Identifying prognostic signatures in the microenvironment of prostate cancer
Introduction
Prostate cancer (PCa) is the most common non-cutaneous malignancy among men and the second leading cause of cancer-related death worldwide (1,2). The prognosis and treatment decisions of PCa are based on the malignant grade using the Gleason score, the clinical stage of the tumor-node-metastasis system (TNM), and serum PSA level (3,4). Despite these well-established clinical predictive and prognostic factors, drastically variable outcomes are observed among PCa patients with similar stages and malignant grades (5). Therefore, these observations have highlighted the importance of identifying novel biomarkers for the prognostic assessment of PCa.
The tumor microenvironment (TME) is the cellular environment that surrounds cancer cells. The TME is composed primarily of immune cells, mesenchymal cells, endothelial cells, inflammatory mediators, and extracellular matrix (ECM) molecules (6). Immune and stromal cells are the two major types of non-tumor cellular components in the TME (7). Previous studies reported that the proinflammation immune cells in the TME can promote tumor growth and progression including prostate cancer (8,9). Histological studies have found that 80–90% of prostate cancer specimens have signs of immune infiltration. The composition of immune infiltrates varies greatly depending on the stage of the disease and the age of the patient, but cells of the innate and adaptive immune systems have been found in the prostate stroma. Most immunoinfiltrating cells consist of CD3+ T cells, CD20+ B cells, and macrophages (10), but many other immune cell types have also been found. The immune response in the tumor immune microenvironment composed of these immune cells, including secretion and cytokines, can greatly affect the balance between tumor progression, tumor clearance and treatment response (11). In addition, increasing research has suggested that cells in the TME have clinicopathological significance in predicting patient prognosis and the therapeutic efficacy of drugs (4,5). In various malignancies, including gastric cancer and glioma, gene signatures from the TME were significantly associated with the outcomes of patients (6,12). Although the landscape of TME has been widely investigated across a range of tumor types, little evidence was revealed in PCa. Researchers found the TME in PCa is complex and various (8) and it plays a significant role in the initiation and progression of PCa (13,14). Importantly, a series of distinct cell changes in in the TME were involved in the initiating events of PCa. Previous studies uncovered a link between cells in the TME and malignant tumor cells, demonstrating that early changes in the normal tissue microenvironment can promote tumorigenesis and that tumor cells, in turn, promote further pro-tumor changes in the microenvironment (2). The abundance of immune cells and other cells in the TME can be estimated using computational methods (15,16). Several studies have explored the clinical utility of TME infiltrates using these methodologies (12,17), and several mechanisms underlying the role of TME in immunotherapy response and resistance have been experimentally identified for certain tumor types.
However, a comprehensive profile of the TME infiltrating cells and TME characterization in PCa has not yet been elucidated. In the present study, we developed a method to quantify the TME infiltration patterns (TMEscore) in PCa based on cluster signature genes from 477 patients with PCa. The correlation of TMEscore-related phenotypes with genomic and clinical features of PCa was systematically analyzed. As a result, we identified a set of TME-related signatures from the high and low TMEscore subtypes that can predict survival outcomes in PCa. Our study demonstrates that the TMEscore may be a reliable prognostic biomarker and predictive factor for developing new diagnosis strategies in PCa. We present the following article in accordance with the REMARK reporting checklist (available at https://dx.doi.org/10.21037/tau-21-819).
Methods
PCa data collection
The transcriptional sequencing data of PCa patients (TCGA-PRAD), as well as the clinical information including gender, age, and survival time were separately downloaded from TCGA database (https://xenabrowser.net/datapages) and the Gene Expression Omnibus (GEO) database (https://www.ncbi.nlm.nih.gov/geo/). The study was conducted in accordance with the Declaration of Helsinki (as revised in 2013). After removing the PCa samples without survival data or clinical data, 477 qualified transcriptome samples from TCGA were selected as test sets and 111 samples from GEO were used as the validation cohort (Table 1). The single nucleotide polymorphism (SNP) data of the 477 samples were obtained from TCGA. Among the 477 samples, 471 samples had SNP6 copy number segments available from https://firebrowse.org/.
Table 1
Series accession numbers | Platform | No. of patients | AJCC_Stage | Age, mean [min, max] | Region | Survival outcome |
---|---|---|---|---|---|---|
TCGA-PRAD | Illumina RNAseq | 477 | NA | 61.6 [42, 79] | NA | DFS |
GSE70770 | Illumina HumanHT-12 V4.0 expression beadchip | 111 | NA | 60.5 [41, 73] | UK | RFS |
PCa, prostate cancer; DFS, disease-free survival; RFS, relapse-free survival; NA, not available.
TME analysis
The proportion of immune cells in the TME was calculated using the CIBERSORT algorithm with the leukocyte gene signature matrix (LM22) as the reference and with 1,000 permutations (15). Based on the proportion analysis of immune cells, TME clusters were further determined using the ConsensusClusterPlus R package (18), and the optimum number of clusters K was estimated by algorithms elbow and gap statistics. The limma R package was used to identify the differentially expressed genes (DEGs) between the TME clusters, with thresholds of P<0.05 and |logFC|>0.58. Cluster signature genes identified by random forest method were further analyzed using ClusterProfiler R package for functional enrichment and classified into two categories according to a positive or negative coefficient of the Cox regression model (19). The TMEscore was calculated as follows:
where X represents the expression value of cluster signature genes with a positive Cox coefficient, while Y represents the expression value of cluster signature genes with a negative Cox coefficient.
The maxstat R package was used to determine the optimal cut-off TMEscore for categorizing the 477 PCa samples into low and high TMEscore groups. This TMEscore model was further validated in the GSE70770 cohort from GEO.
Mutation spectrum analysis
We analyzed the mutational spectrum and signatures of 477 samples from TCGA-PRAD by maftools and SomaticSignatures R packages. Copy number variable regions (CNVRs), including chromosomal CNVRs and minimal common regions (MCRs), were detected by GISTIC. Based on the results of CNVs, tumor purity and ploidy were estimated by ABSOLUTE R package.
Survival analysis and correlation analysis
Survival curves were plotted using surimer R software package. Limma R package was used to detect miRNAs and mRNA differentially expressed between high and low TMEscore subtypes. miRNAs and mRNA were enriched and analyzed by miRPathDB and clusterProfiler R package, respectively. The correlation between TMEscore subtype and clinical outcome was analyzed using the survival R package. Kaplan-Meier method was used to evaluate the relationship between the survival of PCa patients and genetic signatures, such as miRNA and mRNA expression, as well as methylation sites. We also explored the correlation between TMEscore and tumor response to immune checkpoint inhibitors (ICIs) in PCa. The tumor response to ICIs was evaluated by the Tumor Immune Dysfunction and Exclusion (TIDE) scoring system, with a higher TIDE score indicating a poorer response to ICIs and a worse prognosis.
Statistical analysis
R (https://www.r-project.org/) or SPSS software (version 25.0) was used for all statistical analyses. Statistical significance was defined as two-tailed P value <0.05.
Results
TME cell infiltrating patterns and DEGs associated with the TME in PCa
We calculated the proportion of immune cells in the TME of 477 PCa samples, based on previous findings that immune cells, especially tumor infiltrating lymphocytes, are more related to prognosis and immunotherapy efficacy compared with stromal cells (6,20). We obtained a comprehensive profile of the immune cell interactions, lineages and their effects on the overall survival (OS) of PCa patients and identified four distinct patterns of TME cell infiltration (Figure 1A). Cell cluster A was characterized by the infiltration of M0 macrophages, naïve B cells, resting mast cells and other cells. Cell cluster B was characterized by the infiltration of activated mast cells, neutrophils, activated NK cells and other cells. Cell clusters C and cluster D were characterized by the infiltration of resting memory CD4+ T cells and M2 macrophages, respectively. Based on the proportion of immune cells, the PCa samples can be divided into three TME clusters through consensus clustering analysis (Figure S1A-S1C). Interestingly, in mapping the TME clusters to the proportion of immune cells, we found significant differences in the distribution of immune cells between different TMEcluster samples, with TMEcluster 1 and 2 showing marked differences from TMEcluster 3 (Figure 1B). However, survival analysis showed that the OS was not significantly different among the three TME clusters (Figure 1C), which is likely because of redundant information caused by the LM22 gene. To eliminate the interference of redundant information, an unsupervised clustering method was used to re-cluster the 477 PCa samples based on the expression profile of TME-infiltrating phenotype related differential genes, yielding a total of 3,637 DEGs. After dimensionality reduction by random forest algorithm, the most relevant signature genes (n=104) were used to classify the 477 samples into two clusters (Figure 1D). These genes are mainly enriched in the pathways of immune activation, such as neutrophil chemotaxis, alpha-beta T cell activation, alpha-beta T cell differentiation, neutrophil migration and other pathways (Figure 1E).
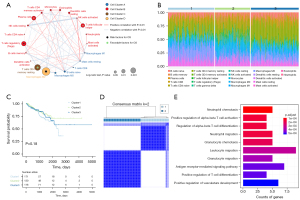
Construction of the TMEscore model for PCa samples
Using the re-clustering model, we evaluated the correlation between DEGs and prognosis. The Cox regression model was used to evaluate the relationship between the DEGs and OS in PCa samples. According to the coefficient value of genes, the 477 samples were classified into two groups: the high (n=308) and low (n=169) TMEscore phenotypes (x=−0.31).
As shown in Figure 2A, the prognostic performance in the high TMEscore group was significantly better than in the low TMEscore group. A comprehensive comparison of different TME clusters (Figure 2B) indicated that cluster samples based on immune cell components and proportions were significantly correlated with DEGs and TMEscore. Consistent with these findings, the TMEscore of the 111 PCa samples from GEO also reflected the prognosis of samples (Figure 2C,2D).
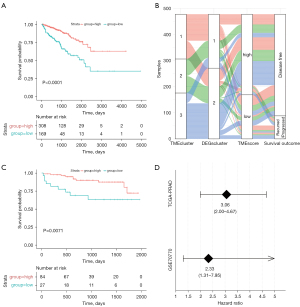
Correlation of the mutational signatures with the TMEscore phenotypes in PCa
The common genomic mutations, such as SNPs, have been reported as predictors for aggressive PCa. Exploration of unique SNPs would enable more accurate risk stratification for individualized management of PCa patients (21). Therefore, we explored the relationship between SNPs and the TMEscore. The results showed that the most common mutation in PCa is the missense mutation, mainly caused by SNPs, with C > T as the most common type (Figure S2). The frequently mutated genes in the low and high TMEscore groups are presented in Figure 3A,3B, respectively, and the variant allele fractions (VAFs) were different between the two groups (Figure 3C). The contribution of 96 base substitution types is presented in Figure S3A,S3B. Mutational signature analysis showed that the high TMEscore group was mainly related to signature1, signature3 and signature5 (Figure 3D), and the TMEscore low group was primarily related to signature1, signature5 and signature6 (Figure 3E), with significant differences between the two groups.
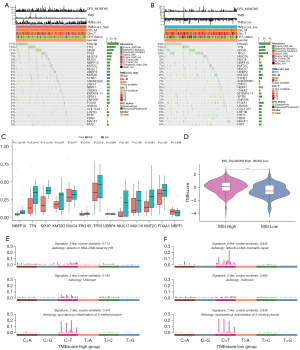
Microsatellite instability (MSI) is an emerging biomarker used to predict the outcome of cancer treatment, and patients with MSI-High usually have better prognosis than those with MSI-Low and microsatellite stable (MSS). Correlation analysis between TMEscore and MSI showed that the high TMEscore was significantly associated with MSI-H (Figure 3F).
Correlation of CNV with the TMEscore phenotypes in PCa
As shown in Figure 4, our analysis showed that there were significant differences in chromosome copy number amplifications or deletions between the two TMEscore-related phenotypes. CNV analysis by GISTIC showed that amplifications of chromosomal arms 8p, 8q, 7p and 7q as well as deletions of chromosomal arms 8p, 18p, 18q and 16q frequently occurred in the high TMEscore subtype (Figure 4A,4C), whereas amplifications of chromosomal arms 8p, 8q, 7p and 7q as well as deletions of chromosomal arms 8p and 18q were common in the low TMEscore subtype (Figure 4B,4D). There were also significant differences in major chromosomal rearrangements (MCRs) between the high TMEscore group and low TMEscore group. In the high TMEscore group, 14 amplifications and 30 deletions were detected, and the 3q26.2 amplification and 8p21.3 deletion were mostly representative (Figure 4E). In the low TMEscore group, 22 amplifications and 24 deletions were detected, with the 8q24.21 and 8q21.13 amplifications and 6q14.3 and 13q14.13 deletions being the most common (Figure 4F). However, we found no significant correlations between tumor purity and tumor ploidy with the TMEscore-related phenotypes (Figure S3C,S3D).
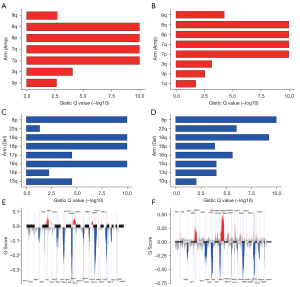
Comprehensive analysis of genomic signatures associated with the TMEscore in PCa
Based on the TMEscore phenotypes, we conducted a comprehensive analysis of the genomic signatures associated with the prognosis of PCa. DEG analysis identified 5 miRNAs and 127 mRNAs that were differentially expressed between the high and low TMEscore subtypes, with a threshold of P<0.05 and |logFC|>1 for miRNA and |logFC|>1 and P<0.05 for mRNA. Among the DEGs, has-mir-133b (Figure 5A) and FMOD (Figure 5B) were most significantly correlated with PCa survival.
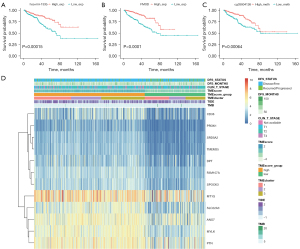
In addition, as DNA methylation status is closely related to tumor progression and prognosis, we performed differential methylation analysis between the high and low TMEscore groups and identified 38 significant differential methylation sites. Among the identified sites, cg03804126 was most significantly correlated with OS (Figure 5C).
A comprehensive genomic landscape of PCa is presented in Figure 5D, with 12 identified survival-related genes, including CD38, PROK1, SRD5A2, TMEM35, DPT, FAM107A, SPOCK3, MT1G, SLC22A3, ANO7, MYLK and PTN.
Discussion
Prostate cancer is driven by a variety of genomic changes, with different patterns and clinical significance. Alterations occurring early in the timeline of the disease define core subtypes of localized, treatment-naive PCa. Recent studies have explored the biological characteristics of these different gene expression characteristics, enabling them to express their unique functional pathways. This facilitates the development of specific treatment strategies for each subclass. For example, fusion of ETS family genes with androgen-regulated prostate-specific genes is the most common recurrent rearrangement of PCa and is considered to be a unique “ETS positive” subtype that is itself a marker of poor prognosis (22,23). The most common recurrent point mutations in PCa involve mutations in SPOP (Speckle-Type POZ Protein, SPOP mutant subclass), FOXA1 (forkhead box A1), etc. Specifically, FOXA1 mutations are mostly mutually exclusive with ETS fusion positive and SPOP mutations. In addition, for metastatic PCa, loss of function of PTEN and TP53 is very common, and most of the molecular alterations of PTEN belong to the ETS-fusion-positive subclass (24,25). All subtypes of localized diseases initially respond to androgen deprivation therapy (ADT), but as castration-resistant PCa (CRPC) progresses, the anti-ADT mechanism has changed the molecular landscape. A broad spectrum of potentially actionable molecular alterations contribute to tumor heterogeneity, such as metastatic prostate cancer, which is a lethal, highly heterogeneous malignancy. And somatic changes increase gradually over time and with treatment exposure.
In recent years, gene signatures representing TME status have been identified and evaluated for their potential clinical relevance in a variety of cancers (12,21,26). In the present study, we focused on the TME signatures that contribute to survival of PCa samples from TCGA and GEO databases. Based on the TME infiltration pattern analysis of 477 PCa samples, our results suggested that infiltrating immune cells such as M2 macrophages, resting memory CD4+ T cells, regulatory T cells, plasma cells and follicular T cells were closely related to the survival outcome of PCa patients. Notably, our bioinformatic findings were consistent with several previous experimental studies. For example, most (but not all) pathological studies in PCa found that a higher number of tumor-associated macrophages was associated with worse prognosis (27,28). Additionally, M2 macrophages were reported to be associated with more advanced stage and higher Gleason scores of tumors (29). Researchers also found that regulatory T cells were elevated in the circulation of patients with PCa, and the increased number was positively associated with poor outcome (30). The immune response in the tumor immune microenvironment composed of these immune cells, involving secretion and cytokines, can greatly influence the balance between tumor progression, tumor clearance, and therapeutic response (31). Interference between cells, soluble and insoluble factors with cancer cells leads to changes in theTME, which modulates and contributes to more aggressive phenotypes, such as the emergence of androgen-independent states and tumors leading to metastatic castration to resist prostate cancer. Patients who progress to this advanced disease have a poor survival prognosis. They are highly resistant to treatment, leading to treatment failure, tumor recurrence and patient death (32). Therefore, understanding the functional relationship that coordinates the relevant immune signals in the TME may help to better predict the progression of PCa and the response of advanced disease treatment strategies, so as to provide personalized medicines for reducing the mortality of PCa.
In this study, we developed the TMEscore model to predict the prognosis of PCa. Consistent with previous studies in other types of tumors (6,12), PCa patients with a high TMEscore high phenotype showed a better prognosis than those with a low TMEscore. We also evaluated the efficacy of the TMEscore on prognosis compared with other common prognostic factors. A previous report showed that SNP may act as a predictor of aggressive PCa and would enable more accurate risk stratification in PCa (33). Another study reported that MSI did not correlate with clinical stage, but might play a role in the development of a subset of PCa. The MSI-H/dMMR molecular phenotype is uncommon in prostate cancer, but it has therapeutic significance (34). Here, we found a strong positive correlation between TMEscore and SNP or MSI-H in PCa, suggesting that the TMEscore may be useful in developing new diagnostic strategies for PCa.
To explore other potential prognostic signatures, we integrated the genomic, clinical and pathological features of PCa data. We identified a list of prognostic signatures, including miRNAs, mRNA and methylation sites. miRNAs are short, endogenous cellular RNAs that function in translational repression and/or destabilizing target mRNAs to regulate various biological processes (35). miRNAs can act as oncogenic miRNAs or tumor suppressors in a multitude of cancers (36). Numerous studies showed that the miRNAs profile is associated with the initiation and progression of PCa (37). Moreover, several miRNAs, such as miR-133b and miR-1, have been identified as novel biomarkers with prognostic and diagnostic value for PCa recurrence (38). Consistent with these findings, we discovered that miR-133b showed the most significant prognostic value in the TMEscore model of PCa. Another study reported FMOD mRNA as a potential biomarker for PCa (39) and we also identified FMOD as showing the most significant prognostic value from the mRNA profiles between high and low TMEscore groups. These results highlight the significance of the TMEscore in recognizing prognostic signatures. Although the TMEscore phenotype of PCa can provide an alternative set of biomarkers with good prognostic ability, our results alone are insufficient for clinical application. Hence, further validation and functional research, especially genetic and experimental studies involving more clinical samples, should be conducted in the future.
Conclusions
Our study provides a comprehensive assessment of the cellular, molecular and genetic factors associated with TMEscore in PCa patients, providing several critical insights into how PCa respond to survival outcomes and may help guide the development of new diagnostic strategies.
Acknowledgments
We thank Shanghai Tongshu Biotechnology Co., Ltd. for support of bioinformatics technology.
Funding: None.
Footnote
Reporting Checklist: The authors have completed the REMARK reporting checklist. Available at https://dx.doi.org/10.21037/tau-21-819
Conflicts of Interest: All authors have completed the ICMJE uniform disclosure form (available at https://dx.doi.org/10.21037/tau-21-819). WC reports that she is from Shanghai Tongshu Biotechnology Co., Ltd. The other authors have no conflicts of interest to declare.
Ethical Statement: The authors are accountable for all aspects of the work in ensuring that questions related to the accuracy or integrity of any part of the work are appropriately investigated and resolved. The study was conducted in accordance with the Declaration of Helsinki (as revised in 2013).
Open Access Statement: This is an Open Access article distributed in accordance with the Creative Commons Attribution-NonCommercial-NoDerivs 4.0 International License (CC BY-NC-ND 4.0), which permits the non-commercial replication and distribution of the article with the strict proviso that no changes or edits are made and the original work is properly cited (including links to both the formal publication through the relevant DOI and the license). See: https://creativecommons.org/licenses/by-nc-nd/4.0/.
References
- Shukla ME, Yu C, Reddy CA, et al. Evaluation of the current prostate cancer staging system based on cancer-specific mortality in the surveillance, epidemiology, and end results database. Clin Genitourin Cancer 2015;13:17-21. [Crossref] [PubMed]
- Shiao SL, Chu GC, Chung LW. Regulation of prostate cancer progression by the tumor microenvironment. Cancer Lett 2016;380:340-8. [Crossref] [PubMed]
- Crawford ED, Ventii K, Shore ND. New biomarkers in prostate cancer. Oncology (Williston Park) 2014;28:135-42. [PubMed]
- Wu X, Lv D, Eftekhar M, et al. A new risk stratification system of prostate cancer to identify high-risk biochemical recurrence patients. Transl Androl Urol 2020;9:2572-86. [Crossref] [PubMed]
- McAllister MJ, Underwood MA, Leung HY, et al. A review on the interactions between the tumor microenvironment and androgen receptor signaling in prostate cancer. Transl Res 2019;206:91-106. [Crossref] [PubMed]
- Boussadia Z, Zanetti C, Parolini I. Role of microenvironmental acidity and tumor exosomes in cancer immunomodulation. Transl Cancer Res 2020;9:5775-86. [Crossref]
- Sun DY, Wu JQ, He ZH, et al. Cancer-associated fibroblast regulate proliferation and migration of prostate cancer cells through TGF-beta signaling pathway. Life Sci 2019;235:116791 [Crossref] [PubMed]
- Kim J, Bae JS. Tumor-Associated Macrophages and Neutrophils in Tumor Microenvironment. Mediators Inflamm 2016;2016:6058147 [Crossref] [PubMed]
- Crusz SM, Balkwill FR. Inflammation and cancer: advances and new agents. Nat Rev Clin Oncol 2015;12:584-96. [Crossref] [PubMed]
- De Nunzio C, Kramer G, Marberger M, et al. The controversial relationship between benign prostatic hyperplasia and prostate cancer: the role of inflammation. Eur Urol 2011;60:106-17. [Crossref] [PubMed]
- Vitkin N, Nersesian S, Siemens DR, et al. The Tumor Immune Contexture of Prostate Cancer. Front Immunol 2019;10:603. [Crossref] [PubMed]
- Zeng D, Li M, Zhou R, et al. Tumor Microenvironment Characterization in Gastric Cancer Identifies Prognostic and Immunotherapeutically Relevant Gene Signatures. Cancer Immunol Res 2019;7:737-50. [Crossref] [PubMed]
- Taverna G, Pedretti E, Di Caro G, et al. Inflammation and prostate cancer: friends or foe? Inflamm Res 2015;64:275-86. [Crossref] [PubMed]
- De Marzo AM, Platz EA, Sutcliffe S, et al. Inflammation in prostate carcinogenesis. Nat Rev Cancer 2007;7:256-69. [Crossref] [PubMed]
- Newman AM, Liu CL, Green MR, et al. Robust enumeration of cell subsets from tissue expression profiles. Nat Methods 2015;12:453-7. [Crossref] [PubMed]
- Becht E, Giraldo NA, Lacroix L, et al. Estimating the population abundance of tissue-infiltrating immune and stromal cell populations using gene expression. Genome Biol 2016;17:218. [Crossref] [PubMed]
- Fu H, Zhu Y, Wang Y, et al. Identification and Validation of Stromal Immunotype Predict Survival and Benefit from Adjuvant Chemotherapy in Patients with Muscle-Invasive Bladder Cancer. Clin Cancer Res 2018;24:3069-78. [Crossref] [PubMed]
- Sorlie T, Perou CM, Tibshirani R, et al. Gene expression patterns of breast carcinomas distinguish tumor subclasses with clinical implications. Proc Natl Acad Sci U S A 2001;98:10869-74. [Crossref] [PubMed]
- Ritchie ME, Phipson B, Wu D, et al. limma powers differential expression analyses for RNA-sequencing and microarray studies. Nucleic Acids Res 2015;43:e47 [Crossref] [PubMed]
- Lang JM. Understanding dynamic interactions in the prostate tumor microenvironment. Urol Oncol 2019;37:532-4. [Crossref] [PubMed]
- Wolff C, Zoschke C, Kalangi SK, et al. Tumor microenvironment determines drug efficacy in vitro - apoptotic and anti-inflammatory effects of 15-lipoxygenase metabolite, 13-HpOTrE. Eur J Pharm Biopharm 2019;142:1-7. [Crossref] [PubMed]
- Nam RK, Sugar L, Yang W, et al. Expression of the TMPRSS2:ERG fusion gene predicts cancer recurrence after surgery for localised prostate cancer. Br J Cancer 2007;97:1690-5. [Crossref] [PubMed]
- Arora K, Barbieri CE. Molecular Subtypes of Prostate Cancer. Curr Oncol Rep 2018;20:58. [Crossref] [PubMed]
- Cancer Genome Atlas Research N. The Molecular Taxonomy of Primary Prostate Cancer. Cell 2015;163:1011-25. [Crossref] [PubMed]
- Robinson D, Van Allen EM, Wu YM, et al. Integrative clinical genomics of advanced prostate cancer. Cell 2015;161:1215-28. [Crossref] [PubMed]
- Pfannstiel C, Strissel PL, Chiappinelli KB, et al. The Tumor Immune Microenvironment Drives a Prognostic Relevance That Correlates with Bladder Cancer Subtypes. Cancer Immunol Res 2019;7:923-38. [Crossref] [PubMed]
- Shimura S, Yang G, Ebara S, et al. Reduced infiltration of tumor-associated macrophages in human prostate cancer: association with cancer progression. Cancer Res 2000;60:5857-61. [PubMed]
- Nonomura N, Takayama H, Nakayama M, et al. Infiltration of tumour-associated macrophages in prostate biopsy specimens is predictive of disease progression after hormonal therapy for prostate cancer. BJU Int 2011;107:1918-22. [Crossref] [PubMed]
- Lanciotti M, Masieri L, Raspollini MR, et al. The role of M1 and M2 macrophages in prostate cancer in relation to extracapsular tumor extension and biochemical recurrence after radical prostatectomy. Biomed Res Int 2014;2014:486798 [Crossref] [PubMed]
- Ebelt K, Babaryka G, Frankenberger B, et al. Prostate cancer lesions are surrounded by FOXP3+, PD-1+ and B7-H1+ lymphocyte clusters. Eur J Cancer 2009;45:1664-72. [Crossref] [PubMed]
- Archer M, Dogra N, Kyprianou N. Inflammation as a Driver of Prostate Cancer Metastasis and Therapeutic Resistance. Cancers (Basel) 2020;12:2984. [Crossref] [PubMed]
- Crawford ED, Petrylak D, Sartor O. Navigating the evolving therapeutic landscape in advanced prostate cancer. Urol Oncol 2017;35S:S1-S13. [Crossref] [PubMed]
- Benafif S, Kote-Jarai Z, Eeles RA, et al. A Review of Prostate Cancer Genome-Wide Association Studies (GWAS). Cancer Epidemiol Biomarkers Prev 2018;27:845-57. [Crossref] [PubMed]
- Antonarakis ES, Shaukat F, Isaacsson Velho P, et al. Clinical Features and Therapeutic Outcomes in Men with Advanced Prostate Cancer and DNA Mismatch Repair Gene Mutations. Eur Urol 2019;75:378-82. [Crossref] [PubMed]
- Valencia-Sanchez MA, Liu J, Hannon GJ, et al. Control of translation and mRNA degradation by miRNAs and siRNAs. Genes Dev 2006;20:515-24. [Crossref] [PubMed]
- Shenouda SK, Alahari SK. MicroRNA function in cancer: oncogene or a tumor suppressor? Cancer Metastasis Rev 2009;28:369-78. [Crossref] [PubMed]
- Pashaei E, Pashaei E, Ahmady M, et al. Meta-analysis of miRNA expression profiles for prostate cancer recurrence following radical prostatectomy. PLoS One 2017;12:e0179543 [Crossref] [PubMed]
- Karatas OF, Guzel E, Suer I, et al. miR-1 and miR-133b are differentially expressed in patients with recurrent prostate cancer. PLoS One 2014;9:e98675 [Crossref] [PubMed]
- Bettin A, Reyes I, Reyes N. Gene expression profiling of prostate cancer-associated genes identifies fibromodulin as potential novel biomarker for prostate cancer. Int J Biol Markers 2016;31:e153-62. [Crossref] [PubMed]
(English Language Editor: L. Huleatt)