4E). There was coincidence with the training group via a total sensitivity of 97.03%. In particular, the true negative ratio was 100%. This result indicates that people without RCC will not be misdiagnosed, which improves the accuracy of the diagnosis. And the methylation level of 15 markers in KIRC, KIRP and normal tissue were shown in Figure 5.
Table 2
Training cohort | Normal kidney | KIRC | KIRP |
---|---|---|---|
Normal kidney | 136 | 1 | 1 |
KIRC | 0 | 204 | 7 |
KIRP | 0 | 11 | 175 |
Total | 136 | 216 | 183 |
Correct | 136 | 204 | 175 |
Sensitivity (%) | 100.00 | 94.44 | 95.63 |
Total sensitivity (%) | 96.26 |
KIRC, kidney renal clear cell carcinoma; KIRP, kidney renal papillary cell carcinoma.
Table 3
Test cohort | Normal kidney | KIRC | KIRP |
---|---|---|---|
Normal kidney | 69 | 0 | 0 |
KIRC | 0 | 103 | 3 |
KIRP | 0 | 5 | 89 |
Total | 69 | 108 | 92 |
Correct | 69 | 103 | 89 |
Sensitivity (%) | 100.00 | 95.37 | 96.74 |
Total sensitivity (%) | 97.03 |
KIRC, kidney renal clear cell carcinoma; KIRP, kidney renal papillary cell carcinoma.
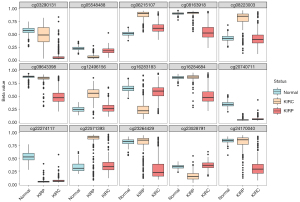
The potential clinical application of the 15 markers
According to the above results, 2 of the 15 markers can be used to distinguish RCC from normal tissues, and the remaining 13 markers can be used for RCC subtyping. In further consideration of the usability for clinicians, we calculated a diagnostic score using the two markers cg20740711 and cg22274117. From the training group, the threshold was set to 3, and we obtained the best classification performance. The sample will be reported as RCC if the diagnostic score is more than 3, and the reverse will be reported as normal tissue (Figure 6A). ROC curve analysis of the diagnosis score for all 535 samples produced an AUC of 1 with specificity and sensitivity of 1.00 and 1.00, respectively (Figure 6B). Then, we validated the diagnosis in the testing group, and ROC curve analysis of the diagnosis score for all 269 samples produced an AUC of 0.99 with specificity and sensitivity of 1.00 and 0.99, respectively. Only one KIRP sample was misclassified as normal tissue (Figure 6C,6D). To confirm the effectiveness of the diagnostic score, we performed Student’s t-test for cg20740711 and cg22274117 between the two types of normal tissues. The results did not exhibit a significant difference, with p values of 0.30 and 0.82 in cg20740711 and cg22274117, respectively (Table S1).
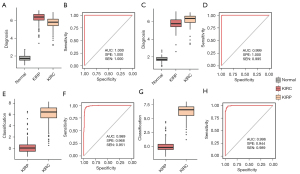
For RCC subtyping, we used the remaining 13 markers to calculate a subtyping score. To obtain the best classification performance, a threshold set as 2.26 was selected in the training group. RCC tissues will be reported as KIRC if the diagnostic score is more than 2.26, and the reverse will be reported as KIRP (Figure 6E). ROC curve analysis of the subtyping score for all 399 samples produced an AUC of 0.98 with specificity and sensitivity of 0.96 and 0.95, respectively (Figure 6F). Then, we validated the diagnosis in the testing group (Figure 6G), and ROC curve analysis of the subtyping score for all 200 samples produced an AUC of 0.99 with specificity and sensitivity of 0.94 and 0.98, respectively (Figure 6H).
The quantified diagnostic score and subtyping score may become direct indicators to assist clinicians in making a diagnosis.
Discussion
The identification of precise biomarkers for cancer subtypes has become increasingly important in recent years, especially in the implementation of personalized medicine for clinicians. Many studies have attempted to uncover biomarkers that are applicable for early disease detection and possess prognostic and predictive capabilities (14-16). DNA methylation analysis requires a small amount of tissue to obtain enough DNA. This, permits the use of lower-quality biopsies, which may be an ideal diagnostic model for many diseases. It may have utility in diagnosing metastatic lesions, particularly when the primary cancer type is unknown. Cancer-specific changes include CpG hypermethylation in gene promoters, hypomethylation of non-CpG islands, and an increase in methylation variation (7,17). In urological cancers, many studies have investigated specific methylation levels or genome-wide profiles of kidney cancer, bladder cancer and prostate cancer (18-22). However, few studies have attempted to distinguish between different subtypes and normal tissues in RCC. There are many studies focusing on constructing a predictive model to diagnose RCC rather than differentiating between its subtypes. KIRC and KIRP are the most common subtypes of RCC, and their pathology, location, cell type of origin, and genetic alterations vary, making it even more important to characterize each subtype. From this perspective, we first determined differentially methylated CpG sites to distinguish KIRC samples, KIRP samples and normal samples and used methylation signatures along with gene annotations to predict prognostic sites. By applying the moderated t-test and LASSO for 485,000 CpG sites along with a machine learning algorithm, we discovered a novel and reliable set of molecular marker classifiers. Meanwhile, a 15-methylation marker panel was used for subtypes of RCC to construct a diagnostic prediction model. Moreover, the diagnostic sensitivity reached more than 95%; thus, this panel has the potential to be used as a new set of clinical markers for the diagnosis of RCC subtypes.
With such high sensitivity and specificity, the 15 methylation markers can be used to relatively accurately distinguish between renal carcinoma and normal tissue, as well as to distinguish different renal carcinoma subtypes. Therefore, these 15 markers were quantitatively scored and could be applied clinically. By selecting 3 and 2.26 as the diagnostic score and subtype score, respectively, we obtained relatively accurate classification performance. We could distinguish between normal tissue and RCC by the diagnostic score and distinguish between KIRC and KIRP by the subtyping score. Because the classification results are quantified in the form of scores, it is more convenient for clinical pathologists to issue a diagnostic report according to the score. Reports in the form of a score is intuitive and easy to adopt in clinical practice, and thus, this model could become a potential clinical diagnostic indicator.
Considering the good performance of the 15 markers, their underlying mechanism was explored. Then, we annotated these 15 methylation markers to the genome and found that except for one marker, cg20740711, that was located in the intergenic region, 14 markers were located in the gene region (Table S2). In this study, ATXN1 was the only gene to be annotated, and it could distinguish normal tissue from RCC. ATXN1 may play a key role in growth and development or tumorigenesis and has been reported to be associated with the development of prostate adenocarcinoma and stomach adenocarcinoma (23). In addition, we determined the expression levels and performed survival analysis for the 14 genes and found that 10 genes showed significant survival differences. Of the 10 genes, 9 were related to KIRP survival, and 2 were related to KIRC survival (Figure S1). These 10 genes have been observed in many studies to be associated with poor prognosis in many types of cancer (24-27), especially STAMBPL1, EGLN3, SHMT2, and LIMD1, which have been validated to be associated with the development of kidney cancer (28-31). These results validate the accuracy of our gene selection strategy. Significantly, the LRBA gene was associated with the survival of both KIRC and KIRP, and it has been reported that LRBA also plays an important role in the development of colorectal cancer and breast cancer. The mutational features of LRBA have also been reported in KIRP. In the GO analysis of the 10 genes, response to hypoxia and regulation of cell proliferation pathways were enriched. Although the results of the enrichment analysis were not statistically significant, on account of the fundamental functions of the two pathways in RCC, we speculate that they could function in RCC diagnosis, prognosis and subtype classification.
One of the limitations of this study was the retrospective nature of the cohort. Further prospective studies are warranted, and it is necessary to expand the sample quantity to explore the clinical applications of methylation sequencing for diagnosis. The genes we annotated should be further researched to validate their relationship with prognosis. Another limitation was that the selected genes need further functional verification. Moreover, urine-based diagnostic assays represent a new, noninvasive diagnostic technique, especially for RCC. DNA methylation markers that are detectable in urine could be used to predict urological cancers (32,33). These methylation markers may be useful for diagnosing subtypes of RCC in urine samples. Noninvasive methods for the diagnosis of renal cancer will be the way forward. It is also our research direction in the future.
Overall, these findings raise the possibility that methylation patterns may help identify subtypes of RCC and predict the prognosis of different subtypes. Further studies are warranted to explore the use of methylation sequencing in clinical applications to help personalize the treatment of RCC patients.
Acknowledgments
Funding: This work was supported by the National Key R&D Program of China (grant no. 2019YFA09006001), the National Natural Science Foundation of China [81872083, 81972379, 81772703 and 81972380], Clinical Medicine Plus X - Young Scholars Project, Peking University, the Fundamental Research Funds for the Central Universities [PKU2020LCXQ014], Wuxi “Taihu Talents Program” Medical and Health High-level Talents Project.
Footnote
Reporting Checklist: The authors have completed the STARD reporting checklist. Available at https://dx.doi.org/10.21037/tau-21-674
Conflicts of Interest: All authors have completed the ICMJE uniform disclosure form (available at https://dx.doi.org/10.21037/tau-21-674). XL serves as an unpaid editorial board member of Translational Andrology and Urology. LZ serves as an unpaid editorial board member of Translational Andrology and Urology from March 2020 to February 2022. The other authors have no conflicts of interest to declare.
Ethical Statement: The authors are accountable for all aspects of the work in ensuring that questions related to the accuracy or integrity of any part of the work are appropriately investigated and resolved. The study was conducted in accordance with the Declaration of Helsinki (as revised in 2013).
Open Access Statement: This is an Open Access article distributed in accordance with the Creative Commons Attribution-NonCommercial-NoDerivs 4.0 International License (CC BY-NC-ND 4.0), which permits the non-commercial replication and distribution of the article with the strict proviso that no changes or edits are made and the original work is properly cited (including links to both the formal publication through the relevant DOI and the license). See: https://creativecommons.org/licenses/by-nc-nd/4.0/.
References
- Capitanio U, Montorsi F. Renal cancer. Lancet 2016;387:894-906. [Crossref] [PubMed]
- Hsieh JJ, Purdue MP, Signoretti S, et al. Renal cell carcinoma. Nat Rev Dis Primers 2017;3:17009. [Crossref] [PubMed]
- Cairns P. Renal cell carcinoma. Cancer Biomark 2010;9:461-73. [Crossref] [PubMed]
- Jaenisch R, Bird A. Epigenetic regulation of gene expression: how the genome integrates intrinsic and environmental signals. Nat Genet 2003;33:245-54. [Crossref] [PubMed]
- Vaissière T, Sawan C, Herceg Z. Epigenetic interplay between histone modifications and DNA methylation in gene silencing. Mutat Res 2008;659:40-8. [Crossref] [PubMed]
- Egger G, Liang G, Aparicio A, et al. Epigenetics in human disease and prospects for epigenetic therapy. Nature 2004;429:457-63. [Crossref] [PubMed]
- Herman JG, Baylin SB. Gene silencing in cancer in association with promoter hypermethylation. N Engl J Med 2003;349:2042-54. [Crossref] [PubMed]
- Feinberg AP, Tycko B. The history of cancer epigenetics. Nat Rev Cancer 2004;4:143-53. [Crossref] [PubMed]
- Hanahan D, Weinberg RA. Hallmarks of cancer: the next generation. Cell 2011;144:646-74. [Crossref] [PubMed]
- Cancer Genome Atlas Research Network. Comprehensive molecular characterization of clear cell renal cell carcinoma. Nature 2013;499:43-9. [Crossref] [PubMed]
- Cancer Genome Atlas Research Network. Comprehensive molecular characterization of urothelial bladder carcinoma. Nature 2014;507:315-22. [Crossref] [PubMed]
- Cancer Genome Atlas Research Network. The Molecular Taxonomy of Primary Prostate Cancer. Cell 2015;163:1011-25. [Crossref] [PubMed]
- Benjamini Y, Hochberg Y. Controlling the False Discovery Rate: A Practical and Powerful Approach to Multiple Testing. Journal of the Royal Statal Society Series B: Methodological 1995;57:289-300. [Crossref]
- Golovastova MO, Korolev DO, Tsoy LV, et al. Biomarkers of Renal Tumors: the Current State and Clinical Perspectives. Curr Urol Rep 2017;18:3. [Crossref] [PubMed]
- Mbeutcha A, Lucca I, Mathieu R, et al. Current Status of Urinary Biomarkers for Detection and Surveillance of Bladder Cancer. Urol Clin North Am 2016;43:47-62. [Crossref] [PubMed]
- Tanase CP, Codrici E, Popescu ID, et al. Prostate cancer proteomics: Current trends and future perspectives for biomarker discovery. Oncotarget 2017;8:18497-512. [Crossref] [PubMed]
- Taby R, Issa JP. Cancer epigenetics. CA Cancer J Clin 2010;60:376-92. [Crossref] [PubMed]
- Costa VL, Henrique R, Danielsen SA, et al. TCF21 and PCDH17 methylation: An innovative panel of biomarkers for a simultaneous detection of urological cancers. Epigenetics 2011;6:1120-30. [Crossref] [PubMed]
- Henrique R, Jerónimo C. Molecular detection of prostate cancer: a role for GSTP1 hypermethylation. Eur Urol 2004;46:660-9; discussion 669. [Crossref] [PubMed]
- Monteiro-Reis S, Leça L, Almeida M, et al. Accurate detection of upper tract urothelial carcinoma in tissue and urine by means of quantitative GDF15, TMEFF2 and VIM promoter methylation. Eur J Cancer 2014;50:226-33. [Crossref] [PubMed]
- Zuiverloon TC, Beukers W, van der Keur KA, et al. A methylation assay for the detection of non-muscle-invasive bladder cancer (NMIBC) recurrences in voided urine. BJU Int 2012;109:941-8. [Crossref] [PubMed]
- Peng D, Ge G, Xu Z, et al. Diagnostic and prognostic biomarkers of common urological cancers based on aberrant DNA methylation. Epigenomics 2018;10:1189-99. [Crossref] [PubMed]
- Wong D, Lounsbury K, Lum A, et al. Transcriptomic analysis of CIC and ATXN1L reveal a functional relationship exploited by cancer. Oncogene 2019;38:273-90. [Crossref] [PubMed]
- Zhang R, Lai L, He J, et al. EGLN2 DNA methylation and expression interact with HIF1A to affect survival of early-stage NSCLC. Epigenetics 2019;14:118-29. [Crossref] [PubMed]
- Ye J, Fan J, Venneti S, et al. Serine catabolism regulates mitochondrial redox control during hypoxia. Cancer Discov 2014;4:1406-17. [Crossref] [PubMed]
- Ito H, Watari K, Shibata T, et al. Bidirectional Regulation between NDRG1 and GSK3β Controls Tumor Growth and Is Targeted by Differentiation Inducing Factor-1 in Glioblastoma. Cancer Res 2020;80:234-48. [Crossref] [PubMed]
- Ghosh S, Ghosh A, Maiti GP, et al. LIMD1 is more frequently altered than RB1 in head and neck squamous cell carcinoma: clinical and prognostic implications. Mol Cancer 2010;9:58. [Crossref] [PubMed]
- Shahriyar SA, Woo SM, Seo SU, et al. Cepharanthine Enhances TRAIL-Mediated Apoptosis Through STAMBPL1-Mediated Downregulation of Survivin Expression in Renal Carcinoma Cells. Int J Mol Sci 2018;19:3280. [Crossref] [PubMed]
- Reustle A, Di Marco M, Meyerhoff C, et al. Integrative -omics and HLA-ligandomics analysis to identify novel drug targets for ccRCC immunotherapy. Genome Med 2020;12:32. [Crossref] [PubMed]
- Wang H, Chong T, Li BY, et al. Evaluating the clinical significance of SHMT2 and its co-expressed gene in human kidney cancer. Biol Res 2020;53:46. [Crossref] [PubMed]
- Zhang CS, Liu Q, Li M, et al. RHOBTB3 promotes proteasomal degradation of HIFα through facilitating hydroxylation and suppresses the Warburg effect. Cell Res 2015;25:1025-42. [Crossref] [PubMed]
- Dahmcke CM, Steven KE, Larsen LK, et al. A Prospective Blinded Evaluation of Urine-DNA Testing for Detection of Urothelial Bladder Carcinoma in Patients with Gross Hematuria. Eur Urol 2016;70:916-9. [Crossref] [PubMed]
- Renard I, Joniau S, van Cleynenbreugel B, et al. Identification and validation of the methylated TWIST1 and NID2 genes through real-time methylation-specific polymerase chain reaction assays for the noninvasive detection of primary bladder cancer in urine samples. Eur Urol 2010;58:96-104. [Crossref] [PubMed]